An open-source EEGLAB plugin for computing entropy-based measures on MEEG signals
crossref(2022)
摘要
Machine learning (ML) is revolutionizing the field of biosignals processing and classification. Feature-based and feature-free (deep learning) ML perform equally well on MEEG (electroencephalography and magnetoencephalography) data classification problems. Yet, feature-based ML approaches dominantly rely on traditional, linear MEEG measures such as power spectral density, potentially missing useful information about the neural time series. There is a need for more advanced features that can capture the nonlinearity, complexity, and irregularities of such signals. Entropy-based measures are promising techniques to fill this gap but are difficult to apply to MEEG signals because of the variety of algorithms and parameters available, while user-friendly tools and methodological guidelines are lacking. Hence, we have developed a set of MEEG tools to fill that gap, where we gathered multiple entropy measures, tailored them for MEEG signal, and packaged them in an open-source EEGLAB plugin with a graphical user interface (GUI). We hope this newly accessible framework will help popularize the use of entropy measures with MEEG data, their cross-comparison, and their use as a feature for ML models.
更多查看译文
AI 理解论文
溯源树
样例
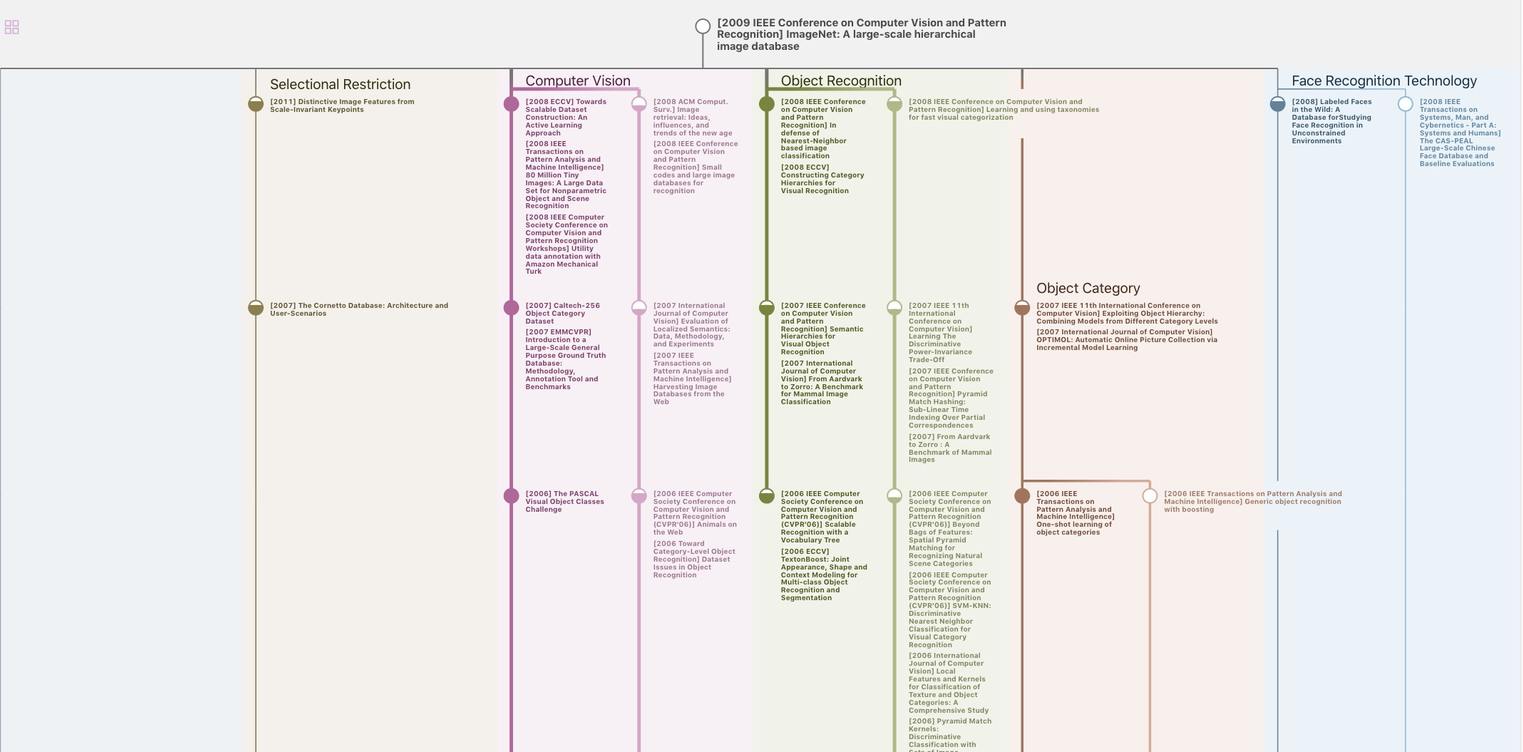
生成溯源树,研究论文发展脉络
Chat Paper
正在生成论文摘要