Multi-scale cross-fusion for arbitrary scale image super resolution
Multimedia Tools and Applications(2024)
摘要
Deep convolutional neural networks (CNNs) have great improvements for single image super resolution (SISR). However, most of the existing SISR pre-training models can only reconstruct low-resolution (LR) images in a single image, and their upsamling factors cannot be non-integers, which limits their application in practical scenarios. In this letter, we propose a multi-scale cross-fusion network (MCNet) to accomplish the super-resolution task of images at arbitrary scale. On the one hand, the designed scale-wise module (SWM) combine the scale information and pixel features to fullly improve the representation ability of arbitrary-scale images. On the other hand, we construct a multi-scale cross-fusion module (MSCF) to enrich spatial information and remove redundant noise, which uses deep feature maps of different sizes for interactive learning. A large number of experiments on four benchmark datasets show that the proposed method can obtain better super-resolution results than existing arbitrary scale methods in both quantitative evaluation and visual comparison.
更多查看译文
关键词
Image super-resolution,Deep convolution neural networks,Multi-scale information
AI 理解论文
溯源树
样例
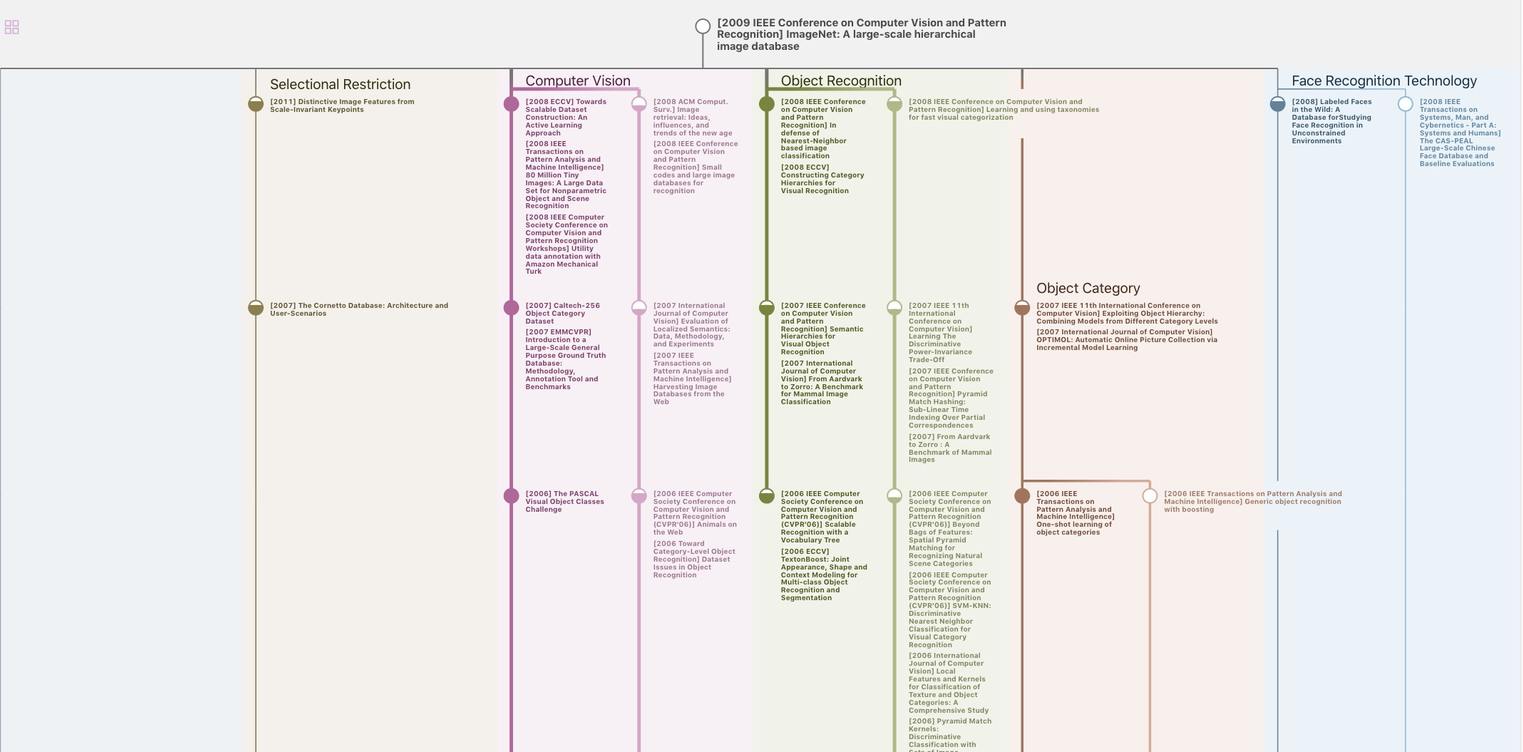
生成溯源树,研究论文发展脉络
Chat Paper
正在生成论文摘要