Neural Process for Health Prognostics With Uncertainty Estimations
IEEE Transactions on Industrial Informatics(2023)
摘要
Gaussian processes (GPs) and neural networks (NNs) are two kinds of function approximation models that are widely used for health prognostics. As an emerging meta-learning method, neural processes (NPs) combine the advantages of GPs and NNs. Similar to GPs, NPs can quickly adapt to new observations and can estimate the uncertainty in their prediction. Similar to NNs, NPs are scalable for complex functions and large datasets. However, NPs do not have the capability to handle the prognostics in the time series prevailing in health prognostics. Inspired by these, an NP-based health prognostic toward prognostic uncertainty is presented. The method is validated by datasets that are sourced from real industrial case. Meanwhile, the proposed method is compared with the state-of-the-art NN- and GP-based health prognostic methods, which are also developed for prognostics with uncertainty estimations. The results show that the proposed method performs the prognostics with superior accuracy and quality.
更多查看译文
关键词
Health prognostics,neural process (NP),remaining useful life (RUL),time series,uncertainty estimation
AI 理解论文
溯源树
样例
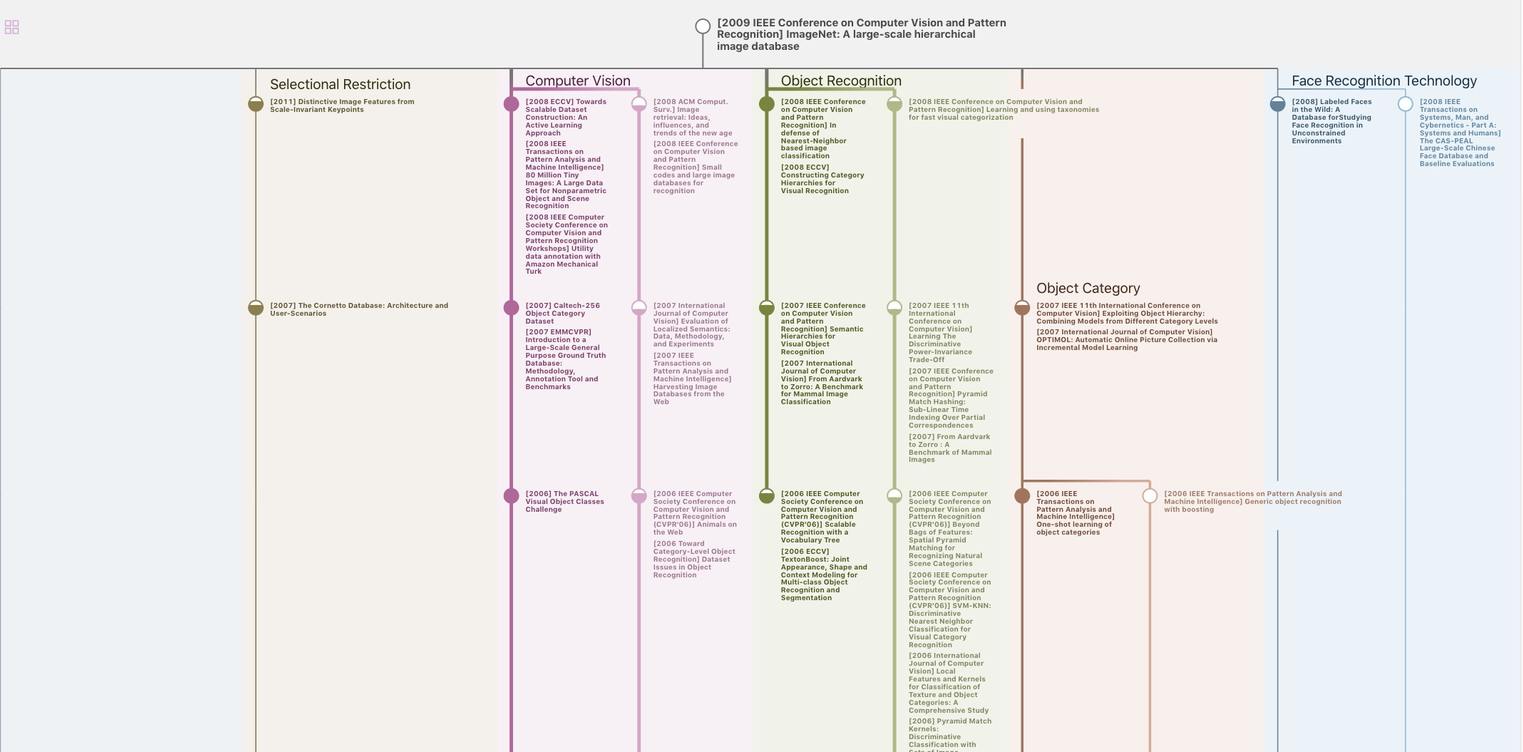
生成溯源树,研究论文发展脉络
Chat Paper
正在生成论文摘要