Joint Learning of Failure Mode Recognition and Prognostics for Degradation Processes.
IEEE transactions on automation science and engineering : a publication of the IEEE Robotics and Automation Society(2023)
摘要
To avoid unexpected failures of units in manufacturing systems, failure mode recognition and prognostics are critically important in prognostics health management (PHM). Most existing methods either ignored the effects of various failure modes on remaining useful lifetime (RUL) prediction or implemented failure mode recognition and RUL prediction as two independent tasks, which failed to exploit failure mode information to obtain accurate RUL prediction. In fact, RUL highly depends on failure modes because sensor signals under different failure modes usually present different degradation patterns. To address the issue, this paper proposes a joint learning model of failure mode recognition and RUL prediction for degradation processes based on multiple sensor signals. The proposed joint learning model first extracts features by considering the degradation mechanism to ensure good interpretability for degradation modeling, and then takes the extracted features as inputs to a deep neural network. By conducting failure mode recognition and RUL prediction as a collaborative task, the proposed model can fully characterize the complex relationship among the extracted features, RUL and failure modes, and outputs the recognized failure modes and the predicted RUL of units simultaneously. A case study on the degradation of aircraft gas turbine engines is presented to evaluate the proposed model performance.
Note to Practitioners—:The paper aims to develop a joint learning method for failure mode recognition and RUL prediction of operating units. Specifically, the developed method addresses a challenging issue in practice, i.e., how to effectively conduct failure mode recognition and RUL prediction as a joint task based on interpretable extracted degradation features from multiple sensor signals. To implement this method in practice, four steps are included as follows: First, collect multiple sensor signals, failure time, and failure modes of historical units. Second, construct the joint learning model based on features extracted from sensor signals by considering the degradation mechanism. Third, estimate model parameters using the data of historical units. Fourth, recognize the failure mode and predict the RUL of an in-service unit. Since the proposed method is a data-driven neural network with flexible model structure that considers complex data relationships, it is expected to be applicable to many practical situations and use cases, especially for manufacturing systems with complex structures and unknown failure thresholds.
更多查看译文
关键词
Joint learning,failure mode recognition,remaining useful lifetime prediction,feature extraction
AI 理解论文
溯源树
样例
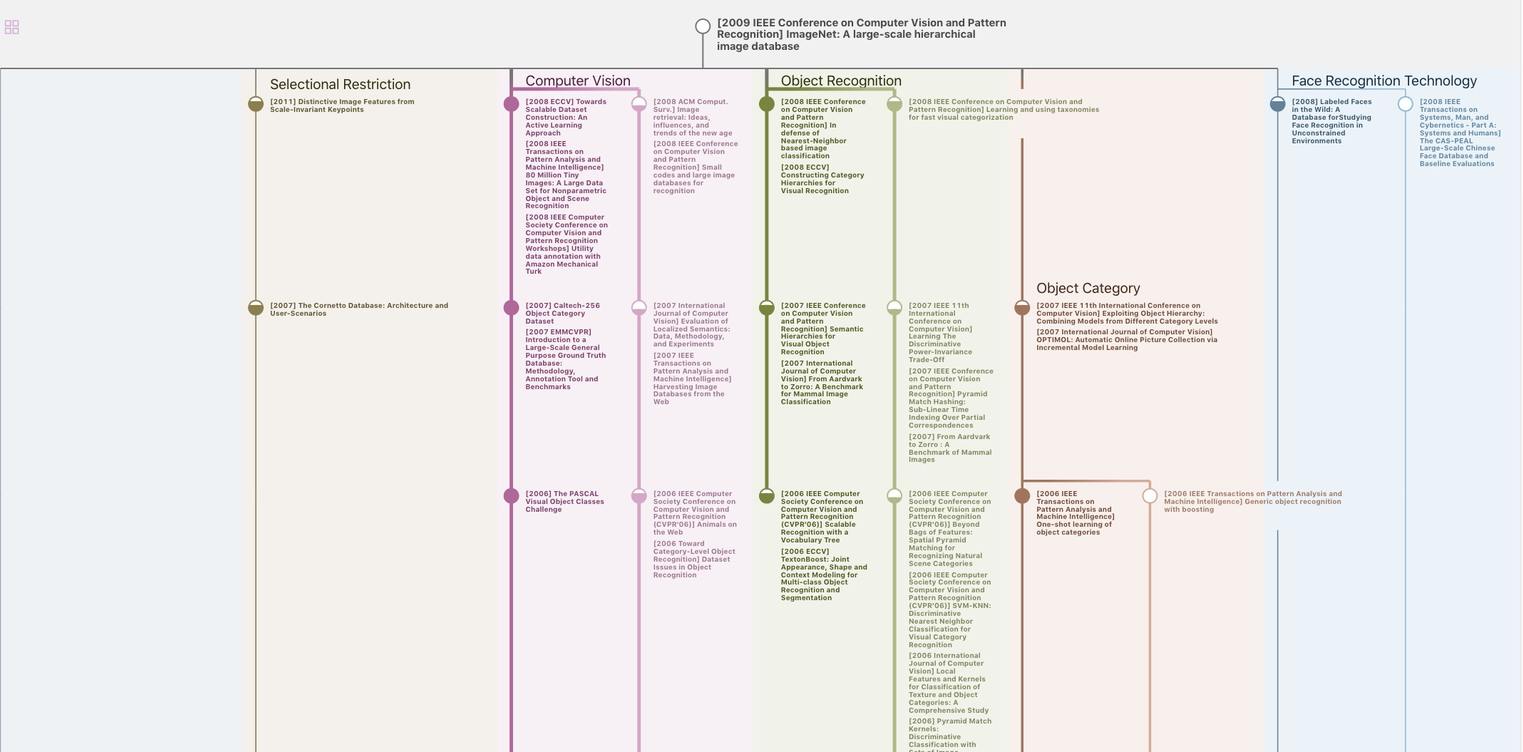
生成溯源树,研究论文发展脉络
Chat Paper
正在生成论文摘要