Data association for maneuvering targets through a combined siamese network and XGBoost model
Signal Processing(2023)
Abstract
Data association plays an important role in forming target tracks when false alarms exist. Its accuracy is key to reducing the computational burden of the combinatorial explosion problem inherent to target tracking in environments where false alarms are densely distributed. Traditional methods are usually de-veloped according to some assumed motion models and suffer performance degradation when there is mismatch between assumed models and the actual motion, which may often be the case for maneu-vering targets. To cope with this problem, we propose a data-driven method that learns the association criteria directly from data. By use of the domain knowledge and a convolutional Siamese network, fea-tures of different attributes present in the observations are extracted. Then the features are fused through the use of XGBoost. Simulation results show that the proposed method performs better than the tradi-tional model-based methods in correlating observations of maneuvering targets and avoiding correlations of false alarms. By visualizing the decision process of the feature fusion model, it is found that the pro-posed method learns from the data to make comprehensive use of multiple features and does not simply set hard thresholds for the features. The computational complexity is also analyzed both theoretically and experimentally.& COPY; 2023 Published by Elsevier B.V.
MoreTranslated text
Key words
Data association,Target tracking,Siamese neural network,XGBoost
AI Read Science
Must-Reading Tree
Example
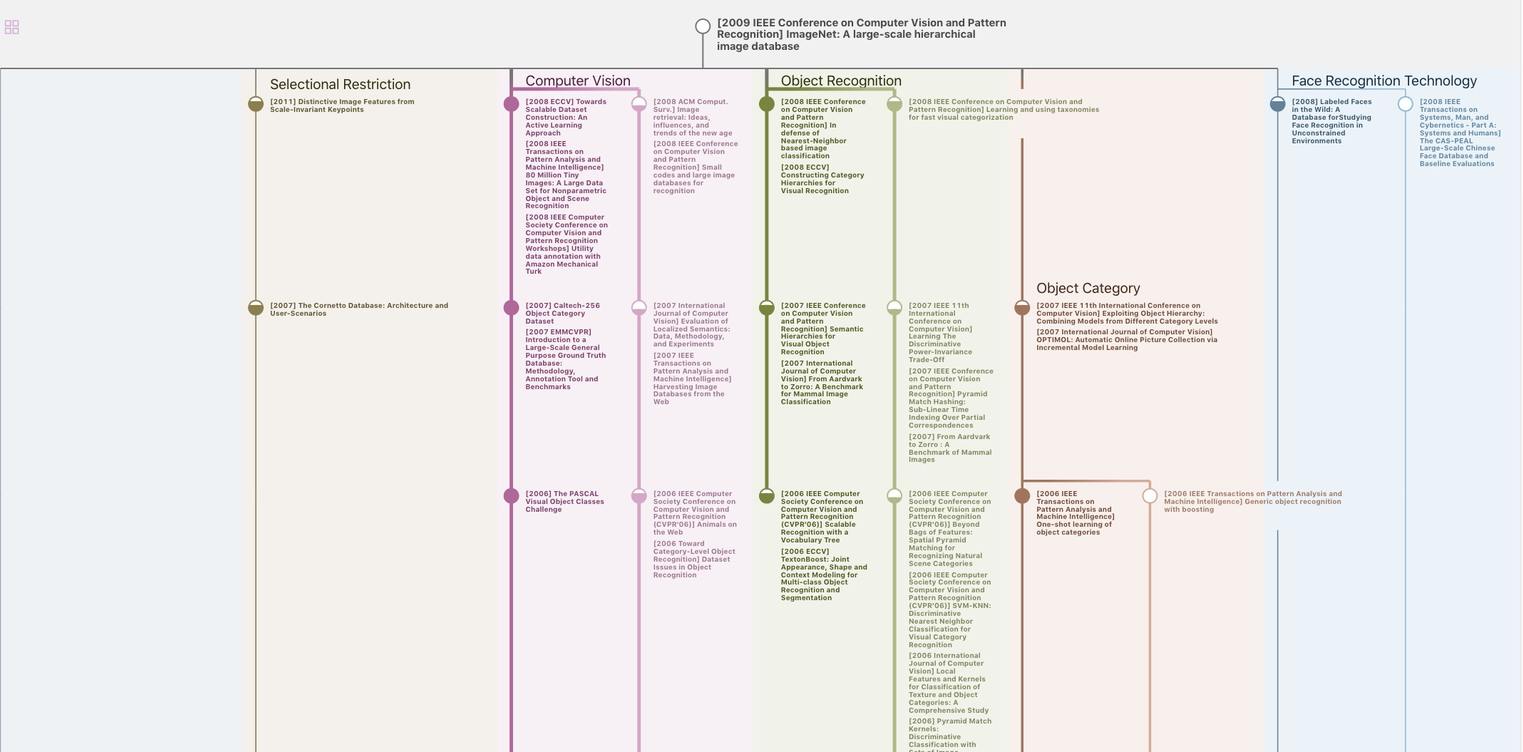
Generate MRT to find the research sequence of this paper
Chat Paper
Summary is being generated by the instructions you defined