Sequential architecture-agnostic black-box attack design and analysis
PATTERN RECOGNITION(2024)
摘要
Although adversarial machine learning attacks on image recognition models have been heavily investigated, the rising popularity of vision transformers revitalized the research on this topic. Due to the fundamental architectural differences between CNNs, which still dominate the image recognition applications, and trans-formers, the state-of-the-art attacks designed for CNNs are not effective against transformers, and vice versa. Such lack of transferability in attacks and the growing architectural heterogeneity in practice make the black -box attack design increasingly challenging. However, skillful attackers can handle the increasing uncertainty in target model architecture following two main approaches: designing transferable attacks that are robust to the architectural uncertainty in target model, and identifying the target architecture for attack selection. In this work, following the latter approach we propose a novel architecture-agnostic black-box attack design and analyze its performance. Experiments show that the proposed method, with a reasonable query overhead, outperforms the recent robust attack designs that follow the former approach. Different from the existing methods, the proposed method optimizes a trade-off between prior information about the target model and number of queries.
更多查看译文
关键词
Adversarial machine learning,Black-box attacks,Transferability of attacks,Vision transformers,Sequential hypothesis testing
AI 理解论文
溯源树
样例
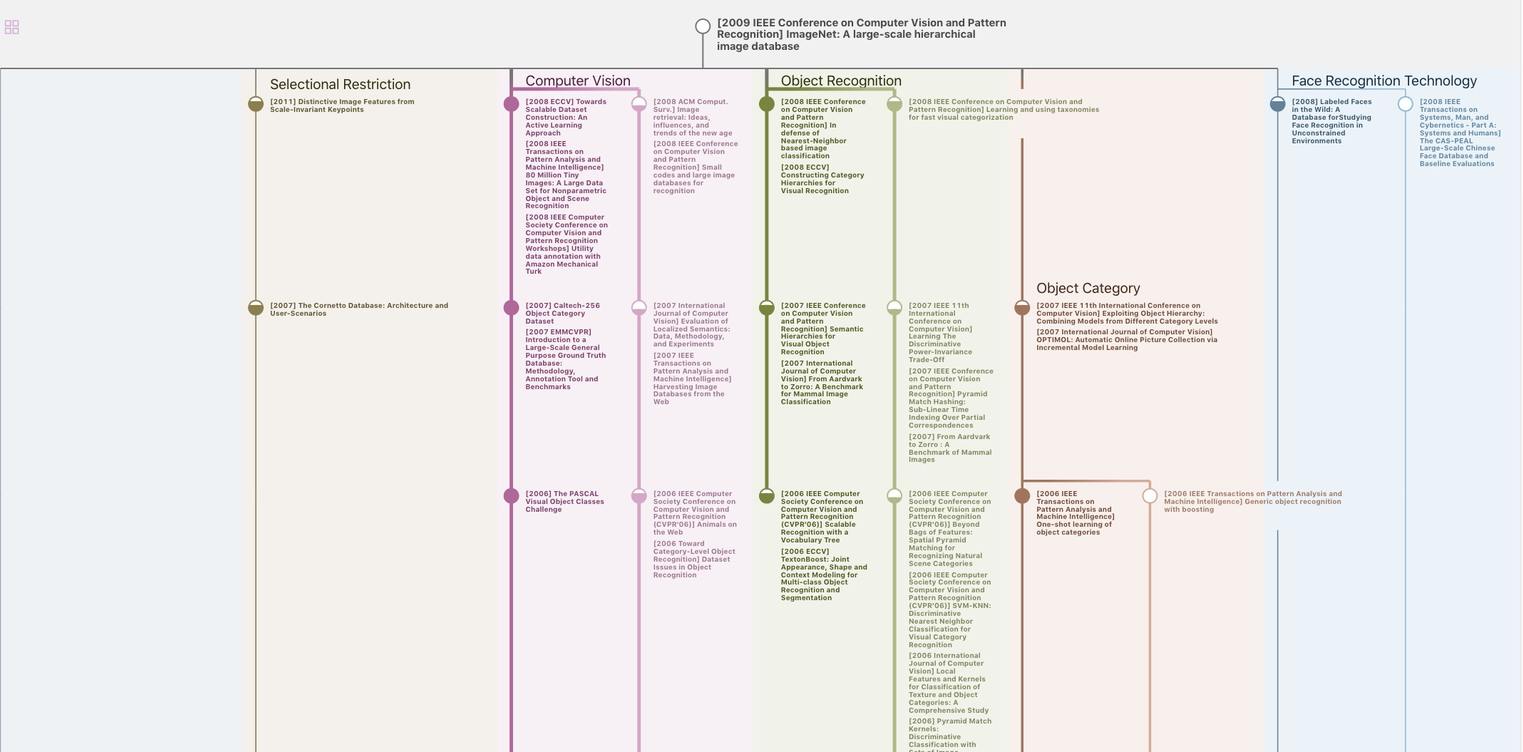
生成溯源树,研究论文发展脉络
Chat Paper
正在生成论文摘要