Training-Free Lane Tracking for 1/10th Scale Autonomous Vehicle Using Inverse Perspective Mapping and Probabilistic Hough Transforms
2022 IEEE Integrated STEM Education Conference (ISEC)(2022)
摘要
Autonomous vehicles (AVs) present a promising alternative to traditional driver-controlled vehicles with potential improvements in speed, energy, and safety domains. Pipelined approaches to AV development isolate individual facets of driving and produce autonomous systems focused on a specific task rather than developing an end-to-end framework for driving. This paper focuses on the design and development of lane tracking for a 1/10th scale autonomous vehicle. The developed lane tracking system implements inverse perspective mapping to autonomously adjust the perception of the vehicle’s camera feed and then applies probabilistic Hough transforms to identify the edge lines of a scaled-down lane. The computed Hough lines are utilized to evaluate the necessary steering adjustment angle in real time for the vehicle. The results of our steering adjustment stability experiments demonstrate the effective performance of the proposed lane tracking system and algorithms with low computational complexity. This work provides both a successful approach to lane tracking that does not require computing intensive model training on potentially location biased annotated data and an approach for vehicle vision feed annotation.
更多查看译文
关键词
inverse perspective mapping,scale autonomous vehicle,training-free
AI 理解论文
溯源树
样例
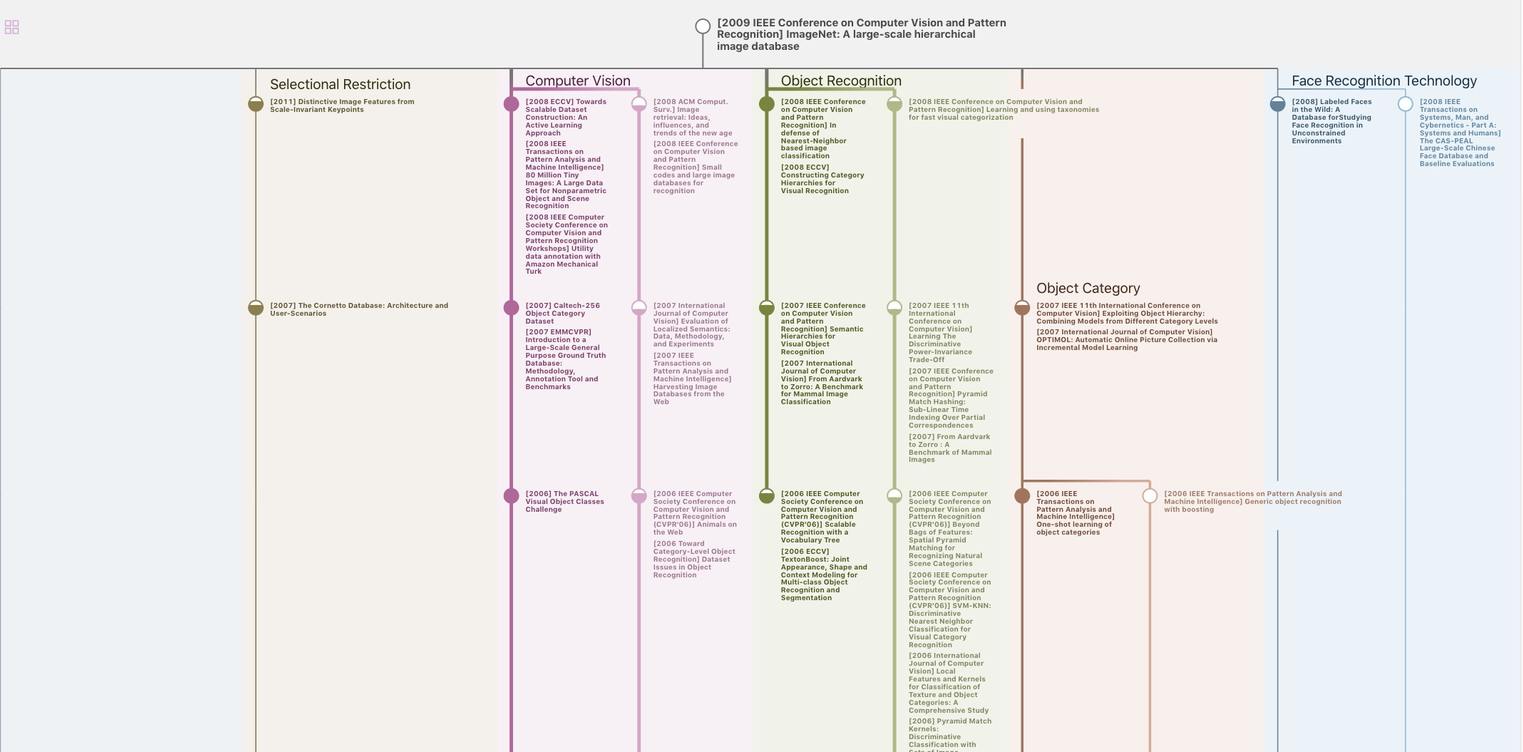
生成溯源树,研究论文发展脉络
Chat Paper
正在生成论文摘要