HB-YOLOv5: Improved YOLOv5 Based on Hybrid Backbone for Infrared Small Target Detection on Complex Backgrounds
Earth and Space: From Infrared to Terahertz (ESIT 2022)(2023)
Abstract
Infrared detecting and tracking system plays an important role in national security. In order to leave enough time to intercept the unknown flying objects, the system needs to "observe" and "report" the objects as early as possible. Due to the long distance and complex background, it is hard to find and locate the small and dim targets. To tackle this difficult task, we propose a hybrid feature extraction network, taking advantages of both convolution and self-attention mechanism. Besides, we use the two-dimension Gaussian distribution to represent the bounding-box, which is convenient to measure the distance between the predicted result and the ground truth comparing to the Intersection over Union measurements. Finally, we also apply multiple data augmentation and training techniques to upgrade the detection performance. To verify effectiveness and efficiency of our method for infrared small target detection, we conduct extensive experiments on a public infrared small target dataset. The experimental results show that the model trained by our method has a significant improvement in detection accuracy and speed compared with other data-based target detection algorithms, with the average precision reaching more than 92%. The proposed method can effectively detect infrared dim-small targets in different complex backgrounds with low false alarm rate and missing alarm rate. It can also achieve outstanding performance in general small object datasets, verifying the effectiveness and robustness of our method.
MoreTranslated text
Key words
Infrared small target detection, convolution network, self-attention, 2D Gaussian distribution
AI Read Science
Must-Reading Tree
Example
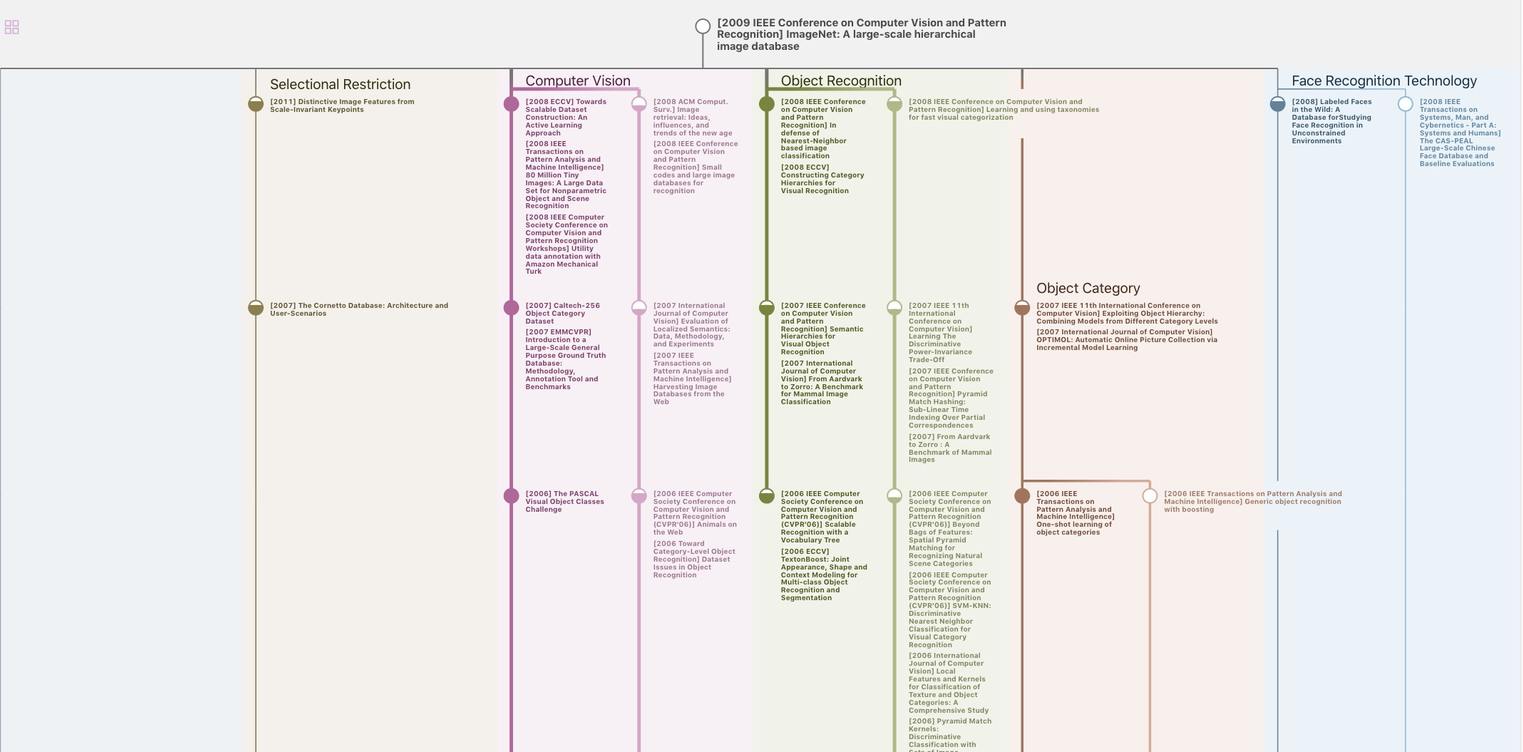
Generate MRT to find the research sequence of this paper
Chat Paper
Summary is being generated by the instructions you defined