Deep Learning Model on 2-Dimensional Image Data Using Convolutional Autoencoder and Fully Connected Neural Networks: Application to Computational Fluid Dynamics
SSRN Electronic Journal(2023)
Abstract
Abstract This study proposes a deep-learning-based image prediction meta-modeling method to develop an image-based approximate optimized design using 2D image data. An image-based meta-model is generated with an autoencoder (AE) and fully connected neural networks (FNN). To create this meta-model, we suggested three methods as FNN-based AE, convolutional autoencoder (CAE) based on convolution neural networks (CNN), and hybrid-convolutional autoencoder (H-CAE) combining the FNN and CAE. To verify the proposed methods, we applied them to predict the pressure distribution for around a 2-dimensional airfoil to replace the computational fluid dynamics simulation. As a result, the H-CAE among the proposed methods shows the high prediction accuracy, 99.9811% of the best image reconstruction rate for the pressure distribution around the airfoil. Therefore, H-CAE offers the best learning capability. There is an advantage that the latent can be predicted using shape parameters of an airfoil as inputs via the FNN based on the latent with the compressed image data.
MoreTranslated text
Key words
computational fluid dynamics,convolutional autoencoder,deep learning,connected neural networks
AI Read Science
Must-Reading Tree
Example
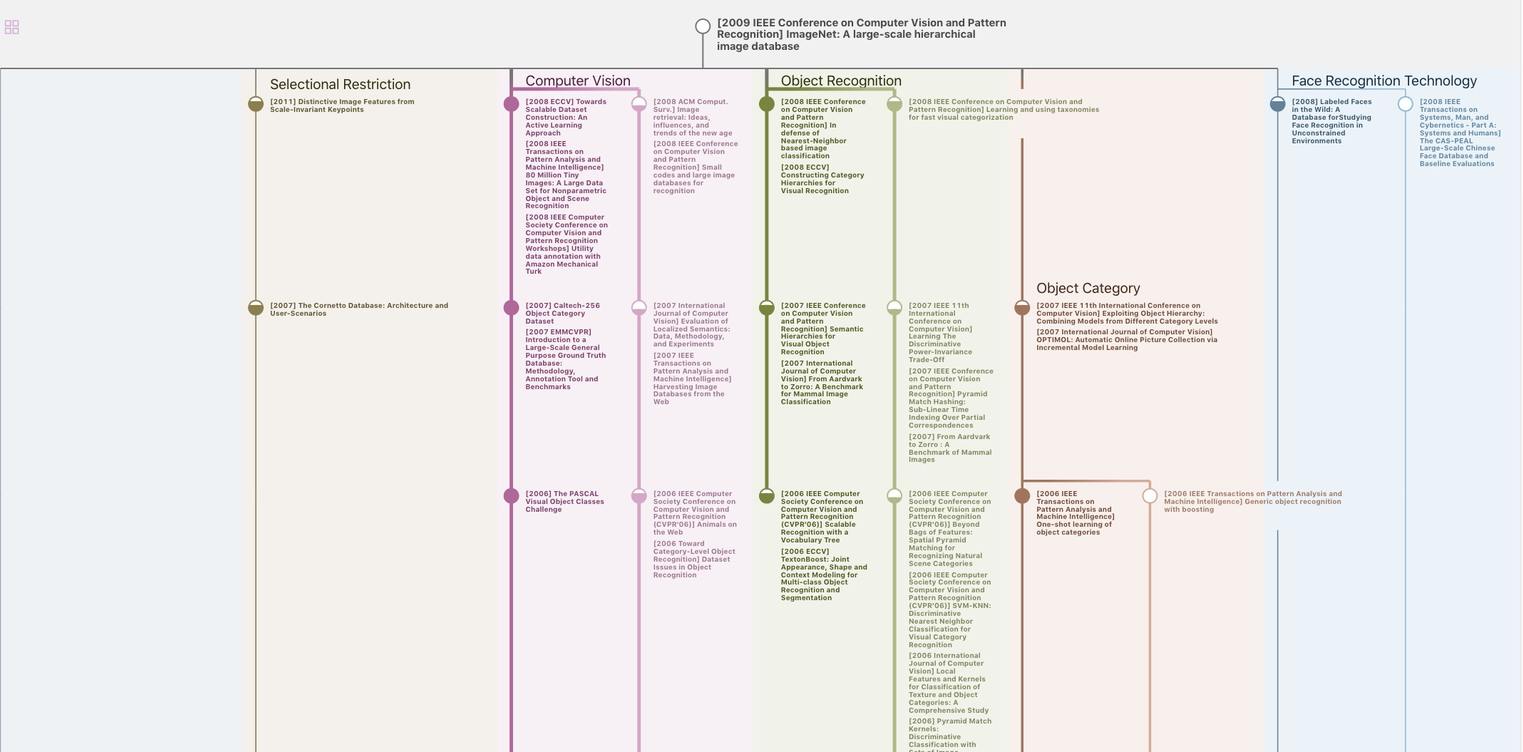
Generate MRT to find the research sequence of this paper
Chat Paper
Summary is being generated by the instructions you defined