1290 A deep learning analysis pipeline for multiplex imaging identifies spatial features associated with clinical outcome in colorectal cancer
Regular and Young Investigator Award Abstracts(2022)
摘要
BackgroundMultiplex immunofluorescence (mIF) can provide invaluable insights on spatial biology and the complexities of the immune tumor microenvironment (iTME), however, current analysis methods are laborious and user dependent. We applied a novel end-to-end deep learning (DL) pipeline (figure 1) to mIF tumor-microarray (TMA) images, to investigate associations between iTME composition and clinical outcome.MethodsA publicly available CODEX dataset,1 consisting of 140 tissue cores from 35 colorectal cancer (CRC) patients stained with 56 protein markers and matched H&E slides was analyzed. 7,000 cell annotations on 57 tissue cores were used to train DL models to segment cells and classify them to 13 subpopulations (figure 2). Performance was evaluated quantitatively on 1,800 annotations from 14 test cores, and qualitatively on all cores by expert pathologists. In addition, positivity for 8 immunomodulatory markers was predicted per cell, using a DL binary classifier trained on 100,000 binary single channel annotations, which were automatically extracted from the 7,000 cell annotations by prior knowledge of lineage marker expression. The model performance was evaluated on novel annotations for each phenotypic marker. H&E slides were utilized to annotate tumor and stromal areas and identify fibroblasts and marker-negative tumor cells. 12 Cell neighborhoods were identified by clustering the 10 nearest-neighbors for each cell (figure 3). Over 600 spatial features were calculated and correlated with good vs. bad prognosis defined by 2-year OS cutoff.ResultsCell typing model reached a 91.9% accuracy (figure 4) and good performance (>75% accuracy) by qualitative assessment in 97.7% of cores, thus markedly outperforming existing clustering-based cell typing approaches showing 65.9% accuracy (figure 5). Phenotypic marker classification demonstrated 93.5% accuracy (figure 6). Stromal abundance of a plasma cell-enriched neighborhood was associated with bad prognosis (p=0.009), while higher fraction of LAG3+ (p=0.01) and VISTA+ (p=0.004) plasma cells in the same neighborhood was associated with good prognosis. In addition, LAG3+CD4+ T-cells upregulation in a CD8+ enriched neighborhood was also associated with better prognosis (p=0.006, figure 7 and table 1). Taken together, we demonstrate the importance of complex spatial features, which capture cell type, neighborhood and functional state information for clinical outcome prediction.ConclusionsA novel DL pipeline for mIF analysis demonstrated high accuracy in classifying cell types and phenotypic markers, thus enabling the identification of multiple cellular and spatial features associated with prognosis in CRC.ReferenceSchürch CM, Bhate SS, Barlow GL, et al. Coordinated cellular neighborhoods orchestrate antitumoral immunity at the colorectal cancer invasive front [published correction appears in Cell. 2020 Oct 29;183(3):838]. Cell. 2020;182(5):1341–1359.e19. doi:10.1016/j.cell.2020.07.005
更多查看译文
关键词
deep learning analysis pipeline,colorectal cancer,multiplex imaging,spatial features
AI 理解论文
溯源树
样例
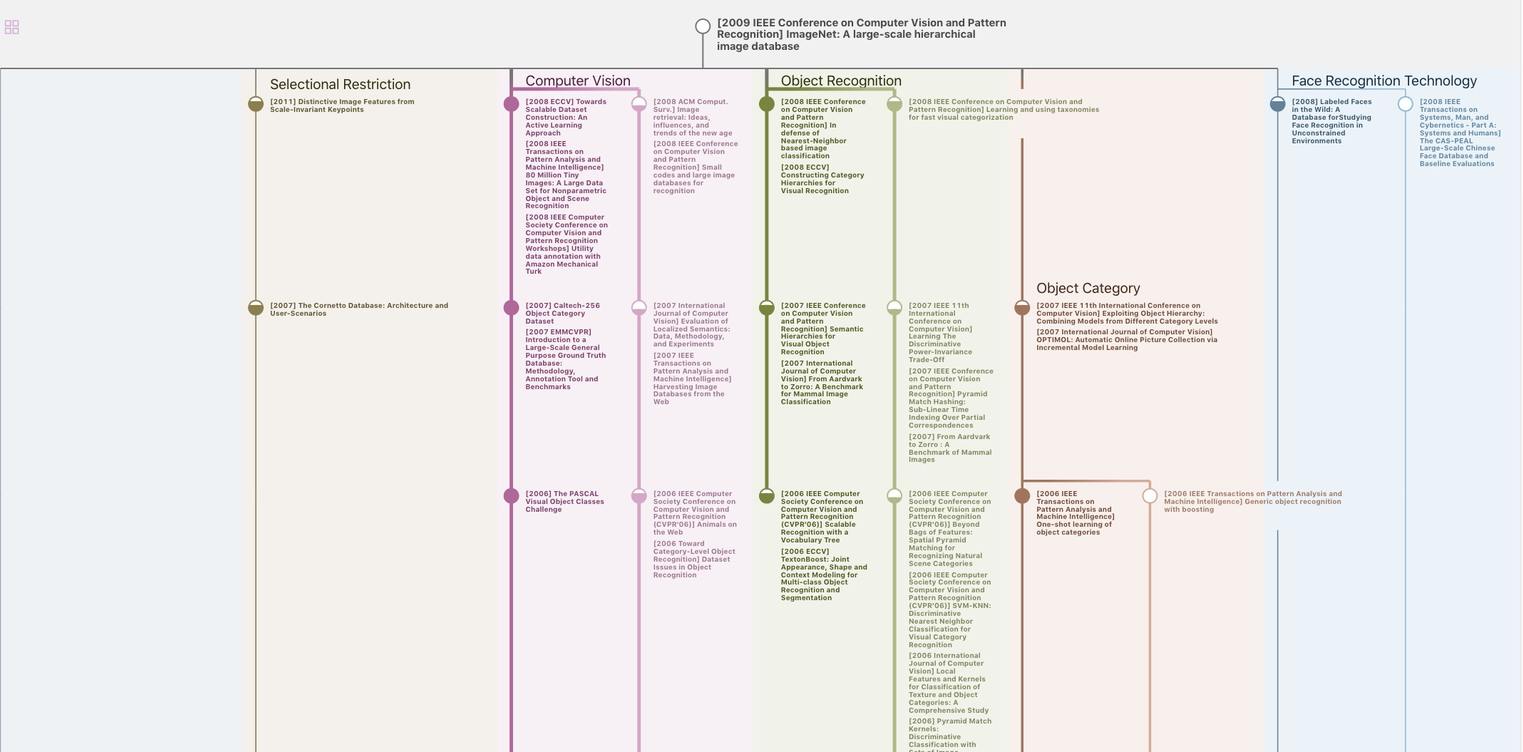
生成溯源树,研究论文发展脉络
Chat Paper
正在生成论文摘要