Rethinking Position Embedding Methods in the Transformer Architecture
Neural Processing Letters(2024)
Abstract
In the transformer architecture, as self-attention reads entire image patches at once, the context of the sequence between patches is omitted. Therefore, the position embedding method is employed to assist the self-attention layers in computing the ordering information of tokens. While many papers simply add the position vector to the corresponding token vector rather than concatenating them, few papers offer a thorough explanation and comparison beyond dimension reduction. However, the addition method is not meaningful because token vectors and position vectors are different physical quantities that cannot be directly combined through addition. Hence, we investigate the disparity in learnable absolute position information between the two embedding methods (concatenation and addition) and compare their performance on models. Experiments demonstrate that the concatenation method can learn more spatial information (such as horizontal, vertical, and angle) than the addition method. Furthermore, it reduces the attention distance in the final few layers. Moreover, the concatenation method exhibits greater robustness and leads to a performance gain of 0.1–0.5% for existing models without additional computation overhead.
MoreTranslated text
Key words
Transformer,Position embedding,Addition method,Concatenation method
AI Read Science
Must-Reading Tree
Example
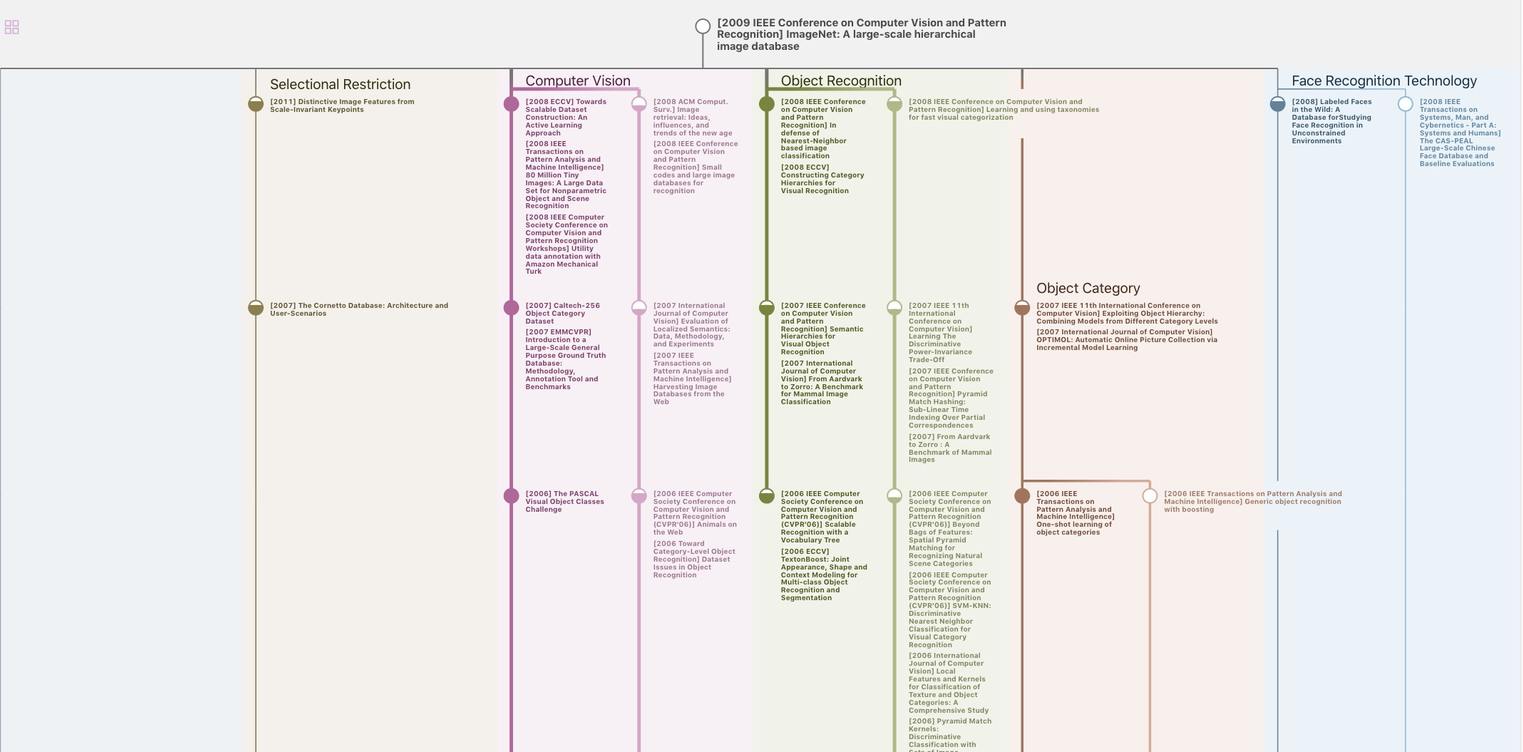
Generate MRT to find the research sequence of this paper
Chat Paper
Summary is being generated by the instructions you defined