Spatial-Temporal Graph Auto-Encoder for Traffic Flow Prediction
SSRN Electronic Journal(2022)
摘要
Accurate traffic flow prediction is crucial for building Intelligent Transportation System. However, the prediction task is challenging due to the problems that (i) the abnormality of traffic data influences model training, (ii) the existing graph methods cannot well encode the complex multilayer spatial dependence rules on the topological graph, and (iii) the nonlinear temporal dynamics of road conditions complicate long-term prediction. To this end, we propose a novel traffic flow prediction model named Spatial-Temporal Graph Auto-Encoder (STGAE). The model integrates Multilayer Graph Attention Adjacency Network (MGAAN) and Gated-Memory Convolutional Neural Network (GMCNN) into the end-to-end framework and is trained by Network Smoothing Training (NST). Specifically, the NST method applies the improved smoother, Historical-Average-Based Rauch-Tung-Striebel (HA-RTS), to smooth the noise of the input and target data for improving robustness. MGAAN adaptively generates a multilayer attention adjacency matrix by combining the prior information about graph topology and a multilayer self-attention mechanism to model multilayer dynamic spatial dependencies. GMCNN introduces a novel gating mechanism of full convolution architecture into the convolutional neural network, which can provide parallel computing and memory processing for serialized hidden features. The experimental results demonstrate that STGAE is more predictive and efficient than baseline models with big traffic data.
更多查看译文
关键词
traffic flow prediction,spatial-temporal,auto-encoder
AI 理解论文
溯源树
样例
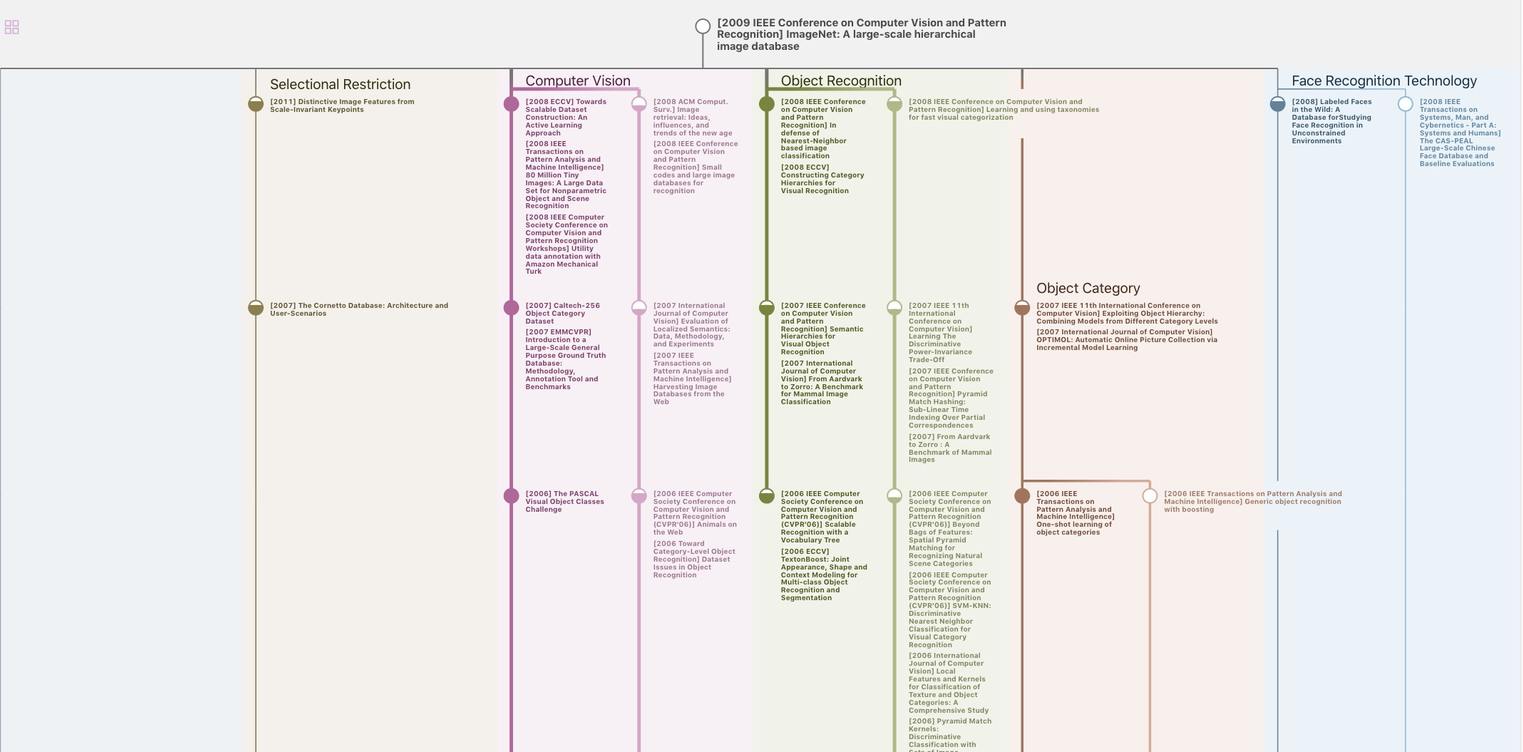
生成溯源树,研究论文发展脉络
Chat Paper
正在生成论文摘要