Learning Light Field Macro-Gepi Representation for Semantic Segmentation
SSRN Electronic Journal(2023)
摘要
2D optical imaging systems cause irreparable stereo visual feature loss in the pixel-level semantic segmentation task, especially in challenging scenes. To overcome this limitation, light field imaging with microlens arrays can acquire the 4D multi angle subaperture images, providing a viable alternative to semantic segmentation. However, these images are redundant and difficult to process. Therefore, we propose a macro generalized epipolar-plane image (macro-GEPI) representation. The macro-GEPI is obtained by slicing the subaperture im-ages, stacking the slice images in sequence, and finally aligning them. This new image representation can clearly highlight the spatial-angular interactive information of different objects in one image. Based on this, we propose a convolutional neural network (CNN) that can independently learn the light field spatial-angular information for semantic segmentation. We also present a light field dataset composed of 1840 macro-GEPIs with annotations. We have studied the proposed network and compared it to state-of-the-art (SOTA) algorithms. The experiments showed that our network outperformed SOTA algorithms on the macro-GEPI dataset and a benchmark light field dataset, especially in challenging scenes. Moreover, our algorithm achieved a high mean intersection over union of 90.83%, demonstrating the reliability of the light field macro-GEPI representation and the effectiveness of our CNN algorithm.
更多查看译文
关键词
light field,semantic segmentation,learning,macro-gepi
AI 理解论文
溯源树
样例
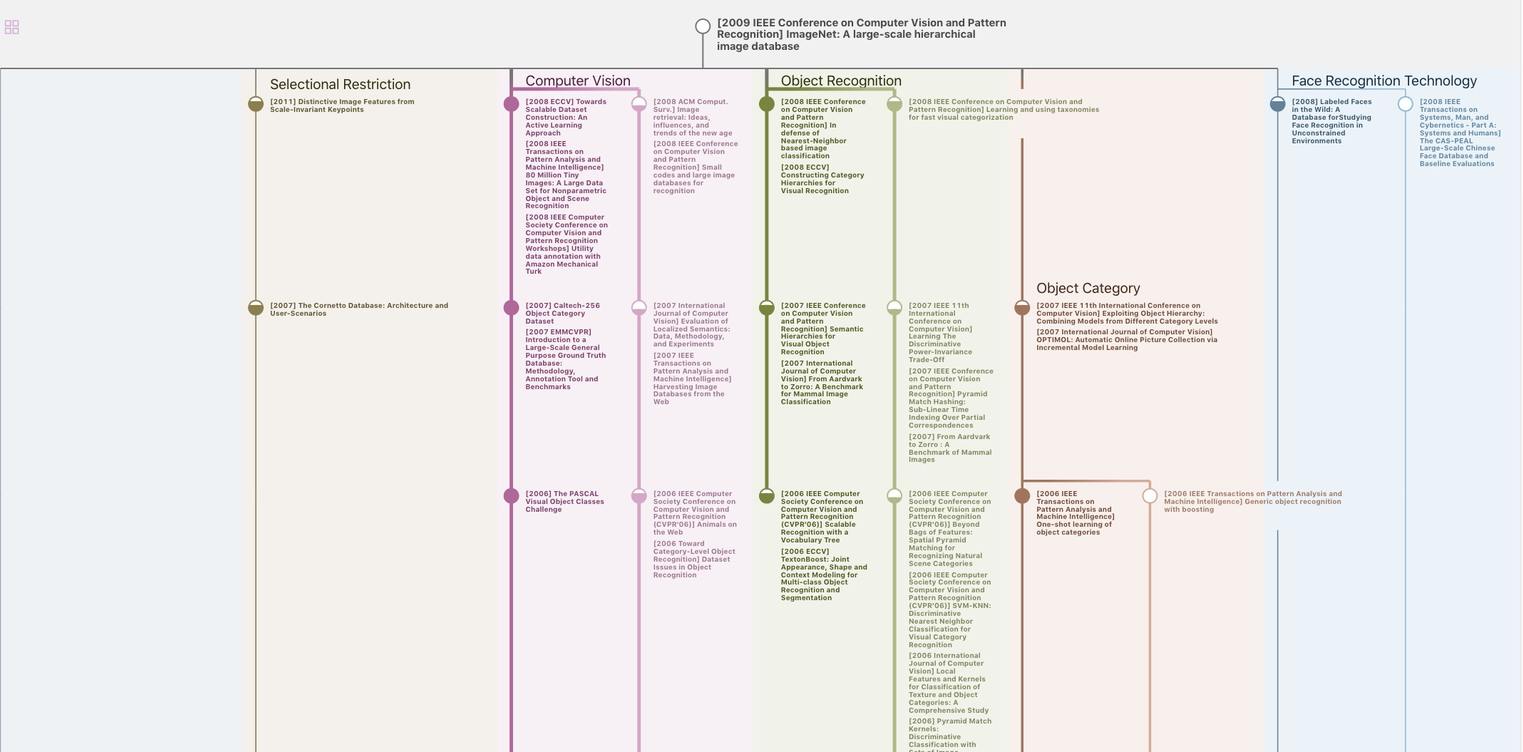
生成溯源树,研究论文发展脉络
Chat Paper
正在生成论文摘要