Adaptative generalisation over a value hierarchy for the k-anonymisation of Origin-Destination matrices
TRANSPORTATION RESEARCH PART C-EMERGING TECHNOLOGIES(2023)
摘要
The study of transportation relies on mobility data, containing information on the whereabouts and movements of individuals in a study area. These data are often represented in the simple form of origin-destination (OD)-matrices, which are a valuable indicator for the management of transportation networks but also present risks to the privacy of the individuals. In particular, the significant size (i.e., number of distinct flows) and high number of modalities (produced by a high-resolution zoning) of OD-matrices call for an adapted, fast algorithm that can efficiently anonymise them. In this paper, we develop a lightweight approach for the k-anonymisation of OD-matrices that exploits the low dimension of the data to explore a larger solution space than regular generalisation algorithms, while keeping relevant restrictions of the search space in order to be scalable on matrices with high number of flows. We apply it to a variety of real world large-scale O-D matrices collected by the New York City Taxi and Limousine Commission and derived from the Data for Development (D4D) challenge organised by Orange in Senegal and Cote d'Ivoire. Compared to an extensive benchmark of regular generalisation algorithms and mobility anonymisation state-of-the-art, we show that our method is 27% more precise and 9 times faster than comparable approaches able to scale on the same datasets.
更多查看译文
关键词
Origin-Destination matrix,Anonymisation,Generalisation,Suppression
AI 理解论文
溯源树
样例
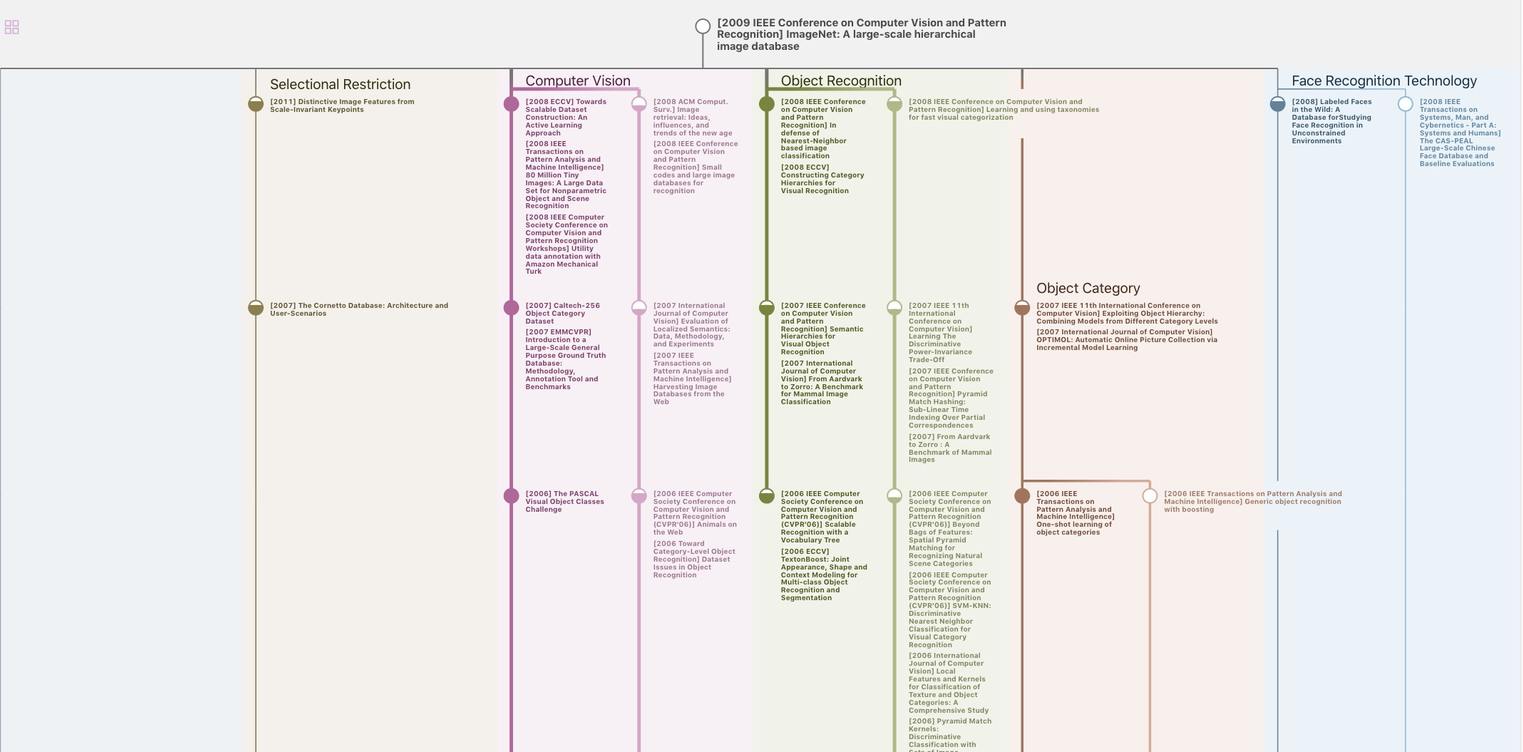
生成溯源树,研究论文发展脉络
Chat Paper
正在生成论文摘要