Abstract WMP58: Scaling Behaviors Of Deep Learning And Linear Algorithms For The Prediction Of Stroke Severity
Stroke(2023)
摘要
Introduction: Deep learning (DL) has allowed for substantial progress in many, often machine vision-focused medical scenarios. Since DL models typically require 10 5 -10 7 examples, it is currently unknown whether DL can also enhance predictions of outcomes post-stroke in real-world samples of stroke patients that are several magnitudes smaller. We here compared the capacities of linear and DL algorithms regarding their prediction of stroke severity. Methods: Our analyses relied on a total of 1435 patients assembled from the MRI-GENIE study and a Massachusetts General Hospital-based study. The primary outcome was acute NIHSS-based stroke severity, which we predicted by means of lesion location. We automatically derived lesion segmentations from DWI scans, performed spatial normalization and principal component analysis (PCA), retaining 95% of the variance of the original data. We then separated a train, validation, and test set, of sizes 918, 230, and 287 respectively. We downsampled the train set to 100, 300, and 900 and learned to predict the NIHSS score for each sample size with regularized linear regression and an 8-layered neural network. We selected hyperparameters on the validation set and conducted 500 dataset splits. We here report explained variance (R-squared) in the test set. Results: While linear regression performed favorably for a sample size of 100 patients (R-squared=0.28), DL started to significantly outperform linear regression when trained on 900 patients (R-squared=0.34, Figure 1 ). Average prediction performance improved by ~20% when increasing the sample size 9x. Conclusions: For sample sizes of ~1000 patients, DL showed a more convincing prediction performance for stroke severity than typically employed linear methods. Our findings suggest the existence of non-linear relationships between lesion location and outcome post-stroke that can be utilized for an improved prediction performance once samples are reasonably large.
更多查看译文
关键词
stroke,deep learning,abstract wmp58,prediction
AI 理解论文
溯源树
样例
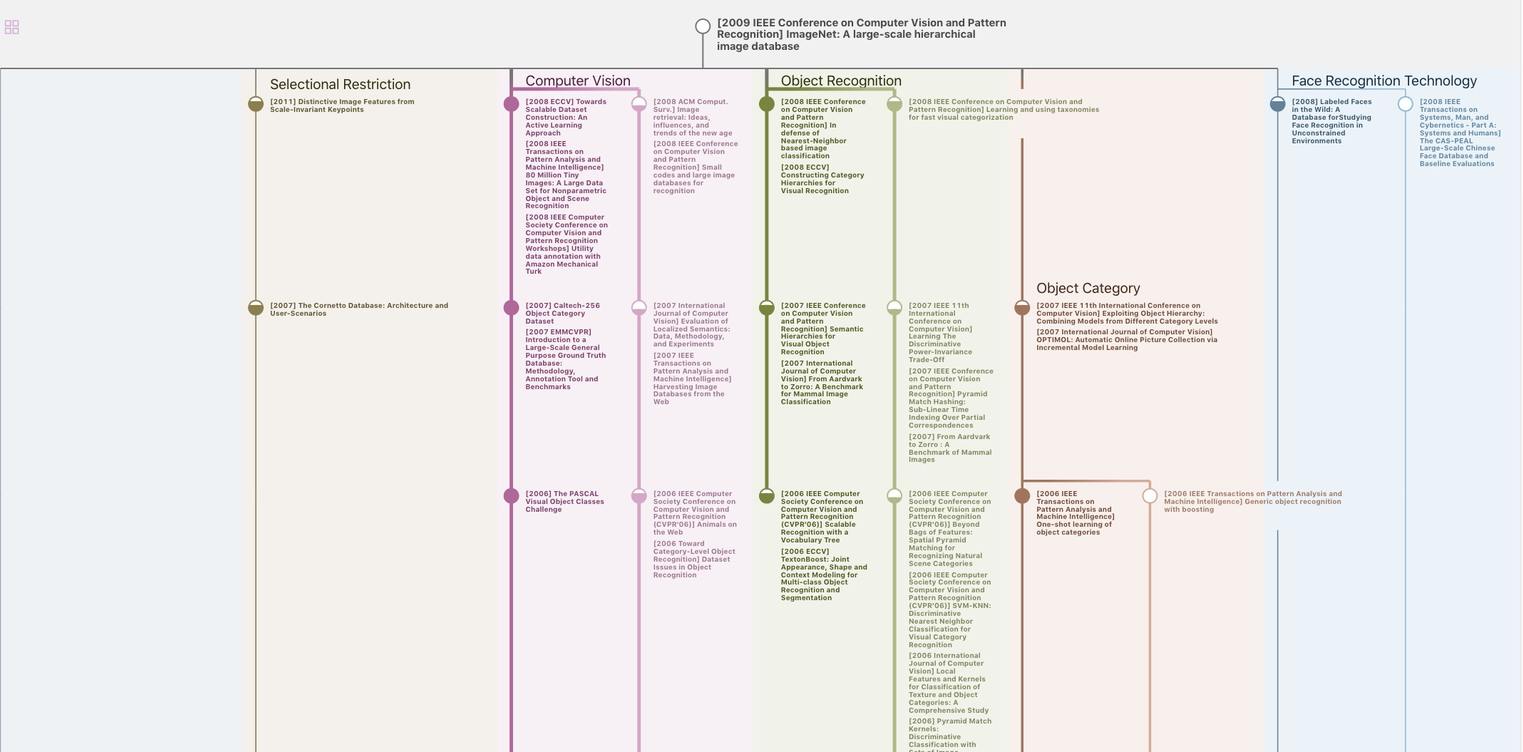
生成溯源树,研究论文发展脉络
Chat Paper
正在生成论文摘要