Novel Imaging-based Morphological Markers For Improved Prediction Of Stroke Outcomes: A Machine Learning Approach
Stroke(2023)
摘要
Patient selection for acute reperfusion therapies of acute ischemic stroke is based on assessment of the core and tissue-at-risk and the time from stroke onset. Response to treatment and clinical outcomes, however, vary significantly since patient-specific cerebrovascular anatomy plays a vital role in governing flow and reperfusion. We developed an end-to-end automated machine learning approach to extract advanced cerebrovascular morphological features, including tortuosity and collateral index, to improve outcome prediction, and patient selection. Methods: Using our validated automatic cerebrovascular segmentation and feature extraction algorithm, we obtained the morphological properties of the brain vasculature, including vessel tortuosity, length, diameter, and bifurcation patterns, in MR angiography scans of 100 anonymized stroke patients. Each patient’s collateral index was also automatically graded using a previously established probabilistic atlas of healthy cerebrovasculature. To predict 90-day functional outcomes (mRS), a statistical model was trained using patients' clinical features (demographics, comorbidities, and baseline NIHSS), imaging features (initial ASPECTS, perfusion mismatch volume, and collateral index), and the extracted morphologic features (length, diameter, number of branches, vessel tortuosity, fractal dimension, and total volume). The predictions were compared against ground truth mRS to assess model accuracy. We also compared the model’s performance against conventional outcome predictors with only the clinical and imaging features. Results: The conventional model including the common clinical and imaging features excluding the collateral index gave an area under the curve (AUC) of 0.63, which is comparable to previously published results. Including the automatically scored collateral index improved the accuracy to 0.74. We found that further including the morphological features to the predictor model significantly improved AUC accuracy to 0.83. Conclusion: Including automatically extracted cerebrovascular morphologic features in traditional clinical and imaging markers significantly improves outcome prediction and response to treatment in stroke patients.
更多查看译文
AI 理解论文
溯源树
样例
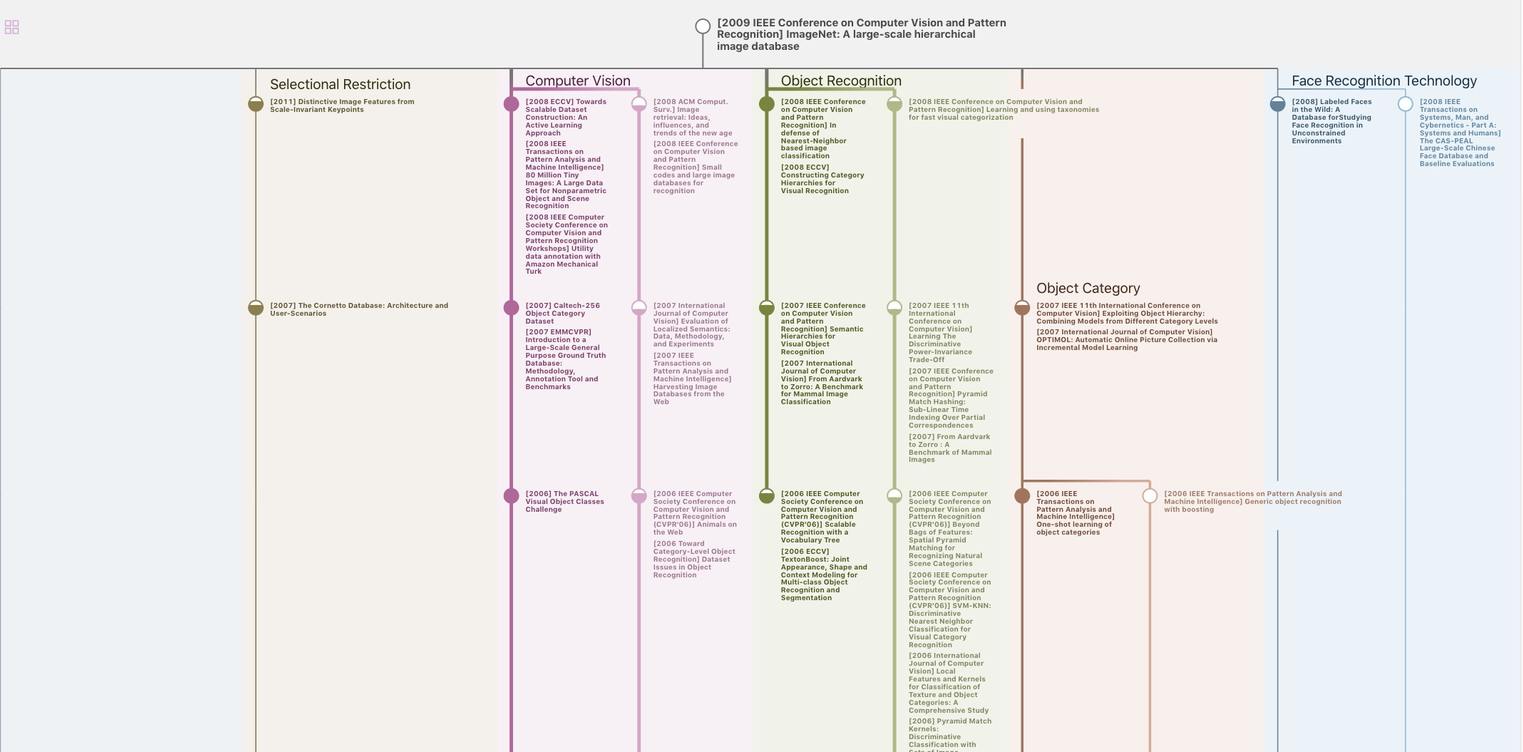
生成溯源树,研究论文发展脉络
Chat Paper
正在生成论文摘要