Auxiliary-lag Dependent Gaussian Process Model for Forecasting Rainfall Data Using Proposed Kernels and Multi-start Optimization Method
crossref(2023)
摘要
Abstract Pakistan is currently facing the biggest flood of history due to monsoon rains. The rainfall forecasting is very important for policy making. In this paper, we have presented an auxiliary-lag dependent Gaussian process, a Bayesian non-parametric machine learning model, for modeling the rainfall data using auxiliary lags. We have also introduced some new multifeatured kernel functions that are versatile in dealing with seasonal data. A simplex cluster-based multi-start technique using the Nelder-Mead optimizer has also been proposed for optimizing the hyperparameters of the kernel functions, which can be used for any available or proposed kernel function(s). For comparison of the proposed model, we have used the autoregressive random forest model, autoregressive artificial neural network model, seasonal autoregressive moving average models, and exponential smoothing models. Results confirmed the superiority of the proposed model over conventional models. The proposed methodology will be helpful for other researchers and local experts in making more reliable forecasting which will be helpful in policymaking relevant to agriculture systems, water management systems, climate change, and natural disasters such as droughts and floods.
更多查看译文
AI 理解论文
溯源树
样例
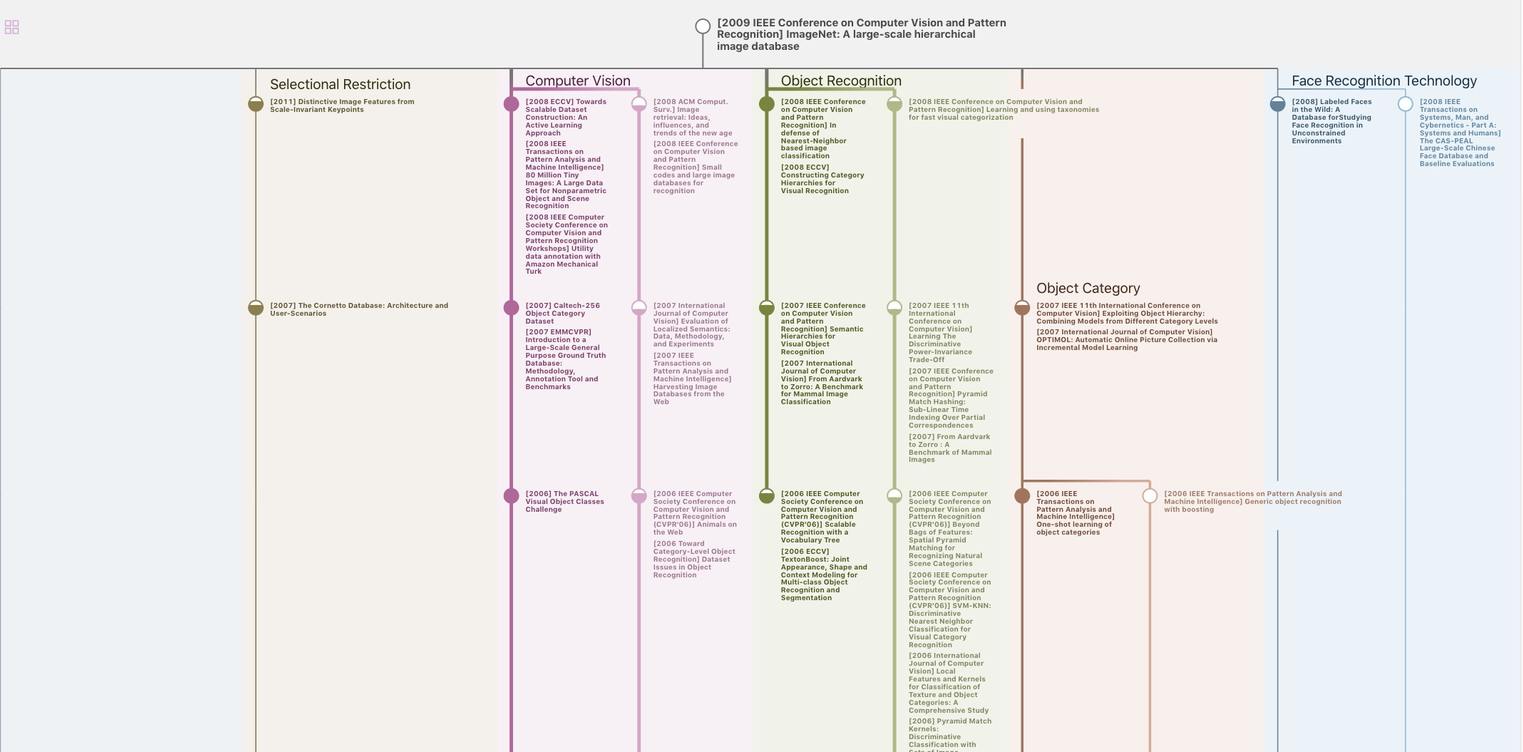
生成溯源树,研究论文发展脉络
Chat Paper
正在生成论文摘要