A sequential decision making approach to learn process parameters by conducting experiments on sacrificial objects
PROCEEDINGS OF ASME 2022 INTERNATIONAL DESIGN ENGINEERING TECHNICAL CONFERENCES AND COMPUTERS AND INFORMATION IN ENGINEERING CONFERENCE, IDETC-CIE2022, VOL 2(2022)
摘要
Learning the right process parameters is essential to efficiently and safely execute tasks in automated manufacturing applications. When there is a risk of damaging the object, conducting experiments on sacrificial objects is a good option to explore the process parameters before performing the tasks on the object of interest. However, using too many sacrificial objects can incur unnecessary costs. We want to know the right amount of sacrificial objects to identify the right process parameters while keeping the expected task completion cost to a minimum. To do this, we use a sequential decision making approach to learn the process parameters. The sequential decision making approach we proposed is a combination of look ahead search, surrogate modeling, and a policy to select process parameters. In computation, the search tree simulates future decisions beyond the current decision and evaluates the costs by considering the effect of future experiments. The decision made in our approach only commits the user to the current stage and does not affect future decisions. When a decision is made to conduct experiments on the sacrificial object, the parameter policy to select process parameters should be simulated. In this policy, we consider the right trade-off between exploration and exploitation. In the beginning, the policy favors exploration since we do not have much data. In later stages, less exploration and more exploitation will be performed as we have sufficient number of experimental data. We validate our approach using the experimental setup of the robotic spray painting application.
更多查看译文
AI 理解论文
溯源树
样例
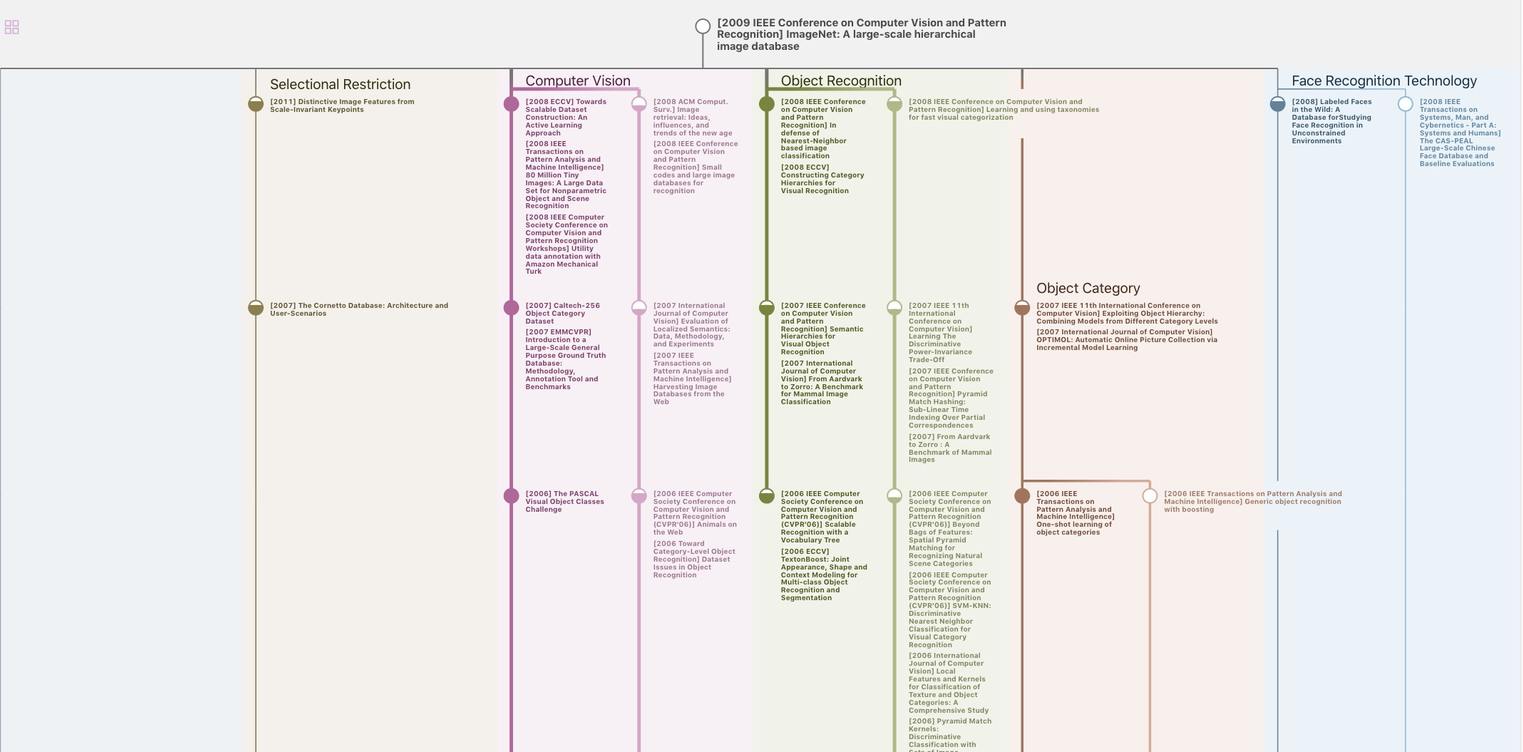
生成溯源树,研究论文发展脉络
Chat Paper
正在生成论文摘要