A Probabilistic Model to Estimate Automated and Manual Visual Inspection Errors
Lecture Notes in Mechanical EngineeringFlexible Automation and Intelligent Manufacturing: The Human-Data-Technology Nexus(2023)
摘要
Primary risks associated with visual inspection are missing a defect (Type II error) and identifying a defect that is a false alarm (Type I error). The focus of this paper is on defect detection on cylinder head surfaces using optical inspection techniques. Several factors were included in the study for their impact on defect identification: lighting, lens angle, camera distance, defect dimensions, and defect accessibility. Using the influence diagram technique, we used quantified uncertainties to determine how these factors affect Type I and Type II errors. For some defects, there can be significant variation in inspection performance results due to subjective judgment of inspectors. We also analyzed the reduction in Type II errors using a machine learning methodology to identify and classify defects. The influence diagram model is populated with data from an experimental study and subject matter expert (SME) input. Presented results may be used to identify and assess factors that can influence success of hybrid inspection processes and mitigate associated risks of Type I and Type II errors.
更多查看译文
关键词
estimate automated,probabilistic model
AI 理解论文
溯源树
样例
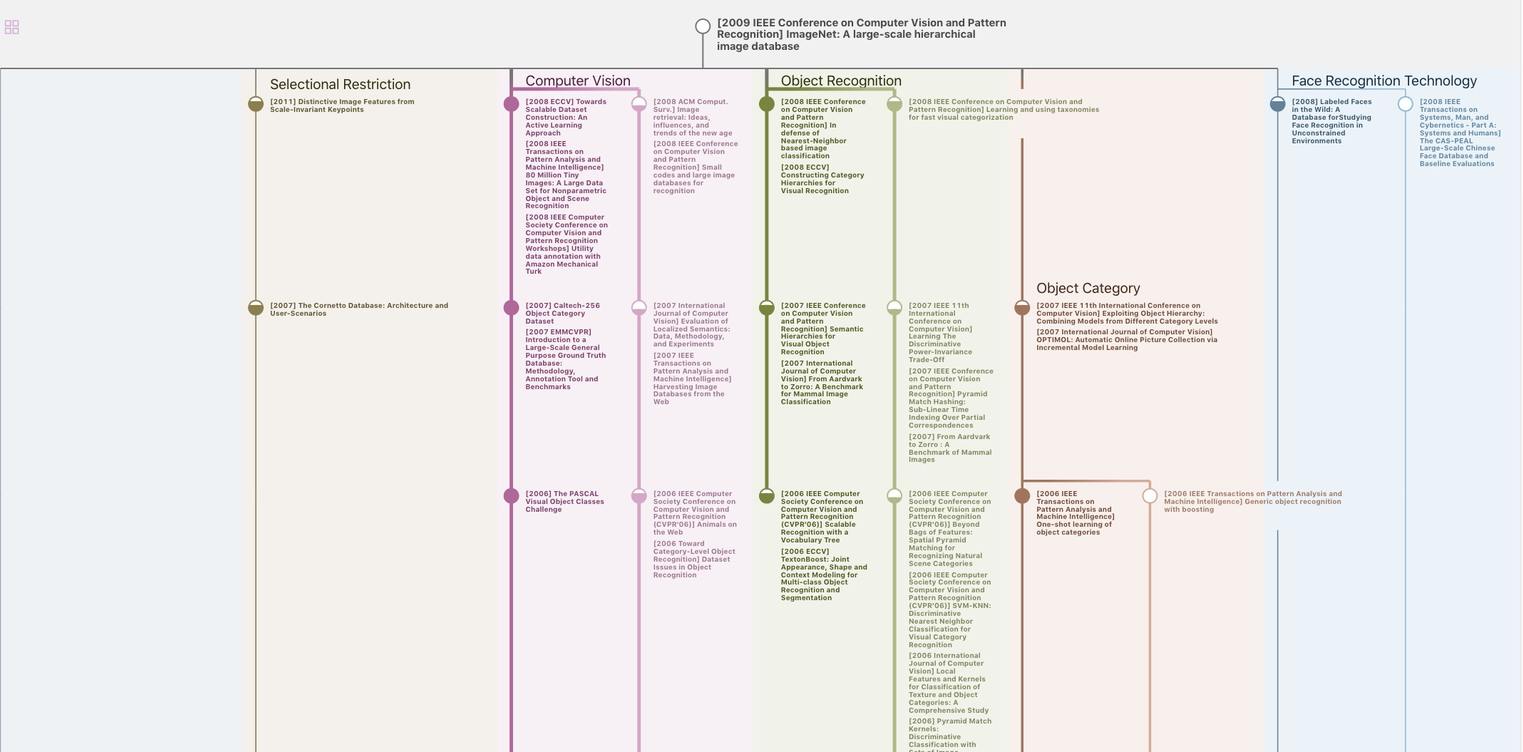
生成溯源树,研究论文发展脉络
Chat Paper
正在生成论文摘要