Towards a Due Foundation for Image Based Discrimination of Multiple Sclerosis Disease Types Using Magnetic Levitation and Vision Intelligence
crossref(2022)
摘要
The emerging advancements in separation and classification of various biological matters (e.g., living cells and proteins) using magnetic levitation (MagLev) technology have proven to be effective for improving disease diagnostics. MagLev technique has the capacity to detect and separate useful diagnostic biomarkers from biocomplex environments (e.g., blood and plasma), minimizing the unpleasant daunting task of sample preparations and labeling procedures. Here, we demonstrate the capability of this technique combined with image analysis and machine learning approaches for discriminating the various types of multiple sclerosis (MS) as an important model disease. To arrive at a systematic expert system, we combined robust statistical analysis with machine learning to (1) detect and remove outliers from the raw MagLev image datasets; then (2) process the images and output a low dimensional representation of massive data without losing the main statistical features; and finally (3) predict the MS clinical disease type (Relapsing-Remitting, Primary–Progressive, or Secondary–Progressive) using a classifier. This is expected to improve MS diagnostics since the current practices rely solely on clinical observation and central nervous system imaging, making management approaches are often reactional and inefficient. Thus, there is a need to identify the disease type early on. MagLev is expected to improve MS diagnostics, thereby aiding in prognosis and guiding adequate treatment choices before the patient exhibits signs of permanent neurological deficits.
更多查看译文
AI 理解论文
溯源树
样例
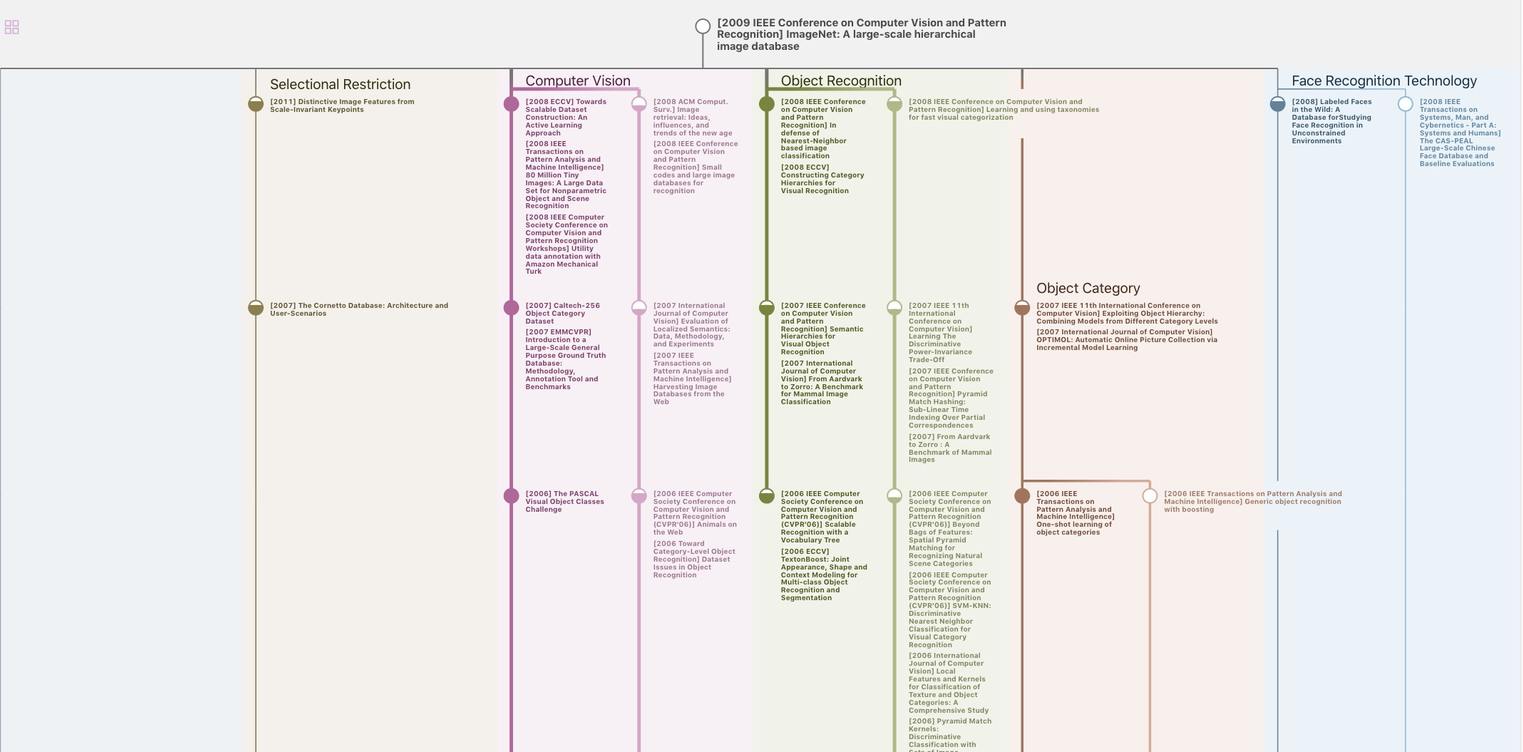
生成溯源树,研究论文发展脉络
Chat Paper
正在生成论文摘要