My experience of working for the JE-1991-2013
JOURNAL OF ECONOMETRICS(2023)
摘要
We propose a method to conduct uniform inference for the (optimal) value function, that is, the function that results from optimizing an objective function marginally over one of its arguments. Marginal optimization is not Hadamard differentiable (that is, compactly differentiable) as a map between the spaces of objective and value functions, which is problematic because standard inference methods for nonlinear maps usually rely on Hadamard differentiability. However, we show that the map from objective function to an Lp functional of a value function, for 1≤p≤∞, are Hadamard directionally differentiable. As a result, we establish consistency and weak convergence of nonparametric plug-in estimates of Cramér–von Mises and Kolmogorov–Smirnov test statistics applied to value functions. For practical inference, we develop detailed resampling techniques that combine a bootstrap procedure with estimates of the directional derivatives. In addition, we establish local and uniform size control of one-sided tests which use the resampling procedure. Monte Carlo simulations assess the finite-sample properties of the proposed methods and show accurate empirical size and nontrivial power of the procedures. Finally, we apply our methods to the evaluation of a job training program using bounds for the distribution function of treatment effects.
更多查看译文
AI 理解论文
溯源树
样例
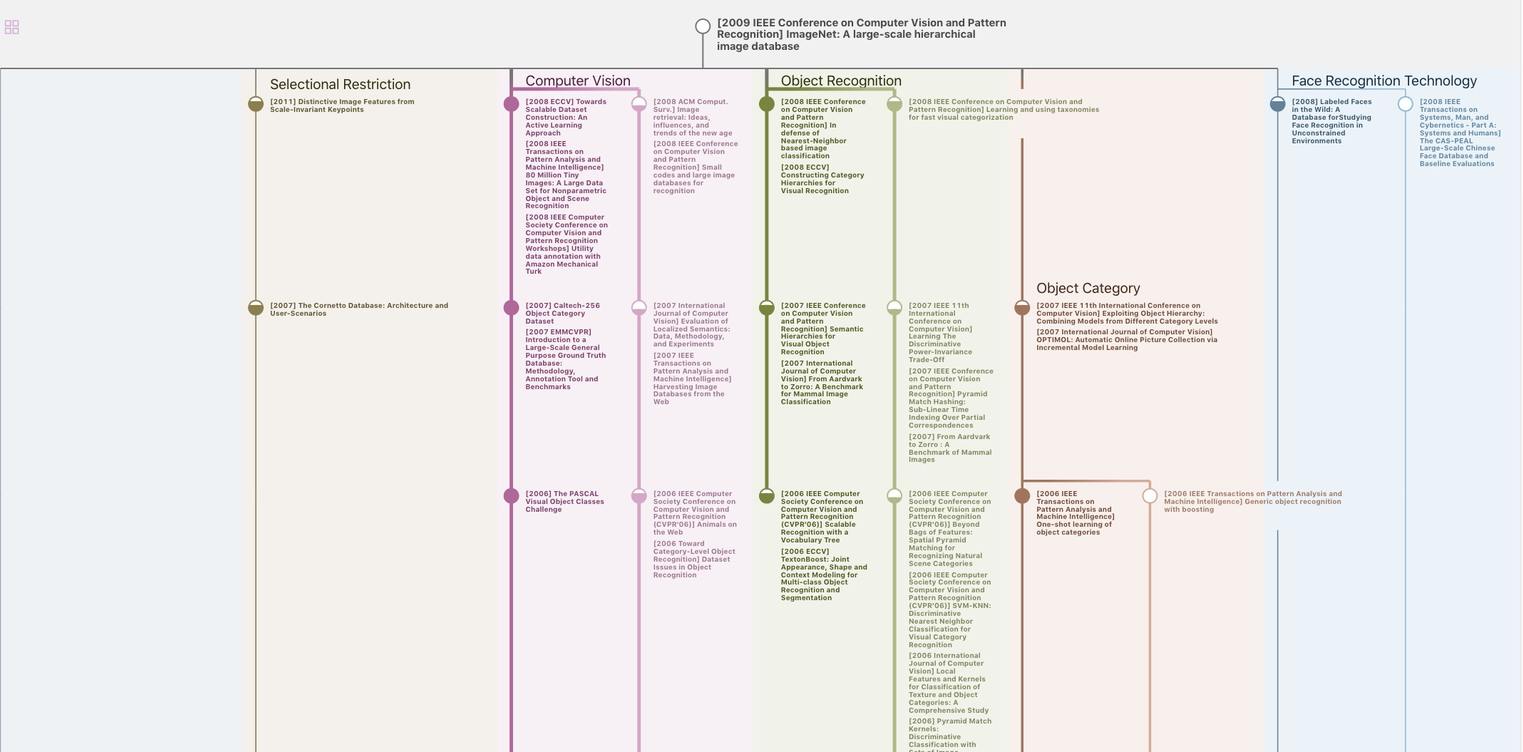
生成溯源树,研究论文发展脉络
Chat Paper
正在生成论文摘要