Neural Networks Based Throughput Estimation of Short Production Lines Without Intermediate Buffers
Intelligent Systems Reference LibraryFusion of Machine Learning Paradigms(2023)
摘要
The accurate throughput estimation of a production line steadily attracts research interest due to its high impact on their economic operation affecting the world production of goods. Since Artificial Intelligence (AI) is embedded in production processes leading to Industry 4.0, it is as well used for throughput estimation. Neural Networks (NN) are a popular and well-established and tested intelligent methodology capable of performing at an excellent level as function approximation techniques for complex domains. In this paper different neural network architectures as well as deep learning approaches have been tested on datasets corresponding to accurate solutions of throughput taken from exact algorithms existing in literature for relatively small size serial production lines without buffers. Three main subproblems are studied in the paper, those of three, four and five workstations without intermediate buffers. The idea is to train a neural network architecture over each dataset corresponding to a specific problem and obtain generalized models of high accuracy, able to accurately calculate throughput for unknown settings and parameters of similar characteristics and parameters within the training range (i.e., the mean service rate) but also out of it. Performance deteriorates as we move away from the training range, a fact that leads to the conclusion that the expert should know the basic operating characteristics and properties of a specific production line, prior to setting the training interval into which the neural network should be trained, and then be used for throughput approximation. It is interesting that the datasets used for training are very small compared to those used for validation, which proves the high neural network generalization power. The performance of the neural network approach is compared to a previously applied genetic programming (GP) approach and it proves that it performs competitively, and in some cases slightly better within the training range, but it worsens more quickly than GP’s performance as we move away from the training range. Results seem to be encouraging for future research in throughput approximation using neural networks regarding longer size production lines, if (a) standard algorithms for accurate throughput estimation such as Markov and DECO-2 are used for training data generation, and (b) in combination with the design of proper sampling techniques that will ensure maximum representativeness of the training data used.
更多查看译文
关键词
short production lines,neural networks,intermediate buffers
AI 理解论文
溯源树
样例
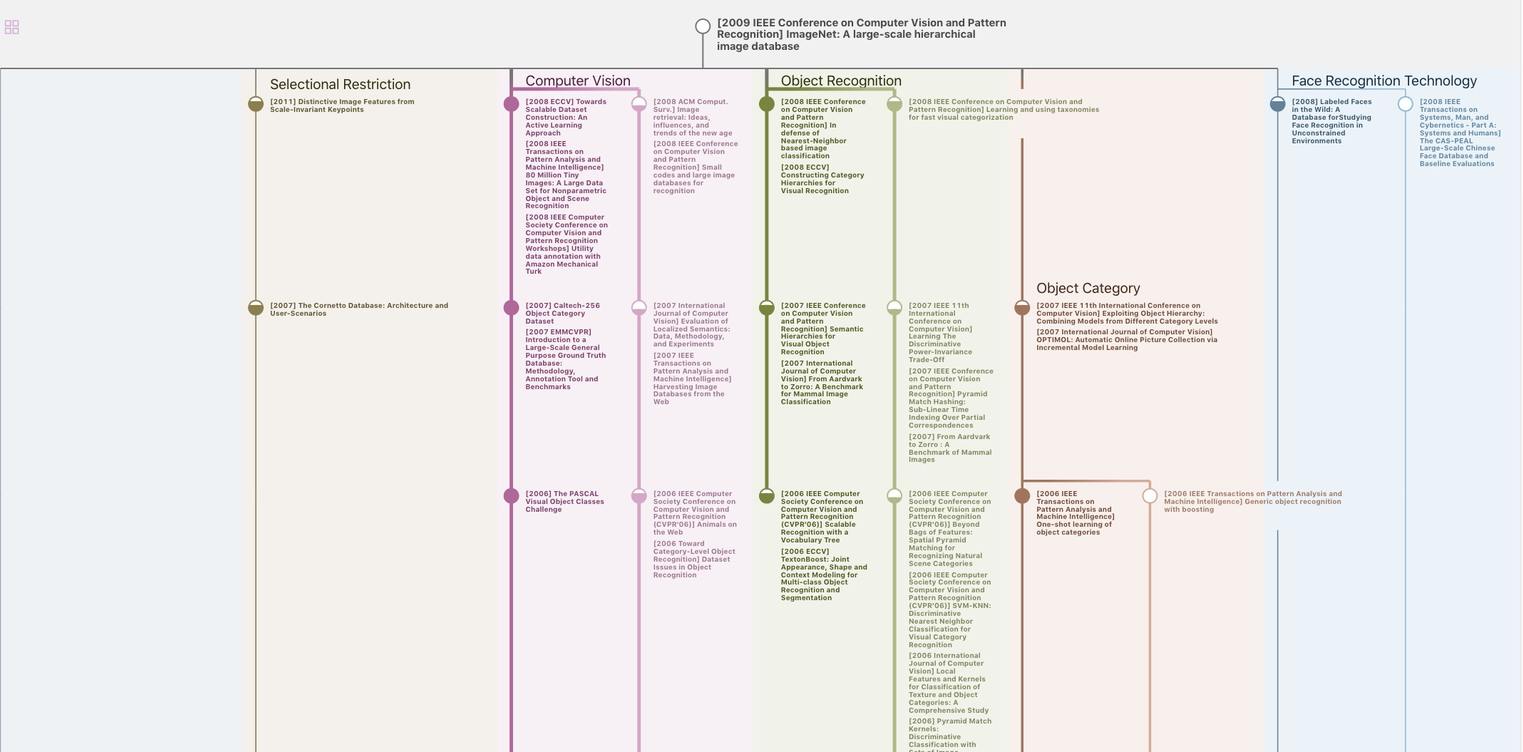
生成溯源树,研究论文发展脉络
Chat Paper
正在生成论文摘要