A Robustness-Assured White-Box Watermark in Neural Networks
IEEE TRANSACTIONS ON DEPENDABLE AND SECURE COMPUTING(2023)
摘要
Recently, stealing highly-valuable and large-scale deep neural network (DNN) models becomes pervasive. The stolen models may be re-commercialized, e.g., deployed in embedded devices, released in model markets, utilized in competitions, etc, which infringes the Intellectual Property (IP) of the original owner. Detecting IP infringement of the stolen models is quite challenging, even with the white-box access to them in the above scenarios, since they may have experienced fine-tuning, pruning, functionality-equivalent adjustment to destruct any embedded watermark. Furthermore, the adversaries may also attempt to extract the embedded watermark or forge a similar watermark to falsely claim ownership. In this article, we propose a novel DNN watermarking solution, named $HufuNet$HufuNet, to detect IP infringement of DNN models against the above mentioned attacks. Furthermore, HufuNet is the first one theoretically proved to guarantee robustness against fine-tuning attacks. We evaluate HufuNet rigorously on four benchmark datasets with five popular DNN models, including convolutional neural network (CNN) and recurrent neural network (RNN). The experiments and analysis demonstrate that HufuNet is highly robust against model fine-tuning/pruning, transfer learning, kernels cutoff/supplement, functionality-equivalent attacks and fraudulent ownership claims, thus highly promising to protect large-scale DNN models in the real world.
更多查看译文
关键词
Deep learning models,intellectual property,robustness,watermark
AI 理解论文
溯源树
样例
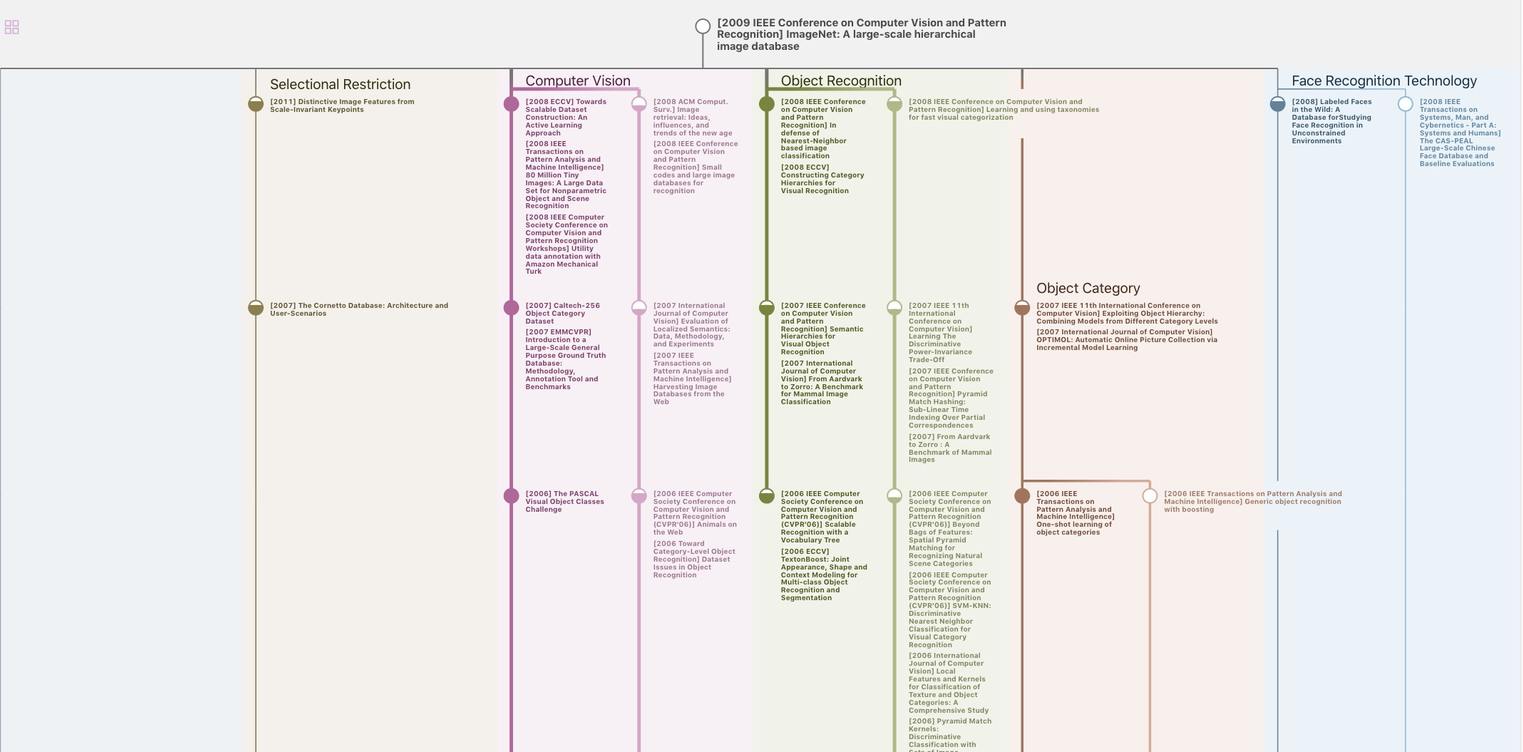
生成溯源树,研究论文发展脉络
Chat Paper
正在生成论文摘要