Classification Certainty Maximization for Unsupervised Domain Adaptation
IEEE Transactions on Circuits and Systems for Video Technology(2023)
摘要
The bi-classifier paradigm is a common practice in unsupervised domain adaptation (UDA), where two classifiers are leveraged to guide the model to learn domain invariant features. Previous approaches only focused on the consistency of the outputs between classifiers, but ignored the classification certainty of each classifier. Therefore, existing methods in some cases may mislead the classifiers into the wrong direction of ambiguous outputs and subsequently undermine the discriminability. To challenge this problem, in this paper we propose Classification Certainty Maximization (CCM) which considers both the joint certainty between classifiers and the individual certainty of each classifier via a novel formulation, and derived their optimal weight ratios by theoretical derivation from the perspective of gradient. In addition, we also propose a dynamic centroid update strategy to mitigate the domain gaps at the feature level. Extensive experiments on four widely used UDA datasets show that CCM performs better than the existing state-of-the-art domain adaptation methods. Notably, our dynamic centroid update strategy can be used as a plug-and-play module for existing bi-classifier domain adaptation methods to boost classification accuracy.
更多查看译文
关键词
unsupervised domain adaptation,domain adaptation,classification
AI 理解论文
溯源树
样例
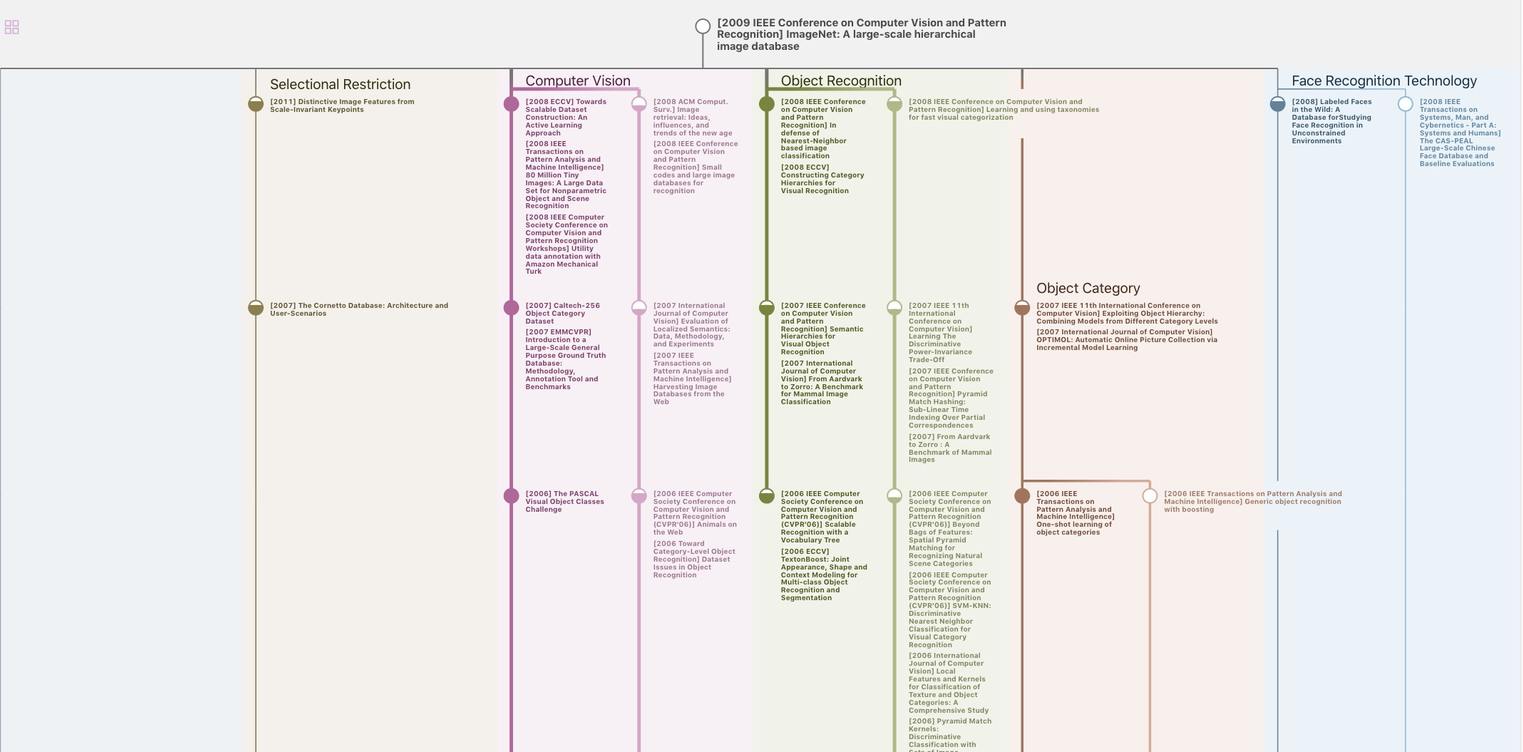
生成溯源树,研究论文发展脉络
Chat Paper
正在生成论文摘要