Prediction-Based EV-PV Coordination Strategy for Charging Stations Using Reinforcement Learning
2022 IEEE Transportation Electrification Conference and Expo, Asia-Pacific (ITEC Asia-Pacific)(2024)
Abstract
Coordinated charging activities of electric vehicles (EVs) in charging stations with distributed photovoltaic (PV) resources play an essential role in promoting PV consumption, saving electricity costs, and improving profits for charging stations. However, the uncertainties caused by PV power output and EV behaviors impose grand challenges to achieving the desired optimal control performance. This paper presents a novel real-time EV-PV coordination strategy using deep reinforcement learning (RL) algorithm for providing real-time controls based on short-term prediction of PV outputs and EV arrivals, which can effectively avoid large deviations between actual and optimal conditions. The EV-PV coordination problem is first described by EV station model and a Markov Decision Process (MDP). A charging control strategy inside the station is also proposed. Then, based on the soft actor-critic (SAC) algorithm, an RL training process for EV-PV coordination strategy is proposed. Though only short-term prediction is used in real-time control, the proposed strategy can balance short-term and long-term profit and achieve optimal charging control for EV stations. Comprehensive case studies with realistic EV charging station data verify the feasibility and effectiveness of the proposed method.
MoreTranslated text
Key words
Charging station,coordination strategy,electric vehicle,photovoltaic,reinforcement learning
AI Read Science
Must-Reading Tree
Example
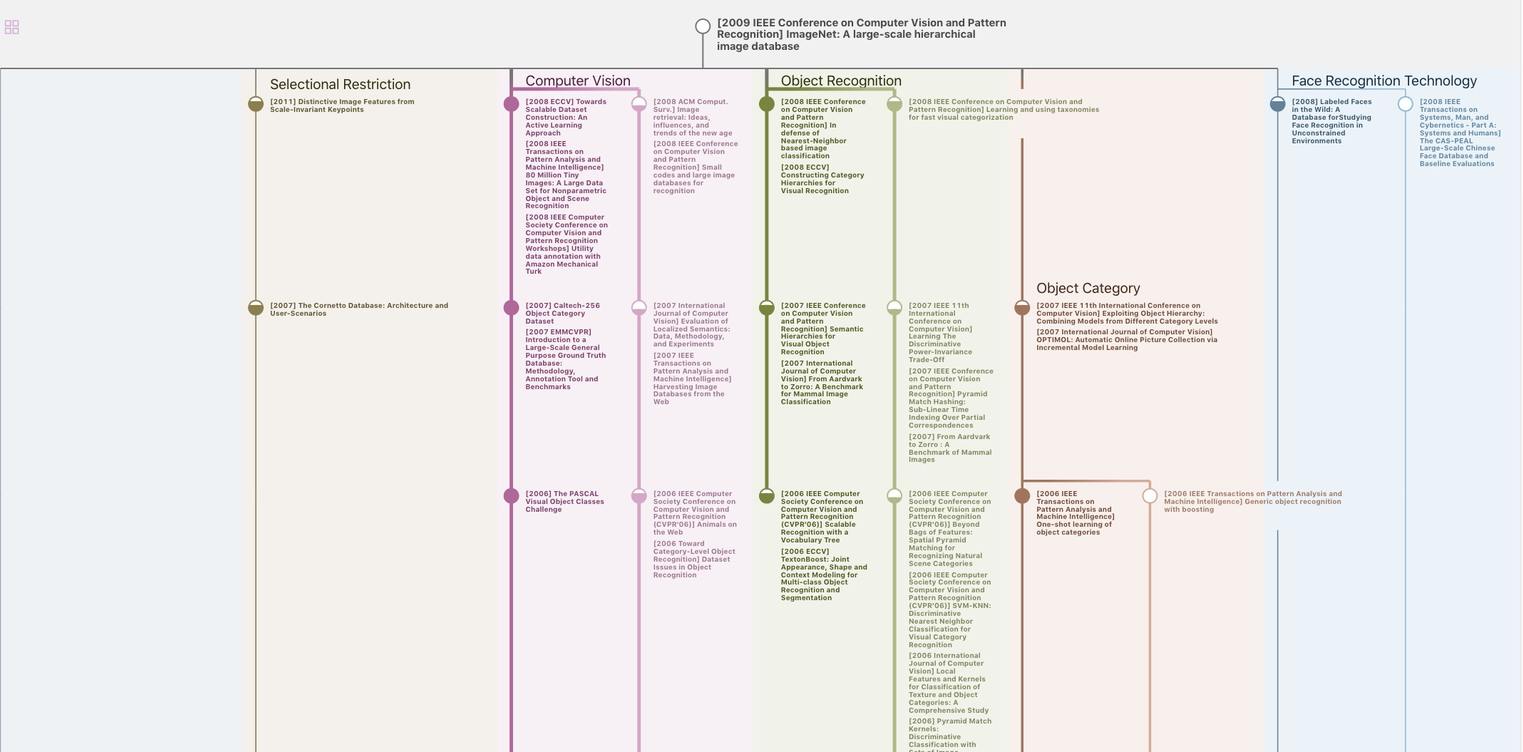
Generate MRT to find the research sequence of this paper
Chat Paper
Summary is being generated by the instructions you defined