Combined Use of Logarithmic Mean Divisia Index and Kaya Identity for Identifying the Relationship between Carbon Emission and Impacting Factors
2022 IEEE Global Conference on Computing, Power and Communication Technologies (GlobConPT)(2022)
Abstract
To achieve the goals of “carbon peaking in 2030” and “carbon neutrality in 2060”, as well as to optimize the carbon emission structure in Hangzhou city (China), this paper examines impacting factors in Hangzhou city from 2010 to 2019 including the changes of industrial structure, industrial GDP proportion, energy structure, and others. The Kaya identity and the logarithmic mean Divisia index (LMDI) decomposition method are first employed to investigate the relationship between each influencing factor and carbon emission for qualitatively analyzing the contribution of each impacting factor to the change of carbon emission. Then, the multiple-layer perception (MLP) neural network model with the well-known back-propagation (BP) algorithm is employed with the support of the genetic algorithm (GA-BP) to predict carbon emissions according to the changes of various impacting factors. Simulation results of numerical examples demonstrate that the GA-BP algorithm can well predict carbon emissions. Finally, by changing the proportion of clean energy in the secondary industry to change the city's carbon emissions, simulation results demonstrate that optimizing the industrial structure, especially reducing the proportion of the secondary industry, and increasing the proportion of clean energy can support Hangzhou to achieve the carbon peaking target in 2030.
MoreTranslated text
Key words
carbon emission,carbon peaking,carbon neutrality,logarithmic mean Divisia index (LMDI),decomposition method,genetic algorithm supported back-propagation (GA-BP)
AI Read Science
Must-Reading Tree
Example
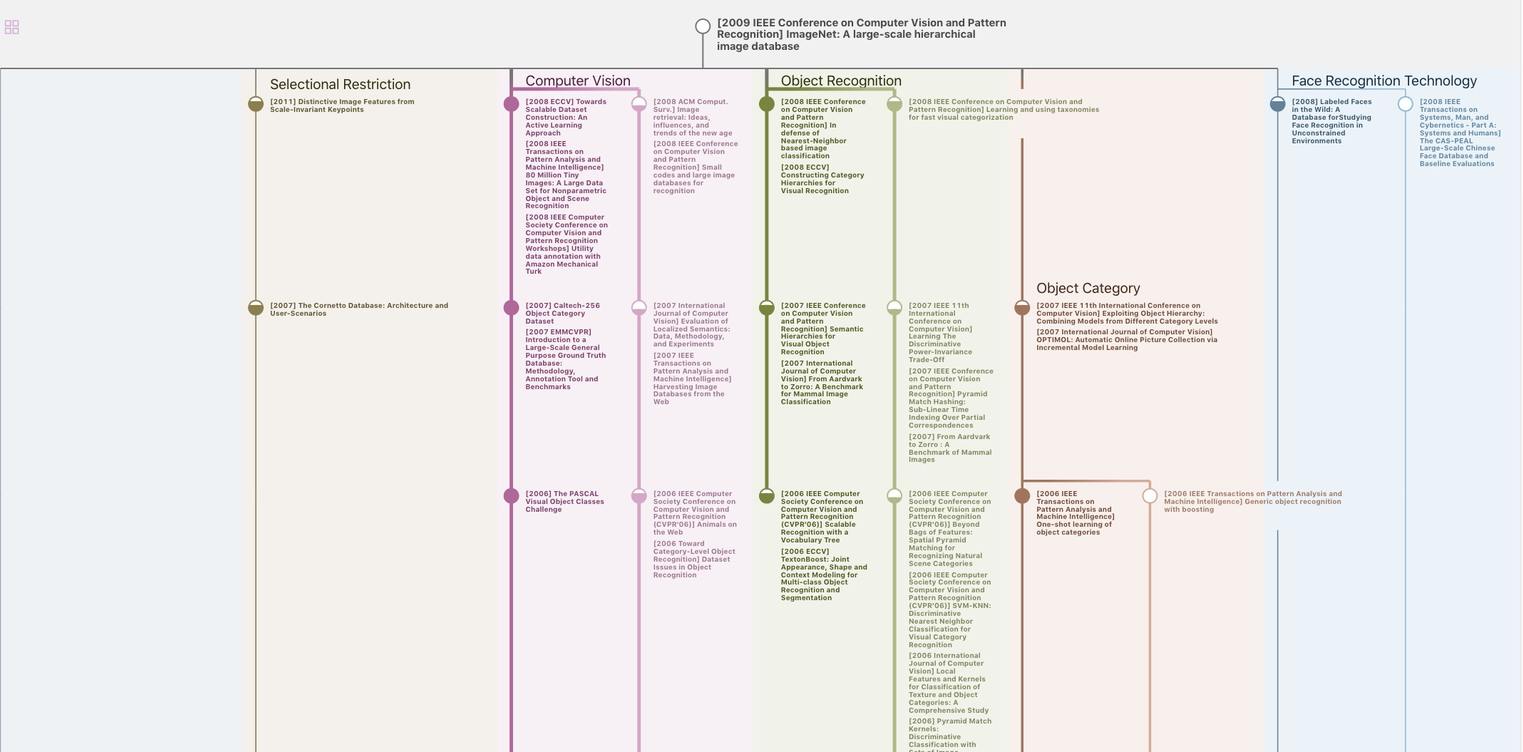
Generate MRT to find the research sequence of this paper
Chat Paper
Summary is being generated by the instructions you defined