Abnormal Identification of Oil monitoring Data Based on Classification-Driven SAE
2022 Global Reliability and Prognostics and Health Management (PHM-Yantai)(2022)
Abstract
In order to accurately understand the operating state of the equipment, monitor the abnormality of the oil state data in time, and effectively extract the abnormal data information in the oil monitoring data, this paper established a classification-driven SAE oil monitoring data abnormality recognition model. The nonlinear characteristics of the data predicted the state of the oil data. The label information is introduced into the collected oil monitoring data, and then the data is preprocessed. The deep features in the oil monitoring data are extracted by the stacked autoencoder (SAE). In the coding stage, the oil monitoring data training network with labels is used to realize the identification of abnormal data. The experimental results showed that: Compared with the Back Propagation Neural Network (BPNN) and the Support Vector Machine (SVM) classifier, the classification-driven stacked autoencoder had higher anomaly identification accuracy and could effectively detect abnormal data in oil monitoring data, so as to identified the abnormal monitoring of equipment status.
MoreTranslated text
Key words
oil monitoring data,abnormal identification,sae,classification-driven
AI Read Science
Must-Reading Tree
Example
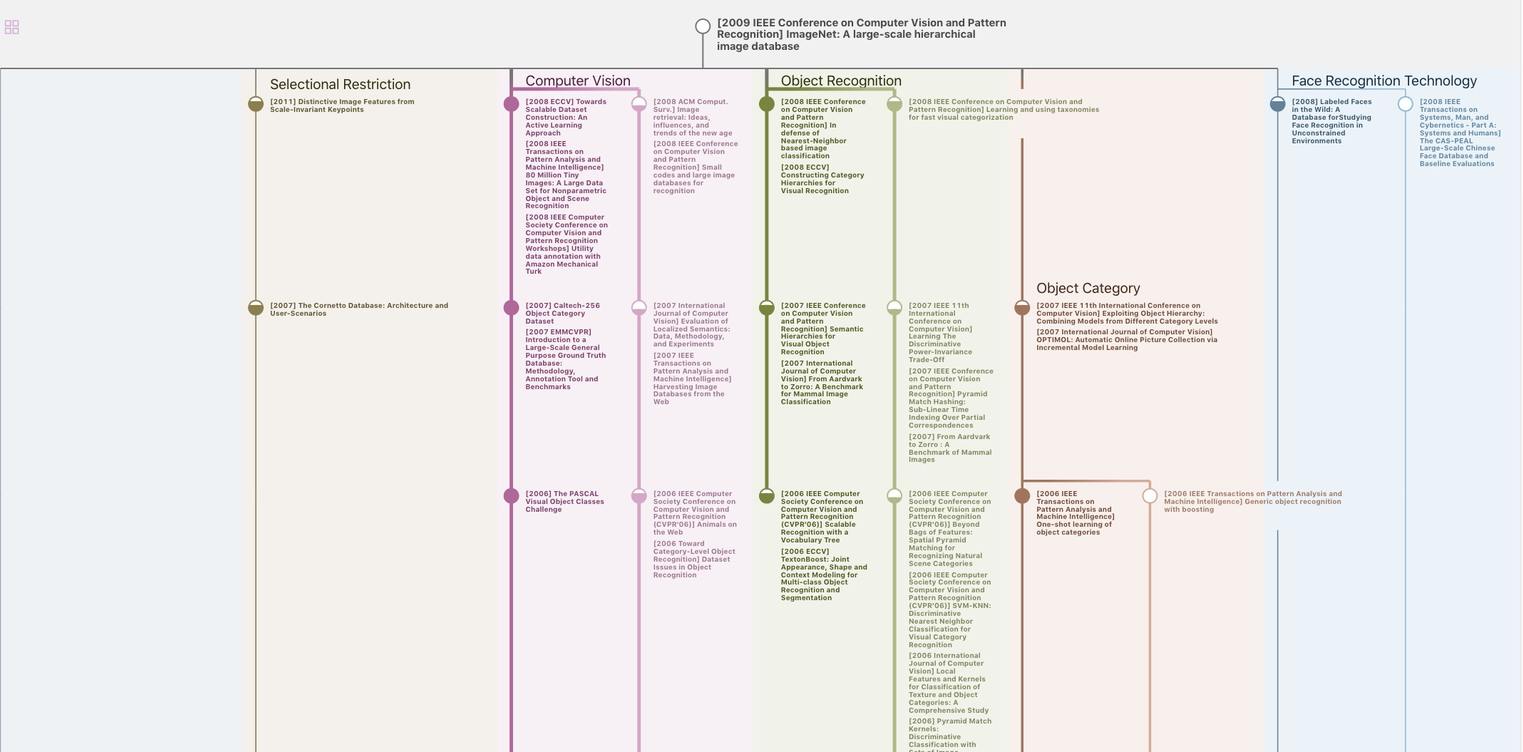
Generate MRT to find the research sequence of this paper
Chat Paper
Summary is being generated by the instructions you defined