Aspect-Guided Syntax Graph Learning for Explainable Recommendation
IEEE Transactions on Knowledge and Data Engineering(2023)
摘要
Explainable recommendation systems provide explanations for recommendation results to improve their transparency and persuasiveness. The existing explainable recommendation methods generate textual explanations without explicitly considering the user's preferences on different aspects of the item. In this paper, we propose a novel explanation generation framework, namely
A
spect-guided
E
xplanation generation with
S
yntax
G
raph (
AESG
), for explainable recommendation. Specifically, AESG employs a review-based syntax graph to provide a unified view of the user/item details. An aspect-guided graph pooling operator is proposed to extract the aspect-relevant information from the review-based syntax graphs to model the user's preferences on an item at the aspect level. Then, an aspect-guided explanation decoder is developed to generate aspects and aspect-relevant explanations based on the attention mechanism. The experimental results on three real datasets indicate that AESG outperforms state-of-the-art explanation generation methods in both single-aspect and multi-aspect explanation generation tasks, and also achieves comparable or even better preference prediction accuracy than strong baseline methods.
更多查看译文
关键词
syntax graph learning,explainable recommendation,aspect-guided
AI 理解论文
溯源树
样例
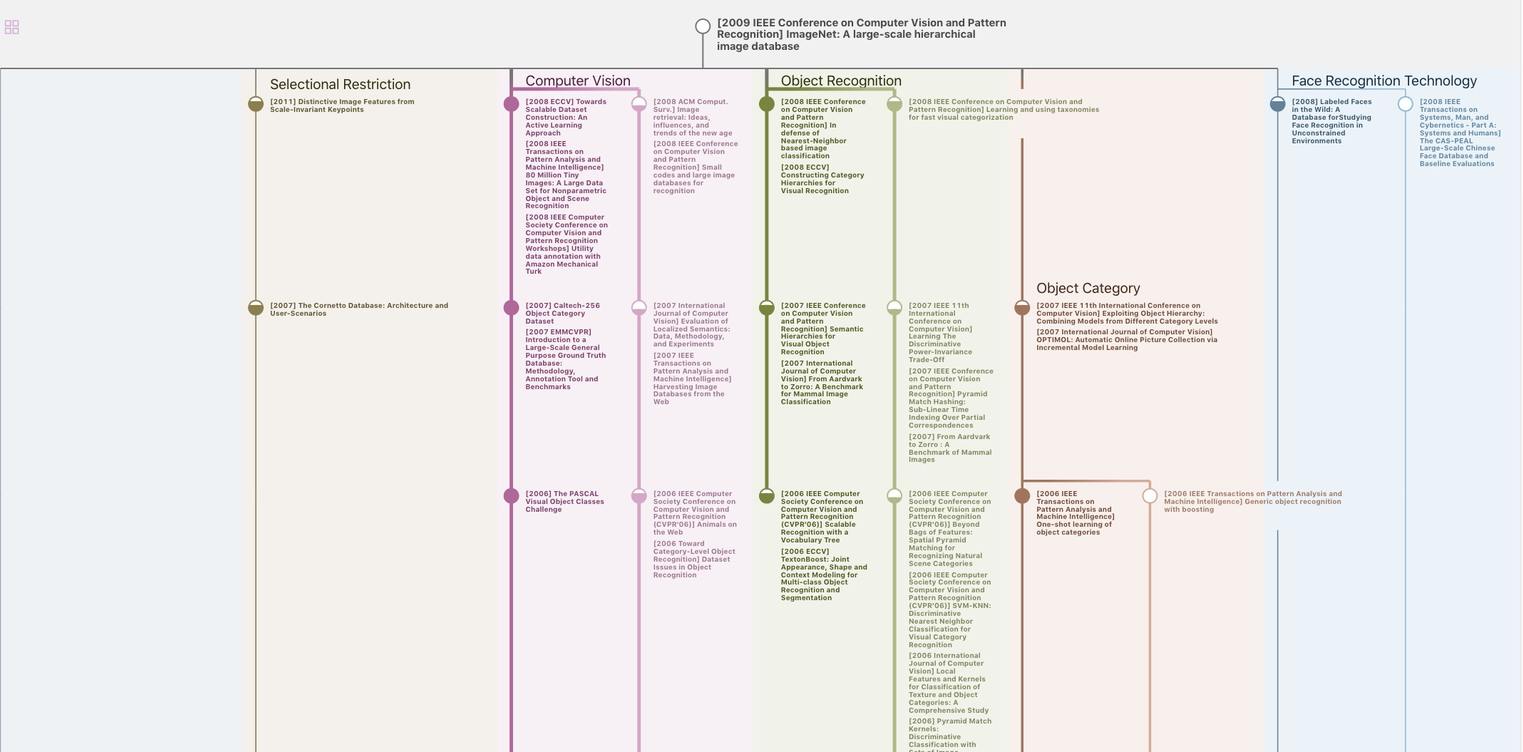
生成溯源树,研究论文发展脉络
Chat Paper
正在生成论文摘要