Denoising Method for Weak Velocity Test Signals Under Laser-Induced Shock Waves Based on Attention-Guided Multi-Scale Convolutional Neural Network
SSRN Electronic Journal(2023)
Abstract
In the research of characteristics of laser-induced shock waves via the laser interference velocity detection method, noise reduction for weak echo signals still relies on the manual setting of filtering parameters, alongside the problem of the loss of useful signals caused by global filtering and excessive filtering. To solve these prob-lems, a multi-scale network for extraction of sparse features, in combination with the ECA channel module, was established in this study to acquire the features of multi-dimensional and multi-fine-granularity noise signals in a self-adaptive manner. By integrating various channel information of the deep/shallow features through skip connection, the deep features were fully observed without ignoring the impacts of the shallow features. Finally, the attention mechanism was adopted to adjust the weight of the features at different locations in the feature space to acquire an accurate representation of stochastic noise, and noise reduction for weak signals was ach-ieved by reconstructing the module and removing the learned noise features from the noisy data. According to the simulation and test results, the method proposed in this study helped extract the noise information from weak signals in an accurate manner; unlimited by the forms and sizes of the noise and the working conditions and independent from manual setting of parameters, it eliminated the blind-source noise from weak echo signals effectively; with this method, the initial position and the coupled peak value were extracted accurately from the weak signals. In terms of the effects of the method, the phenomena of "peak clipping" and "breakpoint" caused by excessive filtering were avoided; compared to global filtering, time-frequency domain filtering and other methods, the method proposed outperformed the conventional ones for a peak value error of only 0.19%; as for the denoising velocity, simultaneous input of multiple pieces of data as well as simultaneous output of denoising outcomes in a real time manner were also achieved under this method, with the averaged processing time for a single piece of data (0.051 s) far superior to the result under other methods.
MoreTranslated text
AI Read Science
Must-Reading Tree
Example
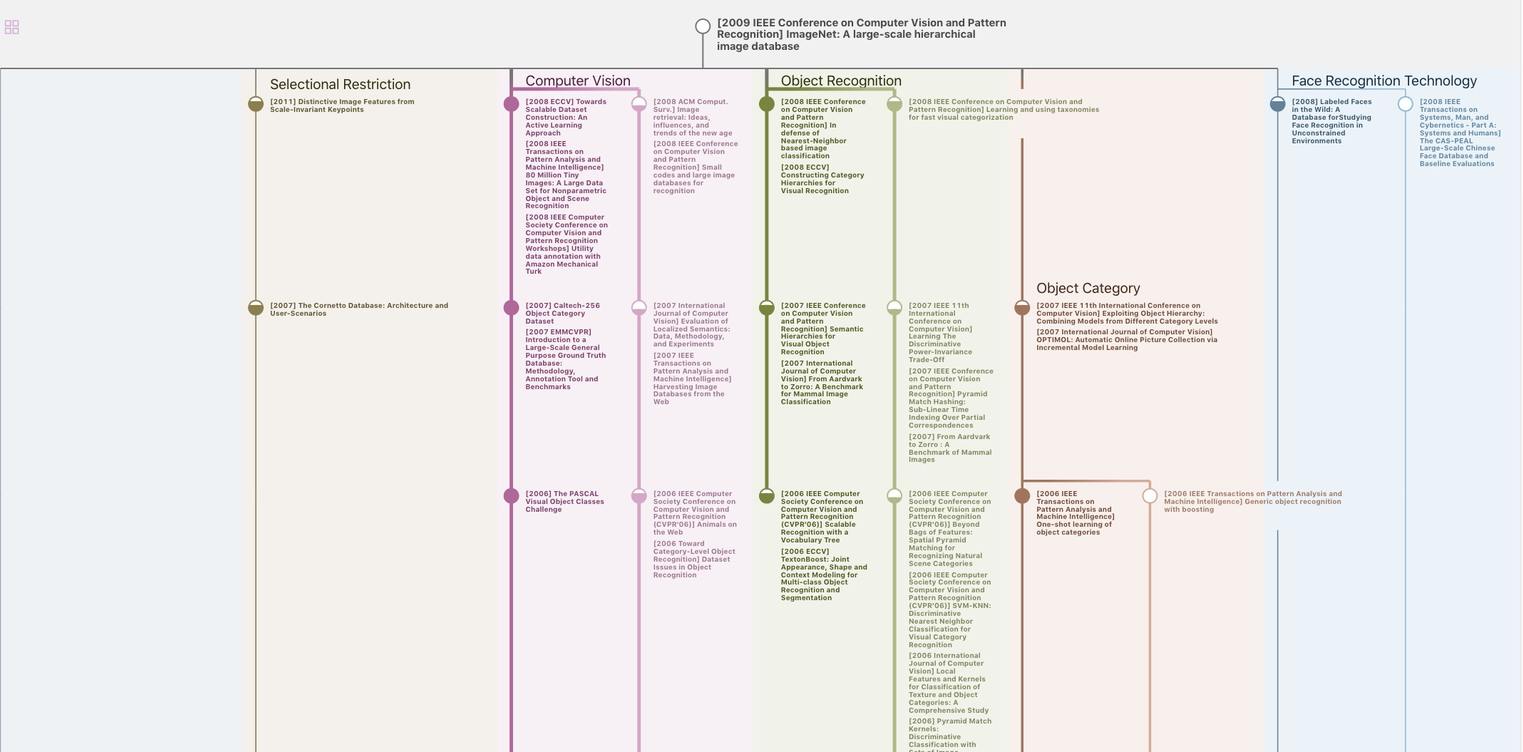
Generate MRT to find the research sequence of this paper
Chat Paper
Summary is being generated by the instructions you defined