Deep Learning Model for Prediction of Compound Activities Over a Panel of Major Toxicity-Related Proteins
Machine Learning and Deep Learning in Computational Toxicology Computational Methods in Engineering & the Sciences(2023)
Abstract
One of the main reasons for high attrition rate for drug candidates is their toxicity mediated through off-target binding. Thus, in vitro screening against major toxicity-related proteins is conventionally used to filter out problematic compounds at an early stage of drug development. However, in vitro testing is expensive and labour intense. Herein, we present a deep learning model that can effectively predict interaction between a small molecule of interest and a panel of 44 toxicity-associated proteins. Contrary to conventional toxicity prediction models (with one model per one endpoint), we employed a unified approach where a single model predicts activity over multiple targets. We demonstrate that this approach is superior, especially for datasets with unbalanced classes. The model is proposed for large-scale toxicity screening as well as for the elucidation of toxicity mechanisms of drug candidates.
MoreTranslated text
AI Read Science
Must-Reading Tree
Example
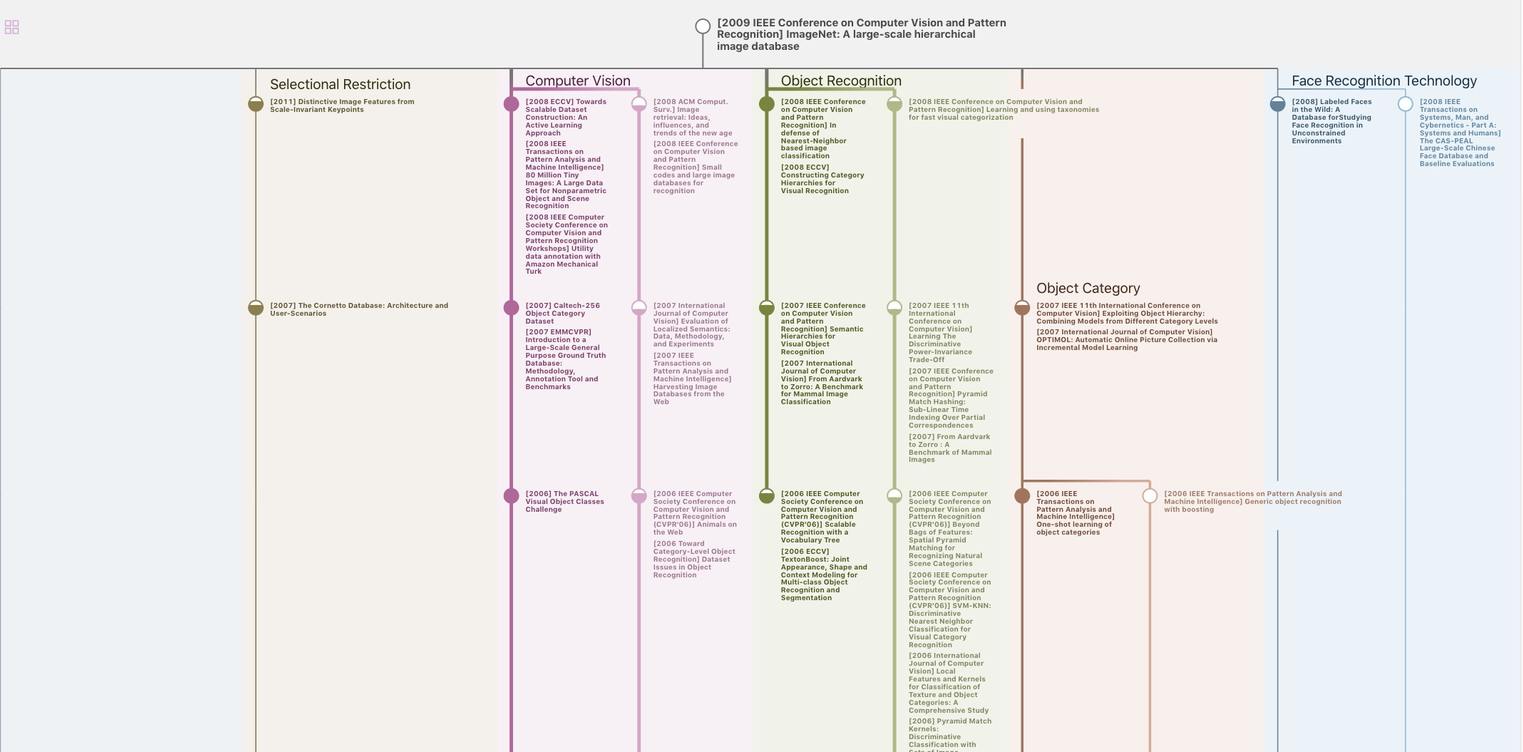
Generate MRT to find the research sequence of this paper
Chat Paper
Summary is being generated by the instructions you defined