Adversarial and Isotropic Gradient Augmentation for Image Retrieval With Text Feedback
IEEE TRANSACTIONS ON MULTIMEDIA(2023)
摘要
Image Retrieval with Text Feedback (IRTF) is an emerging research topic where the query consists of an image and a text expressing a requested attribute modification. The goal is to retrieve the target images similar to the query text modified query image. The existing methods usually adopt feature fusion of the query image and text to match the target image. However, they ignore two crucial issues: overfitting and low diversity of training data, which make the feature fusion based IRTF task not generalizable. Conventional generation based data augmentation is an effective way to alleviate overfitting and improve diversity, but increases the volume of training data and generation model parameters, which is bound to bring huge computation costs. By rethinking the conventional data augmentation mechanism, we propose a plug-and-play Gradient Augmentation (GA) based regularization approach. Specifically, GA contains two items: 1) To alleviate model overfitting on the training set, we deduce an explicit adversarial gradient augmentation from the perspective of adversarial training, which challenges the "no free lunch" philosophy. 2) To improve the diversity of training set, we propose an implicit isotropic gradient augmentation from the perspective of gradient descent-based optimization, which achieves the goal of big gain but no pain. Besides, we introduce deep metric learning to train the model and provide theoretical insights of GA on generalisation. Finally, we propose a new evaluation protocol called Weighted Harmonic Mean (WHM) to assess the model generalisation. Experiments show that our GA outperforms the state-of-the-art methods by 6.2 and 4.7% on CSS and Fashion200 k datasets, respectively, without bells and whistles.
更多查看译文
关键词
Gradient augmentation,image retrieval with text feedback (IRTF),model generalisation
AI 理解论文
溯源树
样例
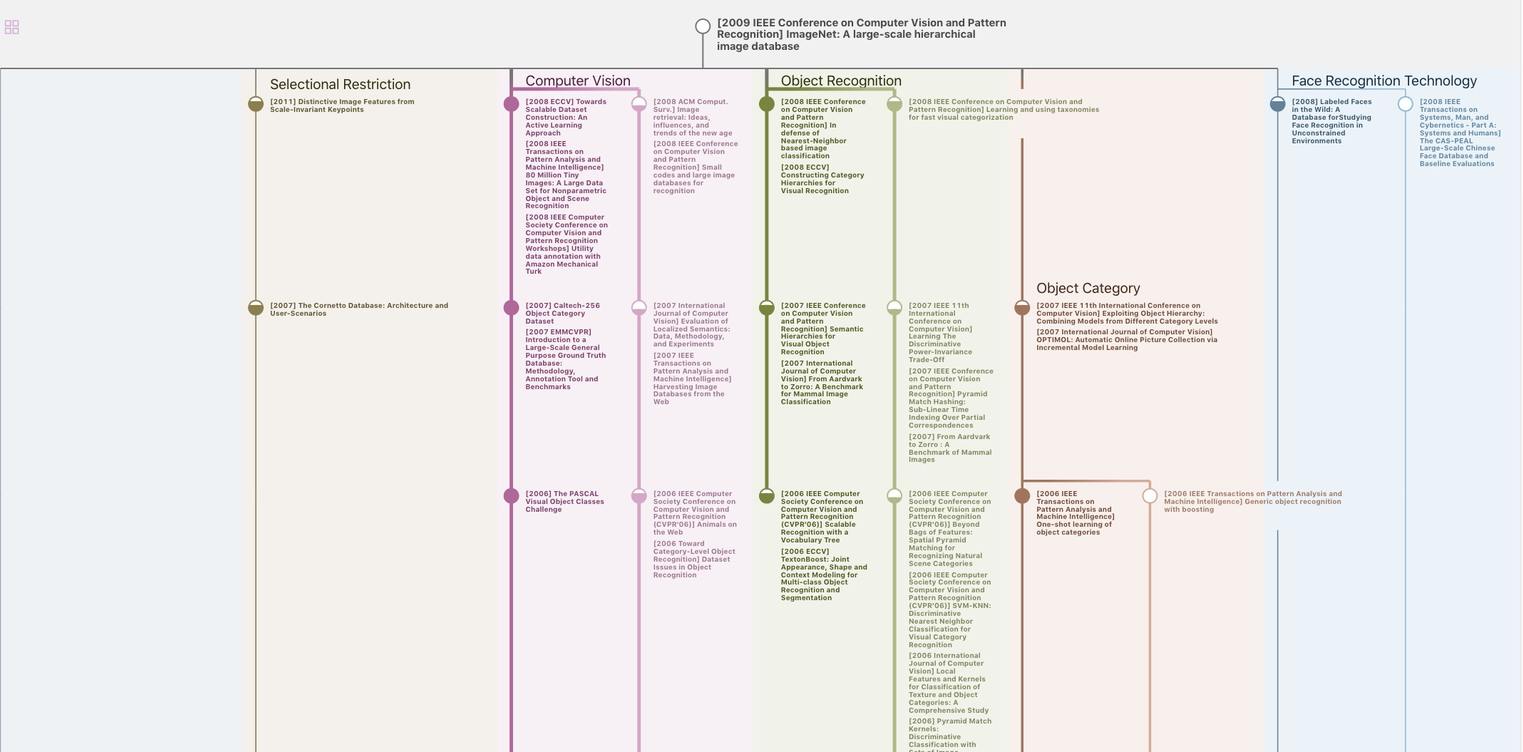
生成溯源树,研究论文发展脉络
Chat Paper
正在生成论文摘要