Multi-Fidelity Machine Learning Applied to Steady Fluid Flows
International Journal of Computational Fluid Dynamics(2022)
Abstract
A machine learning method to predict steady external fluid flows using elliptic input features is introduced. Using data from as few as one high-fidelity simulation, the proposed method produces models generalisable under changes to boundary geometry by using solutions to elliptic boundary value problems over the flow domain as the model input, instead of Cartesian coordinates of the domain. Training data is generated through pointwise evaluation of flow features at points selected through a quad-tree adaptive sampling method to concentrate training points in areas with large field gradients. Models are trained within a training window around the body, while predictions are smoothly extended to freestream conditions using a Partition-of-Unity extension. Predictive capabilities of the machine learning model are demonstrated in steady-state flow of incompressible fluid around a cylinder and a Joukowski airfoil. The predicted flow field is used to warm-start CFD simulations to achieve acceleration in solver convergence.
MoreTranslated text
Key words
machine learning,multi-fidelity
AI Read Science
Must-Reading Tree
Example
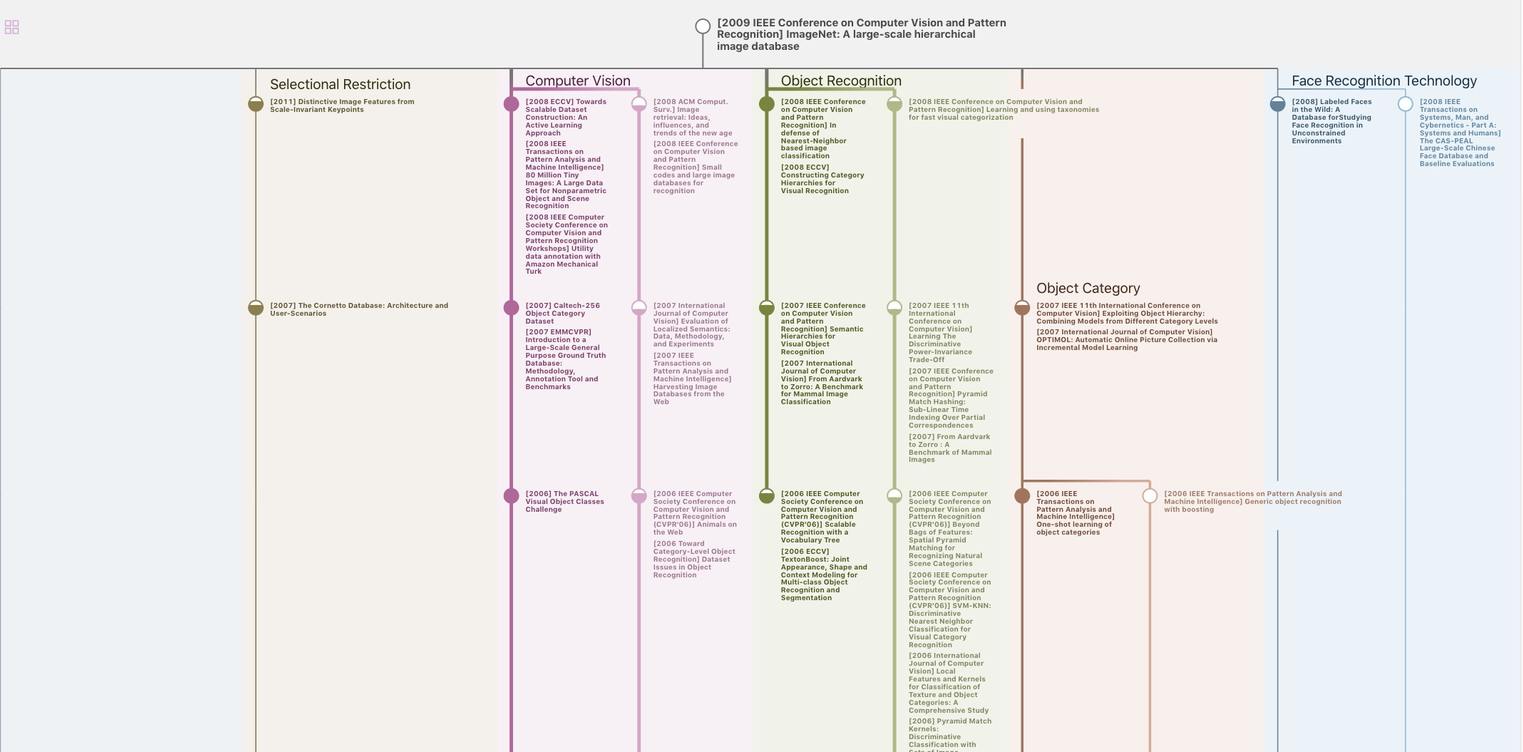
Generate MRT to find the research sequence of this paper
Chat Paper
Summary is being generated by the instructions you defined