Studies on Electricity Theft Detection Approach Based on LSTM-SAE and Support Vector Machine
2022 IEEE/IAS Industrial and Commercial Power System Asia (I&CPS Asia)(2022)
Abstract
The methods of electricity theft diversified and became more camouflaged against the backdrop of creating the smart grid in all respects. Existing detection methods struggled to meet the application's requirements. As a result, using long and short term memory stacked auto encoder (LSTM-SAE) and support vector machine(SVM), this research developed an electricity-stealing identification model. For high-dimensional nonlinear time series, LSTM-SAE has good feature extraction capabilities, making it suitable for mining the deep properties of electricity consumption data. SVM is applied as classifiers, whose parameters are optimized by particle swarm optimization(PSO) algorithm. Features extracted by LSTM-SAE to output the results through SVM classifier. Through the experimental simulation of actual power grid data, false positive rate(FPR) and detection rate(DR) demonstrate the effectiveness and accuracy of the proposed method.
MoreTranslated text
Key words
electricity theft detection approach,support vector machine,vector machine,lstm-sae
AI Read Science
Must-Reading Tree
Example
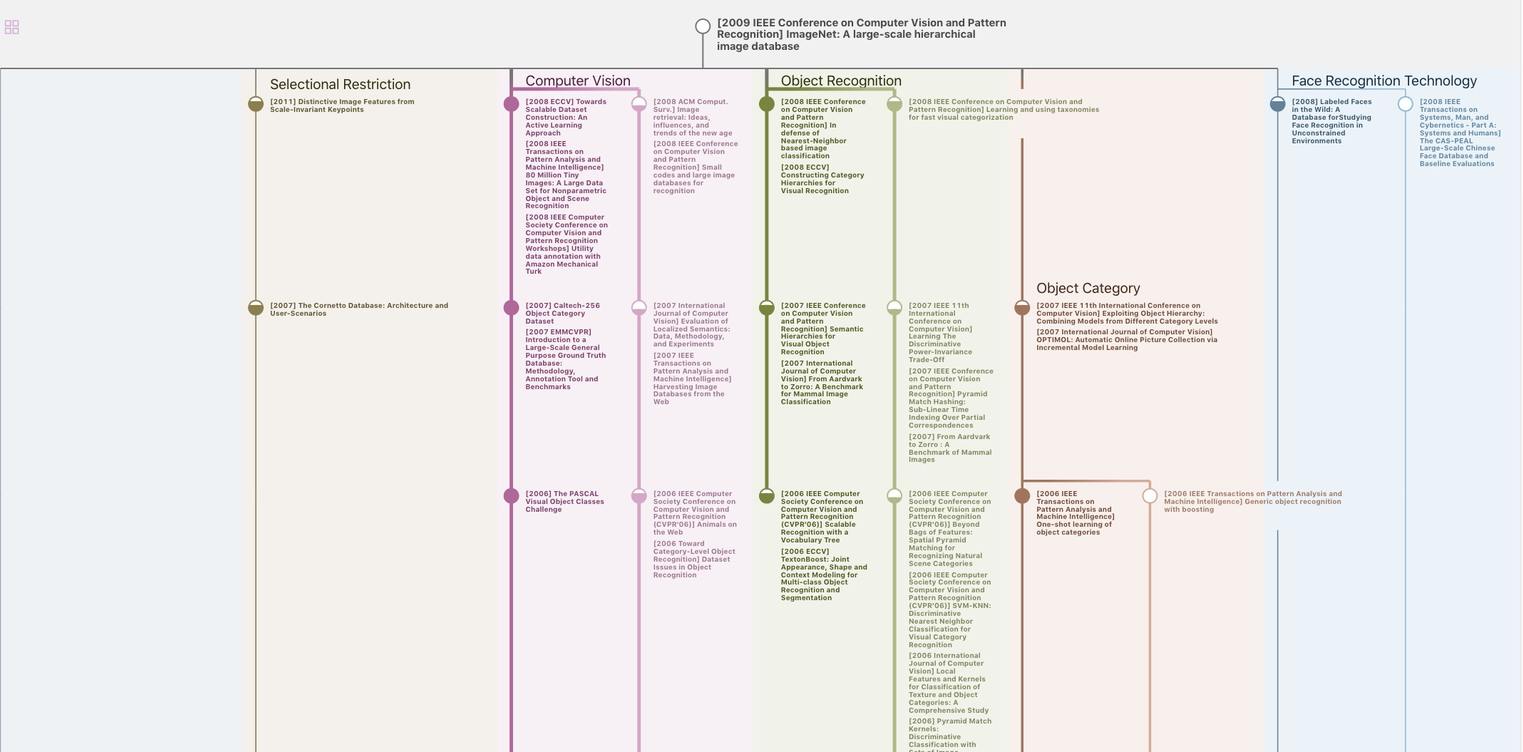
Generate MRT to find the research sequence of this paper
Chat Paper
Summary is being generated by the instructions you defined