Learning in Networks: An Experiment on Large Networks with Real-World Features
Management Science(2023)
摘要
Subjects observe a private signal and make an initial guess; they then observe their neighbors’ guesses, update their own guess, and so forth. We study learning dynamics in three large-scale networks capturing features of real-world social networks: Erdös–Rényi, Stochastic Block (reflecting network homophily), and Royal Family (that accommodates both highly connected celebrities and local interactions). We find that the Royal Family network is more likely to sustain incorrect consensus and that the Stochastic Block network is more likely to persist with diverse beliefs. These patterns are consistent with the predictions of DeGroot updating. It lends support to the notion that the use of simple heuristics in information aggregation is prevalent in large and complex networks. This paper was accepted by Yan Chen, behavioral economics and decision analysis. Funding: The authors thank the Keynes Fund (University of Cambridge), the Creative-Pioneering Researchers Program (Seoul National University), and C-BID (NYUAD) for financial support. Supplemental Material: The data files and e-companion are available at https://doi.org/10.1287/mnsc.2023.4680 .
更多查看译文
关键词
large networks,learning,features,real-world
AI 理解论文
溯源树
样例
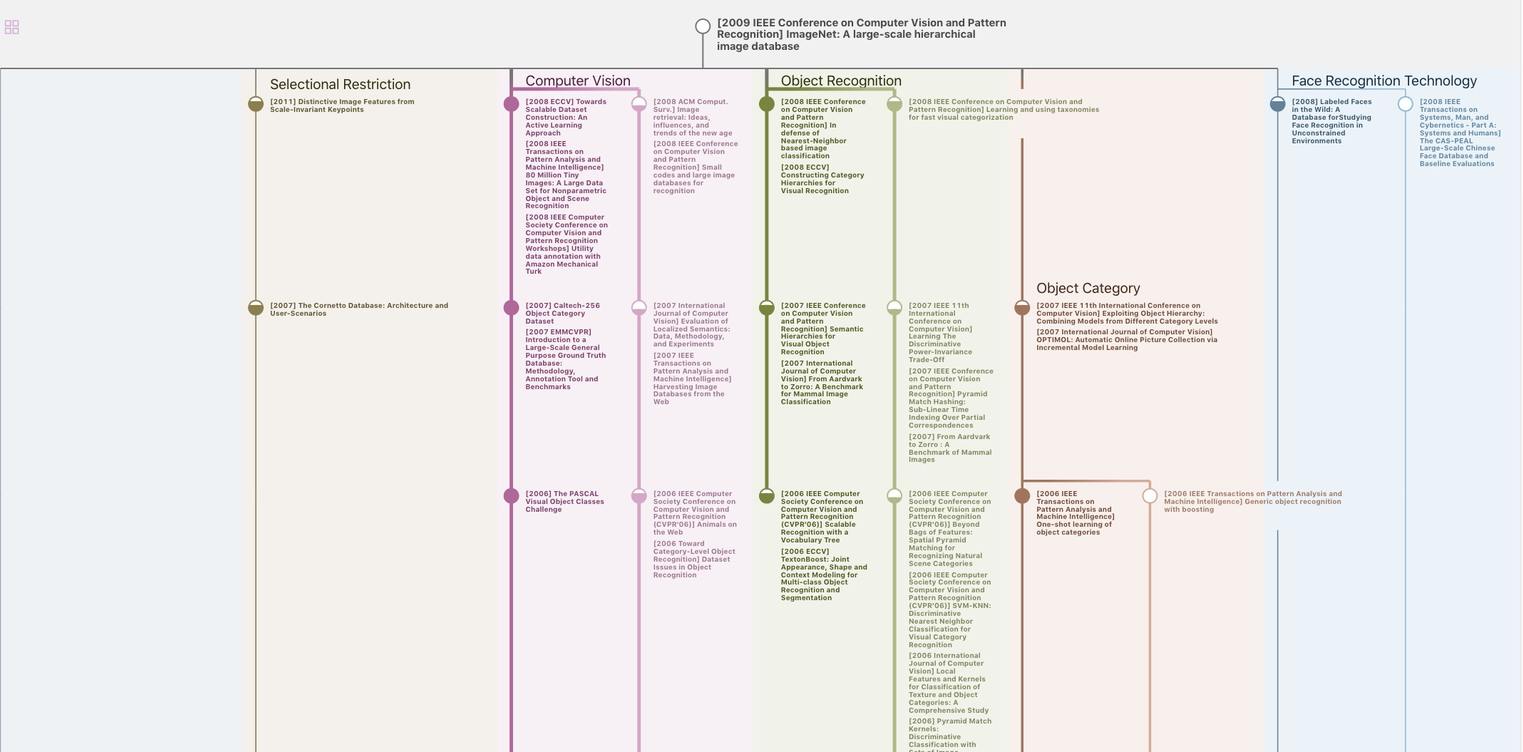
生成溯源树,研究论文发展脉络
Chat Paper
正在生成论文摘要