A Lightweight Detection Scheme for the Safety of Human Behaviors in Power Construction Sites
JOURNAL OF CIRCUITS SYSTEMS AND COMPUTERS(2023)
Abstract
Safety mishaps at electric power construction sites have been more frequent in recent years as a result of the growth of the electric power business, the inescapable carelessness of current safety oversight, and other causes. Traditional manual supervision and video surveillance methods demand a lot of human resources and suffer from issues like low efficiency and lack of objectivity, making it unable to provide security alerts in real-time and accurately. Therefore, solutions like intelligent monitoring based on object detection are required to address this issue. Many object detection methods now in use (such as R-CNN, Fast R-CNN, and YOLOV5) are computationally and energy intensive. They are typically installed on remote back-end servers, which makes it impossible to guarantee the real-time security alert. This paper trains a lightweight NanoDet network model to recognize the attire and actions of power construction workers in order to address the aforementioned issues. Additionally, in order to meet the real-time security warning requirements and fully utilize the limited computing resources of edge devices, the NanoDet network model is divided, with some layers being divided into CPU execution. According to experiments, power consumption can be reduced by deploying the Backbone or PAFPN blocks of the NanoDet model to CPUs and running them in 30W4Core mode.
MoreTranslated text
Key words
Object detection,network segmentation,power construction safety
AI Read Science
Must-Reading Tree
Example
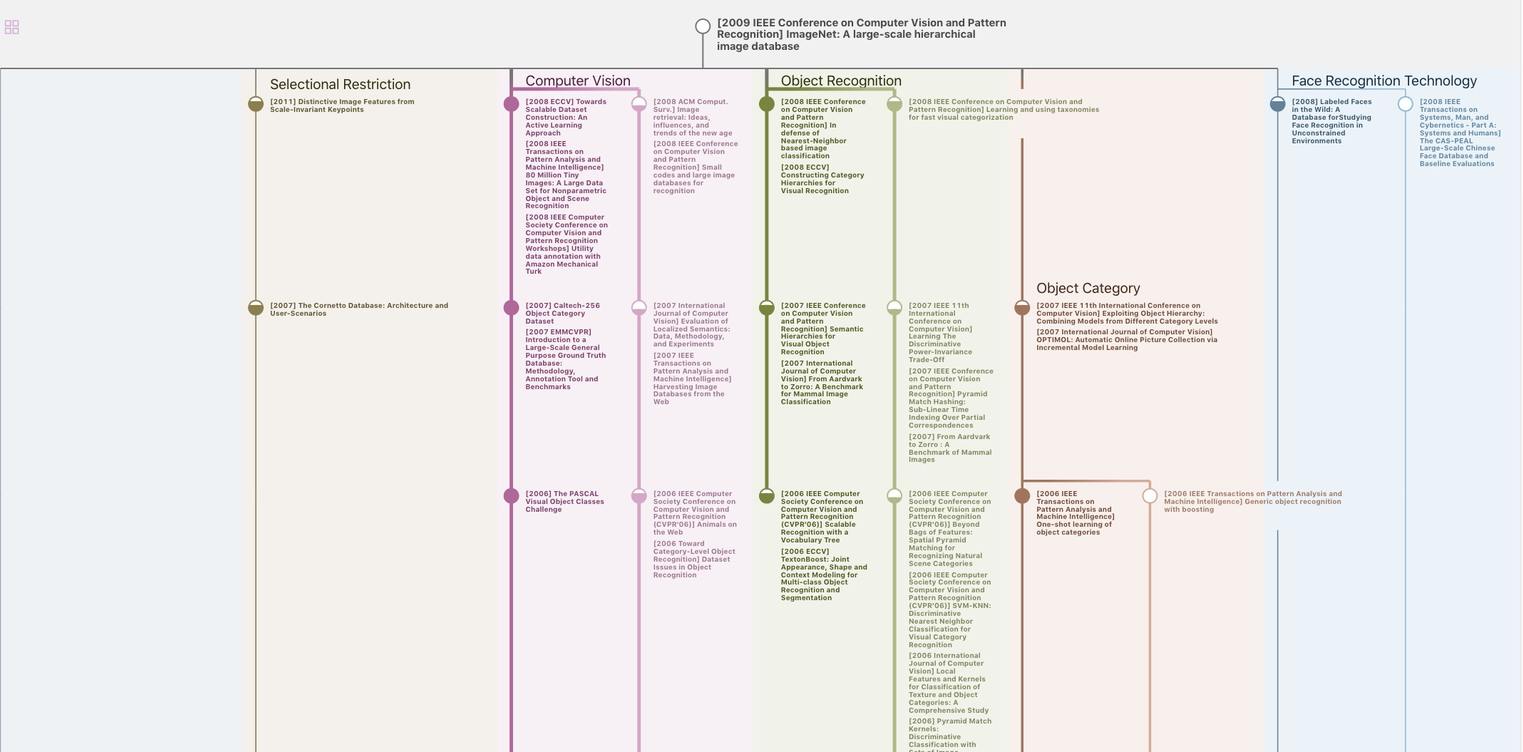
Generate MRT to find the research sequence of this paper
Chat Paper
Summary is being generated by the instructions you defined