Load Probability Prediction Method Considering Parameter Optimization of Improved Extreme Learning Machine
2022 IEEE/IAS Industrial and Commercial Power System Asia (I&CPS Asia)(2022)
Abstract
With the development of technologies such as electric vehicles and the increase in the penetration rate of distributed power sources, the randomness and uncertainty of grid loads are gradually increasing. Using effective methods to accurately predict power load can improve the efficiency of power system planning. Hence, this paper proposes a load probability prediction method considering the parameter optimization of improved extreme learning machine (ELM). Firstly, the historical power load data set is collected and preprocessing such as correlation analysis and data elimination is performed. The processed historical data is used as the input dataset for the ELM model. Secondly, the genetic algorithm (GA) is used to optimize and improve the input weight and bias of the ELM model. The quantile regression optimization is used to improve the output weight of the ELM model. According to the calculation result of individual fitness as the termination condition of GA iteration, the improved ELM model parameters are generated, and the load power prediction interval is calculated according to the optimized model. Finally, the effectiveness of the method proposed in this paper is verified by case studies calculation based on the historical load power data.
MoreTranslated text
Key words
load probability forecasting, improved extreme learning machine, genetic algorithm, quantile regression
AI Read Science
Must-Reading Tree
Example
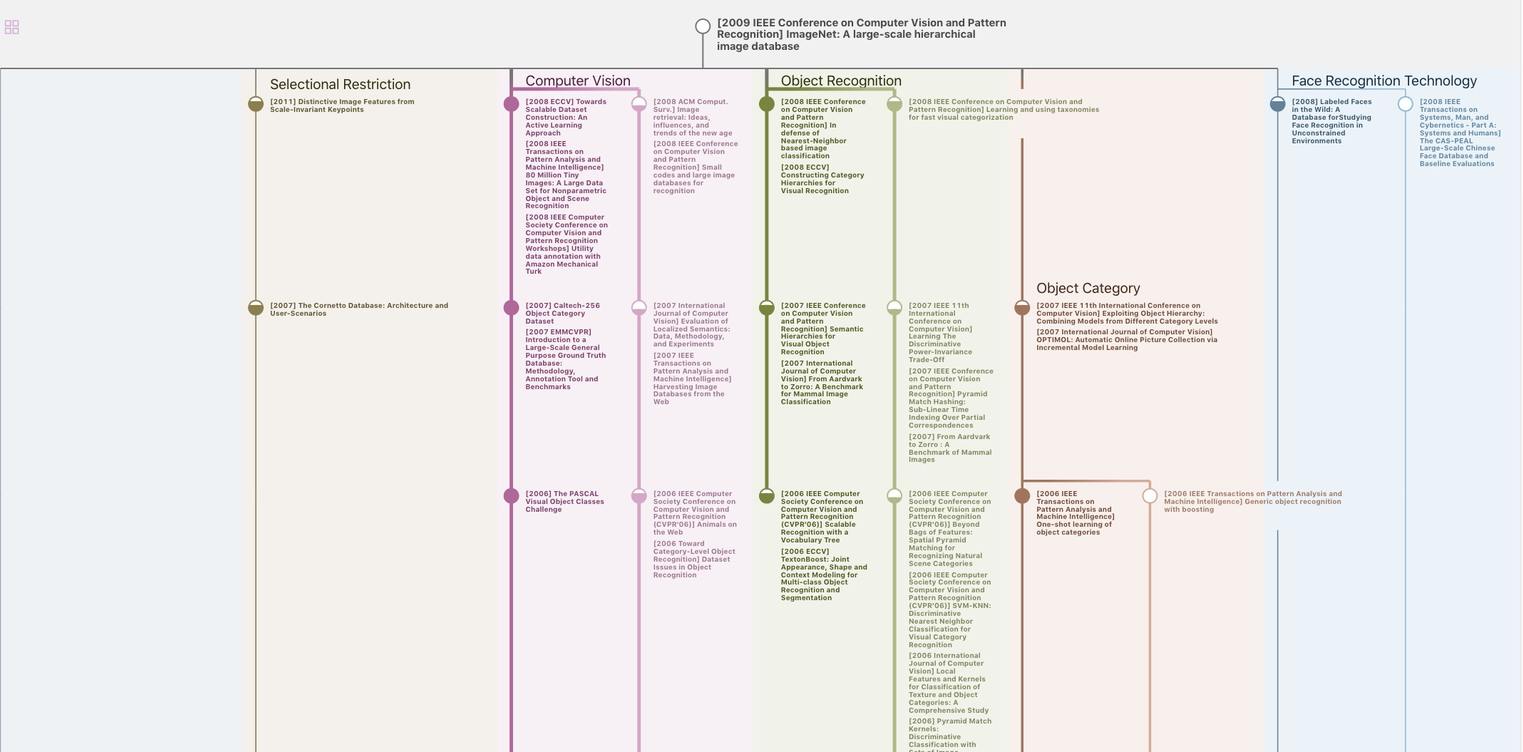
Generate MRT to find the research sequence of this paper
Chat Paper
Summary is being generated by the instructions you defined