Multi-factor ranking method for trading-off accuracy, diversity, novelty, and coverage of recommender systems
International Journal of Information Technology(2023)
摘要
Collaborative filtering (CF) is one of the most popular and commonly used recommendation methods. Currently, most rating prediction CF methods select top-N recommendations based on their predicted rating. Thus, CF achieved a remarkable prediction accuracy, but it has shown modest performance in terms of novelty, diversity, and coverage. This research study presents a new efficient ranking method for CF, namely, multi-factor ranking (MF-R). The proposed method adopts two factors to rank items: the predicted rating and popularity of items. MF-R aims to select recommendations achieving accuracy, novelty, diversity, and coverage objectives. A set of experiments are conducted to compare MF-R with the traditional ranking method. Three benchmark datasets, MovieLens-Latest, MovieLens-100 K, and HotelExpedia, are utilized. Both ranking methods are integrated with different single-criterion and multi-criteria CF techniques. On average, MF-R achieved 26%, 496%, 39%, and 0.9% improvements in terms of precision, novelty, coverage, and diversity, respectively. The results demonstrate the MF-R capability to achieve the four objectives of RS irrespective of the recommendation size. Besides, the results show that MF-R degrades the effect of the long-tail challenge.
更多查看译文
关键词
Recommender system,Top-N ranking,Accuracy,Diversity,Novelty,Coverage
AI 理解论文
溯源树
样例
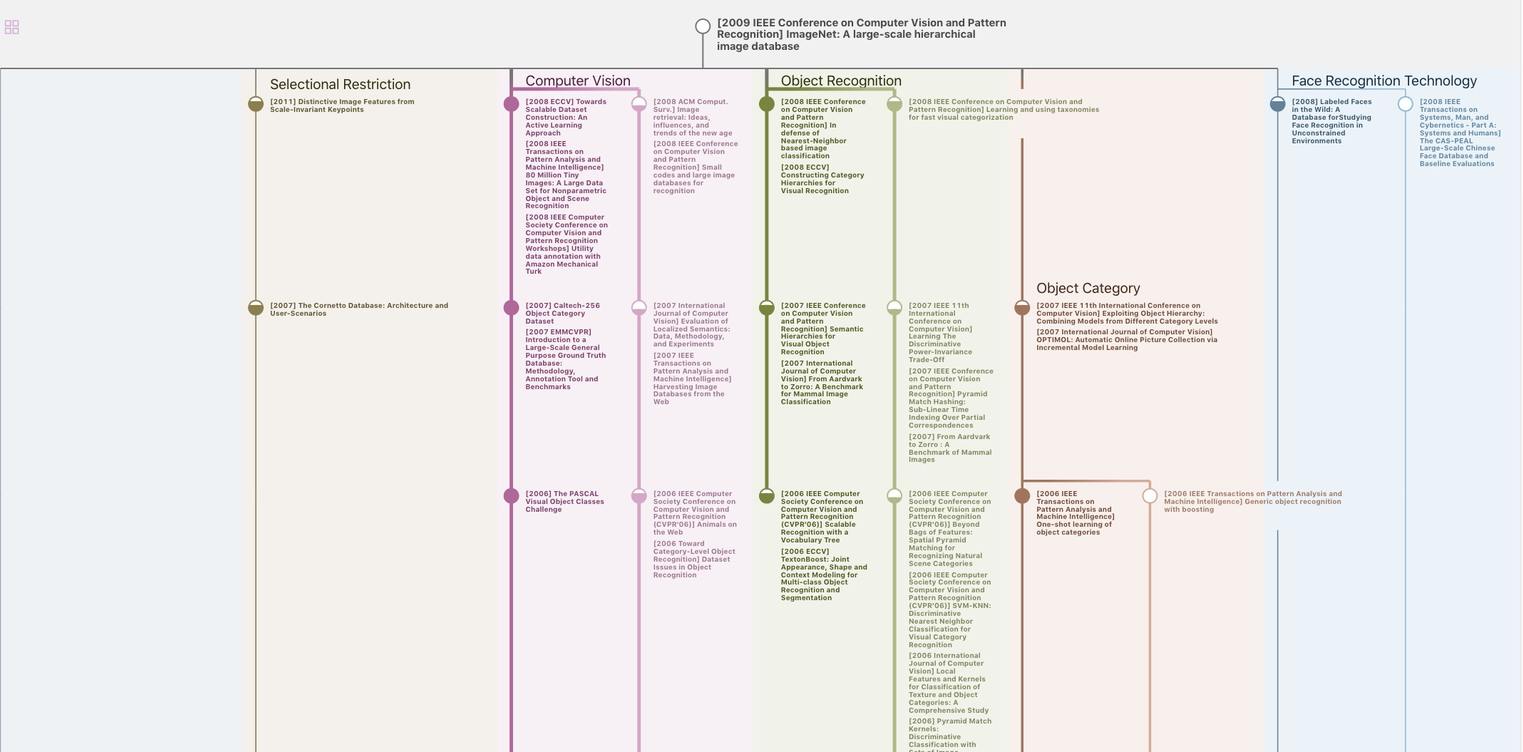
生成溯源树,研究论文发展脉络
Chat Paper
正在生成论文摘要