Digital soil mapping of Italy to map derived soil profiles with neural networks
Geoderma Regional(2023)
摘要
In recent years there has been an increased demand for digital soil mapping (DSM) products. DSM has become the ultimate soil spatial representation framework due to its quantitative results, replicability, and uncertainty analysis. The present study aimed to map the probability distribution of the derived soil profiles (DSPs) of soil typological units (STUs). DSPs are statistical representation of the properties of the soil profiles belonging to STUs. STUs aggregate individual profiles into a group. The criteria used for grouping were homogeneity for World Reference Base (WRB) reference soil group (WRB-RSG), qualifiers (WRB-qu), and Soil Taxonomy particle size for the family classification (USDA-PS), and the belonging to a specific Soil Region. The European Soil Regions have been suggested as the primary grouping criteria for soil mapping at the European continental scale since they define continental-scale soilscapes, distinguished mainly by their climate and geology. To map DSPs, we firstly mapped STUs. The grouping criteria of STUs were mapped at 500 m spatial resolution, using a Neural Network trained on 18,707 georeferenced and analyzed soil profiles selected from the Italian national soil database. A 10% of the soil profiles were randomly sampled using a stratified sampling approach for validation. In particular, the procedure consisted of: i) mapping the grouping criteria WRB-RSG, WRB-qu, and USDA-PS, on a 500 m national grid, through Neural Network; ii) grouping soil profiles on the base of the combinations of grouping criteria (WRB-RSG, WRB-qu, USDA-PS, and Soil Regions) as mapped with the first step at each grid node, to produce a map of Soil Typological Units (STUs); iii) calculating statistics for the soil parameters of the groups of soil profiles created, to produce a map of Derived Soil Profiles (DSPs). DSPs statistics (average, standard deviation, and sample numerosity) were elaborated for the following parameters: soil rooting depth, pH (in water), soil organic carbon, clay, silt, sand, coarse fragments, and cation exchange capacity. The maps obtained were validated against the test set. The same test set was used for the comparison with the National benchmark map (Soils Map of Italy 1:1,000,000) and with the global scale SoilGrids at 250 m spatial resolution. The overall accuracy was 45.98% for the WRB-RSG map compared with the 30.74% of WRB-RSG as mapped with the Soil Map of Italy, and 28.79% as mapped with SoilGrids; 33.07% for WRB-qu compared with the 15.69% of WRB-qu as mapped with the Soil Map of Italy, and 12.45% as mapped with SoilGrids, and 45.48% for USDA-PS, not comparable with the National and Global benchmarks. Tau statistics showed a higher accuracy Kappa of our approach than in others, due to the unbalanced classes numerosity. The predictive ability in the validation of DSPs parameters resulted in a R2 of 0.35 for clay (0.16 with SoilGrids), 0.28 for sand (0.08 with SoilGrids), 0.18 for pH in water (0.21 with SoilGrids). The proposed approach produced harmonized soil type maps with higher accuracy than the previous generation of conventional field-based soil maps for the national benchmark and the calculation of the uncertainty. The STUs express variability of soil properties between groups so their knowledge might improve our understanding of the soil distribution, the planning of their management, monitoring, and the decisions for further surveys. A future challenge will be including more dynamic parameters in the criteria used to create STU, to help monitoring soil management effects.
更多查看译文
关键词
digital soil mapping,soil profiles
AI 理解论文
溯源树
样例
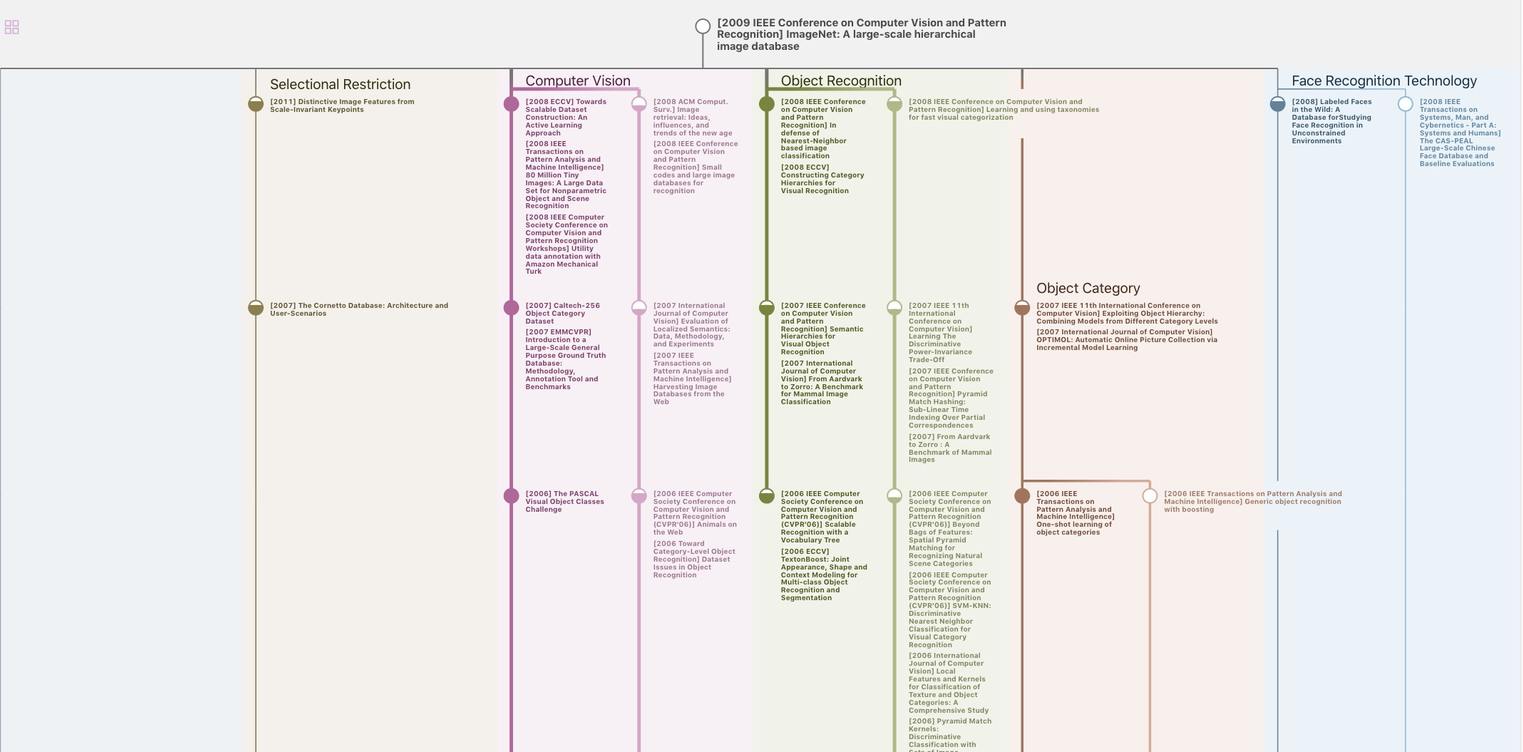
生成溯源树,研究论文发展脉络
Chat Paper
正在生成论文摘要