Classification of Vegetation Types in the Mountainous Terrain Using Random Forest Machine Learning Technique
Springer Climate(2023)
摘要
Classification of vegetation into appropriate classes is important for management and conservation planning. Field-based observations are now extensively supported with remote sensing-based observations for such classifications. We demonstrate here the application of a machine learning technique using the random forest (RF) to classify Landsat imageries in the mountainous terrain of the Indian Western Himalayas. The region represents a mega-diverse area having a wide variation in climate and vegetation types with a varied topography. In mountainous regions, vegetation classification is crucial to identify the natural resources for its conservation and management planning. Normalized difference vegetation index (NDVI) using near infra-red and red bands was created for the period 2013-2019. As the imageries are available at a temporal resolution of 16 days, a Fourier transformation was done to compress a large amount of data. To achieve a better accuracy of classification, topographic variables of elevation and slope together with climate variables of temperature and precipitation were considered while implementing the classification algorithm. We successfully characterized the mountainous terrain into the classes of non-forest, evergreen needle leaf trees, evergreen broadleaf trees, moist deciduous trees, dry deciduous trees, shrub, and agriculture with an overall accuracy of 80%. We compared the classified maps with existing vegetation type maps to see inconsistency in mapping with the demonstrated approach. The methodology demonstrated in this study can be used for classifying the landscape into distinct classes with improved accuracy for various purposes.
更多查看译文
关键词
Forest type,Indian Western Himalaya,Land use/land cover,NDVI,Remote sensing
AI 理解论文
溯源树
样例
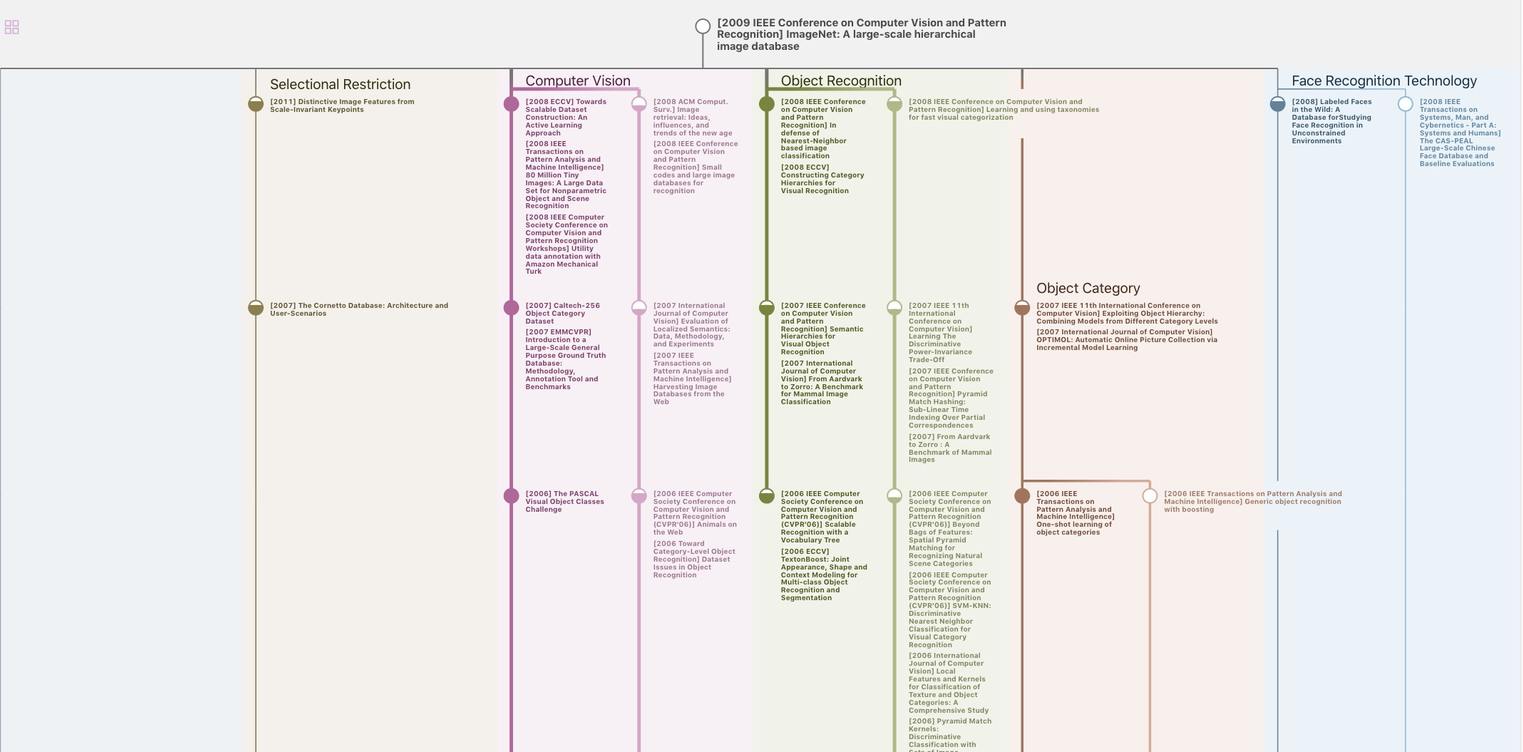
生成溯源树,研究论文发展脉络
Chat Paper
正在生成论文摘要