Efficient Self-Learning Evolutionary Neural Architecture Search
SSRN Electronic Journal(2023)
Abstract
The evolutionary algorithm has become a major method for neural architecture search recently. However, the fixed probability distribution employed by the traditional evolutionary algorithm may lead to structural complexity and redundancy due to its inability to control the size of individual architectures, and it cannot learn from empirical information gathered during the search process to guide the subsequent search more effectively and efficiently. Moreover, evaluating the performance of all the searched architectures requires significant computing resources and time overhead. To overcome these challenges, we present the Efficient Self-learning Evolutionary Neural Architecture Search (ESE-NAS) method. Firstly, we propose an Adaptive Learning Strategy for Mutation Sampling, composed of a Model Size Control module and a Credit Assignment method for Mutation Candidates, to guide the search process by learning from the model size information and evaluation results of the architectures and adjusting the probability distributions for evolution sampling accordingly. Additionally, we developed a neural architecture performance predictor to further improve the efficiency of NAS. Experiments on CIFAR-10 and CIFAR-100 datasets show that ESE-NAS significantly brings forward the first hitting time of the optimal architectures and reaches a competitive performance level with classic manual-designed and NAS models while maintaining structural simplicity and efficiency.
MoreTranslated text
Key words
architecture,search,self-learning
AI Read Science
Must-Reading Tree
Example
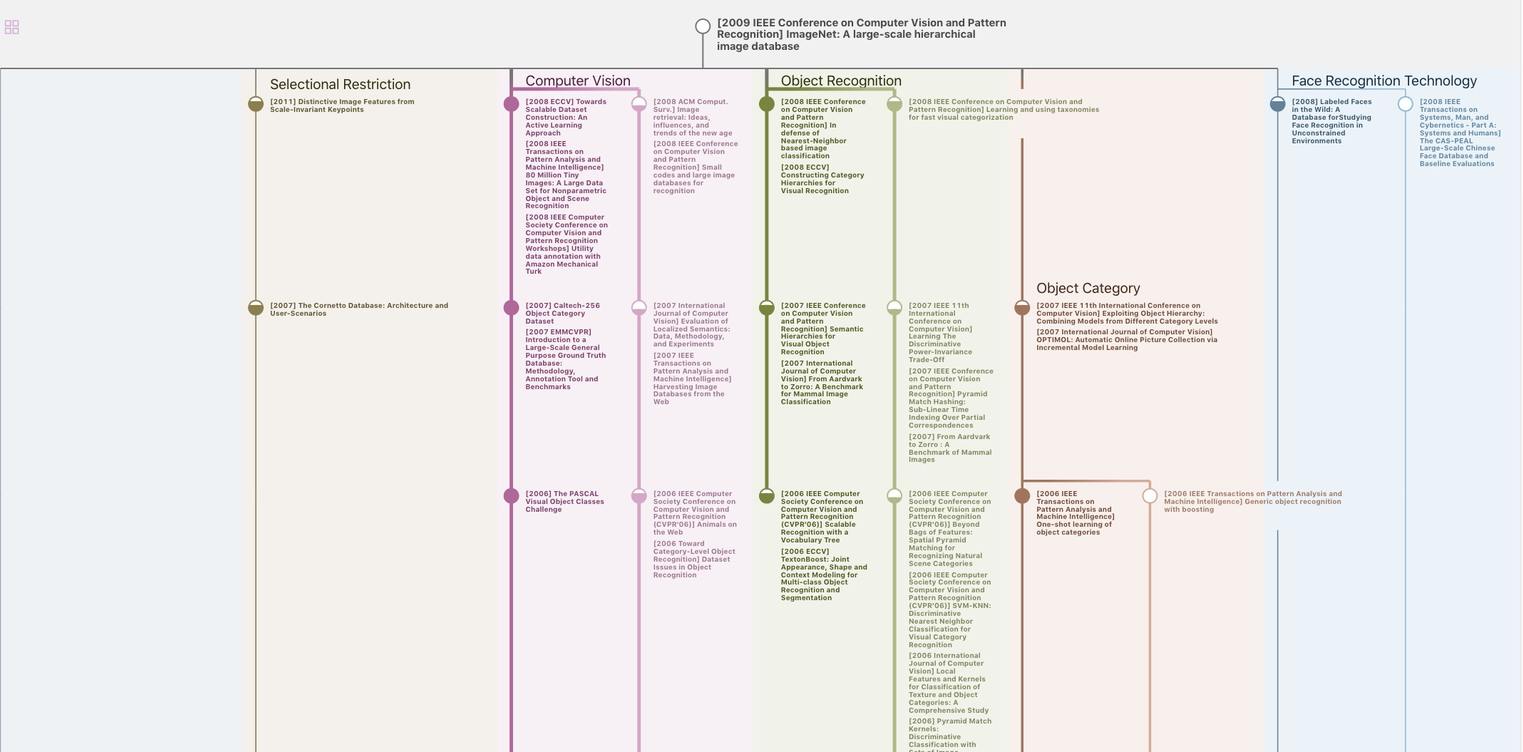
Generate MRT to find the research sequence of this paper
Chat Paper
Summary is being generated by the instructions you defined