Physics-aware learning of nonlinear limit cycles and adjoint limit cycles
INTER-NOISE and NOISE-CON Congress and Conference Proceedings(2023)
摘要
Thermoacoustic oscillations occur when the heat released by a flame is sufficiently in phase with the acoustic pressure. Under this condition, the linear instability can saturate to a nonlinear self-excited oscillation with a large amplitude. A typical nonlinear regime is a limit cycle, which is characterised by a periodic orbit in the thermoacoustic dynamics. In this paper, we develop a physics-aware data-driven method to predict periodic solutions using forward neural networks. The physics is constrained in two ways. First, the training is informed by a physical residual, which penalises solutions that violate the conservation of mass, momentum, and energy. Second, periodicity is imposed by introducing periodic activation functions in the neural network. We test the algorithm on a nonlinear time-delayed model of a Rijke tube. Adjoint methods offer a cheap and easy way to calculate the gradients with respect to design parameters, hence we extend our study to learning the adjoint variables of the Rijke system, which also settle onto periodic oscillations. We find that (i) periodic solutions of thermoacoustic systems can be accurately learned with this method, (ii) for periodic data, periodic activations outperform conventional activations in terms of prediction capability beyond the training range, and (iii) under the physical constraints, fewer data is sufficient to achieve a good performance. This work opens up possibilities for the prediction of nonlinear thermoacoustics by combining physical knowledge and data.
更多查看译文
关键词
nonlinear limit cycles,learning,physics-aware
AI 理解论文
溯源树
样例
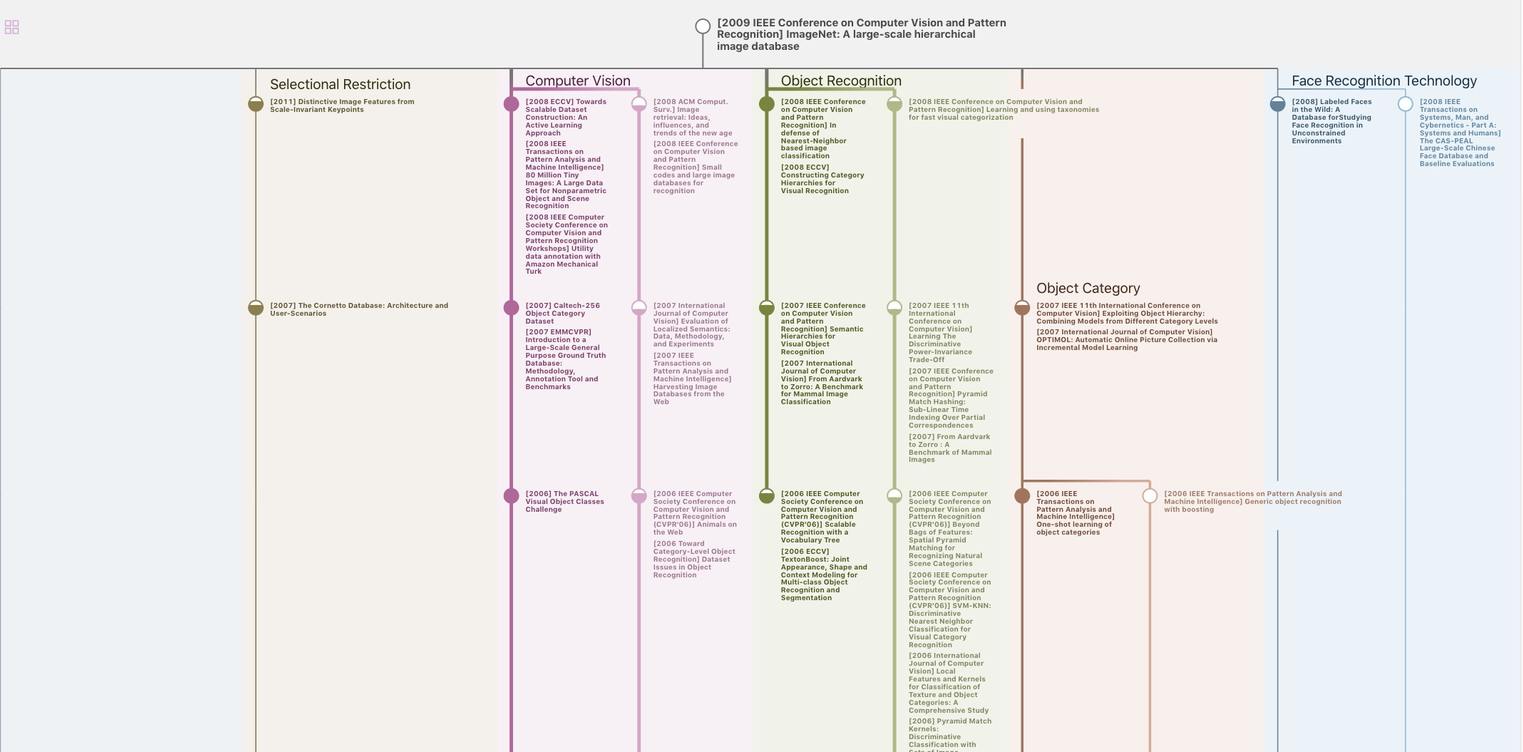
生成溯源树,研究论文发展脉络
Chat Paper
正在生成论文摘要