Generative Adversarial Imitation Learning from Human Behavior with Reward Shaping
2022 34th Chinese Control and Decision Conference (CCDC)(2022)
Abstract
Generative adversarial imitation learning (GAIL) aims to make a robot learn optimal policy from expert demonstrations. However, its application mainly focuses on the robot’s partial body control, such as the mechanical arm, and is rarely applied in whole-body action learning. Although making robots mimic human behavior has always been a mesmerizing goal in robotics, it is challenging for GAIL to reproduce complex human-like behaviors because of unsatisfactory performance. Furthermore, for multi-dimensional complex humanoid action imitation, only using output from discriminator may limit the performance of GAIL. To solve these problems, we propose a method, Generative Adversarial Imitation Learning from Human Behavior with Reward Shaping (GAIL-RS), enabling the robot to learn humanoid behaviors effectively. Instead of directly learning policy, a reward shaping mechanism is incorporated to improve the discriminator’s performance. In addition, we utilize the proximal policy optimization (PPO) algorithm as our generator to enhance imitation performance. Experiments were conducted on two robotic humanoid imitation tasks in simulation. The results demonstrate that our method can learn the optimal policy from reference motion and performs better than other methods.
MoreTranslated text
Key words
Robot Learning,Imitation Learning,Humanoid behavior,Generative Adversarial Imitation Learning,Reward Shaping
AI Read Science
Must-Reading Tree
Example
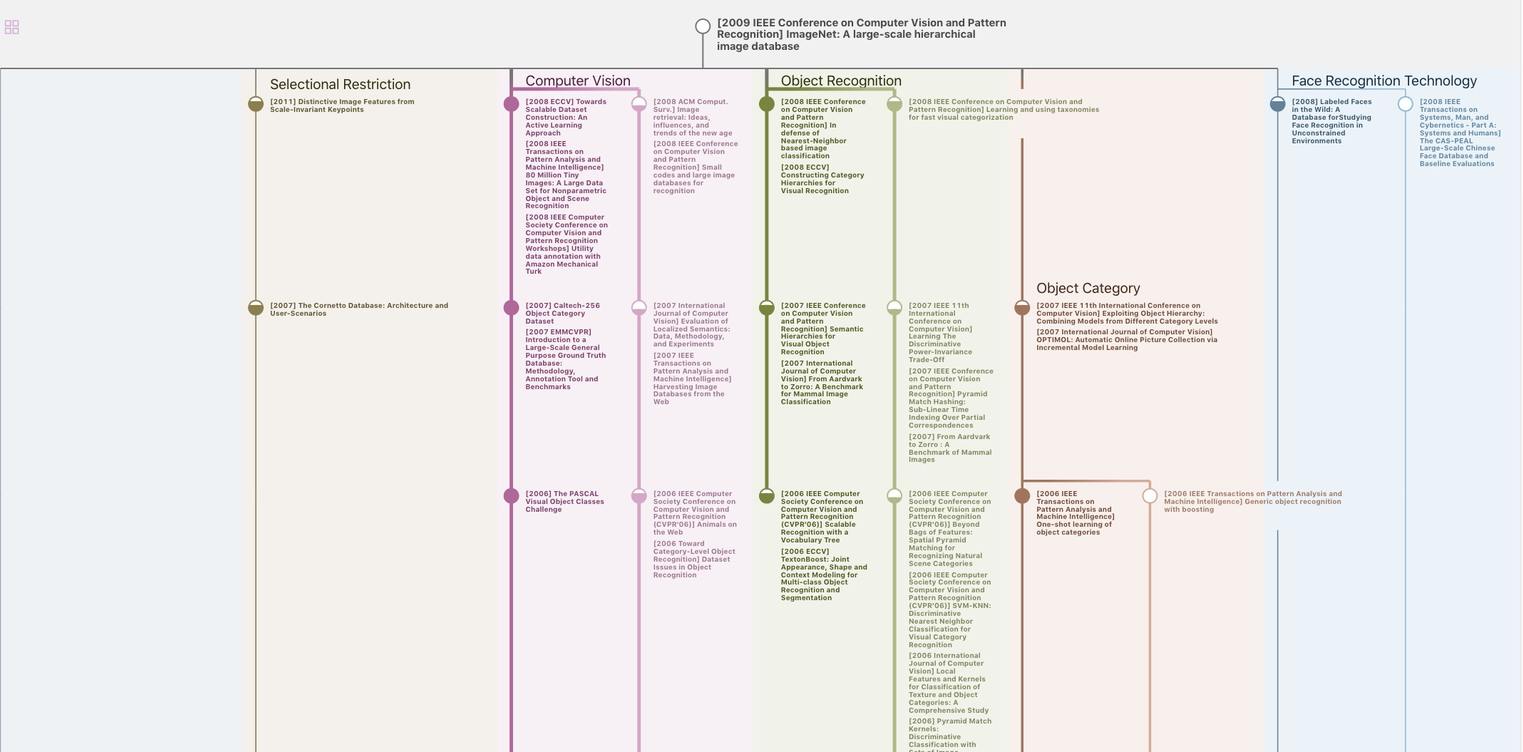
Generate MRT to find the research sequence of this paper
Chat Paper
Summary is being generated by the instructions you defined