An Efficient Deep Learning Based Workflow Incorporating a Reduced Physics Model for Drainage Volume Visualization in Unconventional Reservoirs
Machine Learning Applications in Subsurface Energy Resource Management(2022)
摘要
We present a novel deep learning based workflow incorporating a reduced physics model that can efficiently visualize well drainage volume and pressure front propagation in unconventional reservoirs in near real time. There are three key features in our approach: first, the fast-marching method (FMM)-based rapid simulation is used in place of full-physics simulation to efficiently generate training datasets while capturing key underlying physics. Second, an autoencoder network is applied to reduce the high-dimensional training data to facilitate machine learning application. Finally, instead of pressure and saturation maps for multiple time steps, the well drainage volume given by the diffusive time-of-flight (DTOF) map is used as a representative image of the subsurface flow to further simplify the deep learning network architecture. These features make the workflow very efficient, and well suited for field-scale applications. The novelty of our approach lies in the framework that combines the strengths of deep learning based models and the FMM-based rapid simulation. The proposed workflow has the flexibility to incorporate various spatial and temporal data types as demonstrated using a field application.
更多查看译文
关键词
drainage volume visualization,unconventional reservoirs,deep learning
AI 理解论文
溯源树
样例
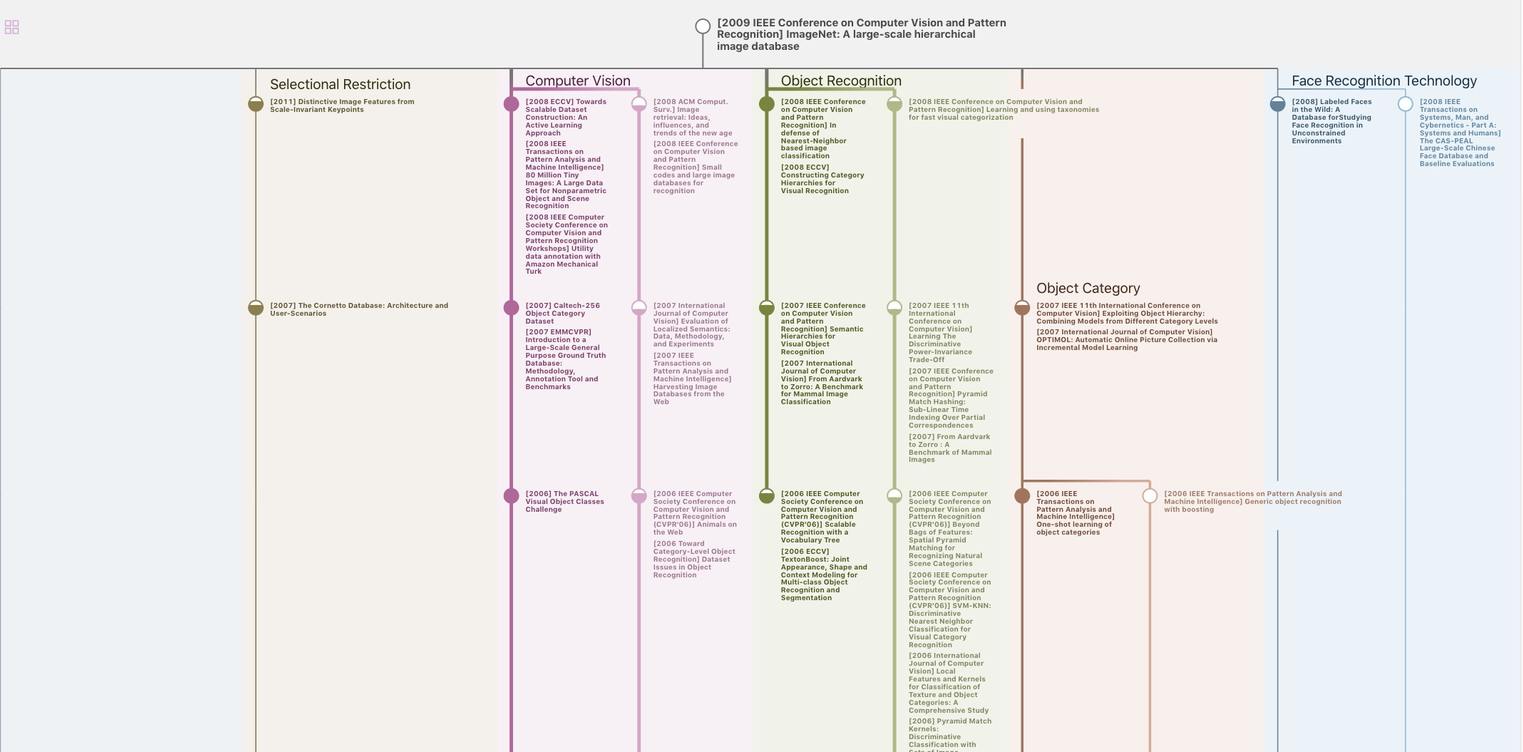
生成溯源树,研究论文发展脉络
Chat Paper
正在生成论文摘要