Classification of Vulnerable Road Users based on Range-Doppler Maps of 77 GHz MIMO Radar using Different Machine Learning Approaches.
2022 The 6th International Conference on Graphics and Signal Processing (ICGSP)(2022)
摘要
This paper involves the development of an intelligent delineator for road traffic detecting potential conflict situations between motor vehicles and vulnerable road users at an early stage. By emitting warning signals, collisions between the road users concerned can then be prevented. The prototype used here includes, among other sensors, a high-resolution FMCW radar capable of detecting, and imaging objects. The goal of this work is to develop a Machine Learning (ML) model for object classification of vulnerable road users in radar frames. A 77 GHz chirp-sequence radar is used to record Range-Doppler maps from object classes of car, bicyclist, pedestrian and empty street at different locations. Objective of this is to cover different levels of background noise in the data caused by the different environments due to trees or bushes. For the data acquisition, simple traffic scenarios have been simulated at Heilbronn University. In selecting a suitable ML algorithm for the classifier, the main challenge was that modern machine learning methods are data-based models which require a lot of data and are generally lacking in explainability, such as neural networks. However, the great advantage is that the correlations in the data are learned automatically. With knowledge-based methods, on the other hand, the big advantage is that they are explainable and require much less data, but assume an extensive domain knowledge. Hybrid learning, also called Informed ML, represents a combination of the methods previously mentioned and their advantages. In this paper, one approach from each of these methods is selected as well as trained, and its results are compared to each other. The respective approaches investigated are a deep neural network (DNN), a Support Vector Machine (SVM), and a hybrid model of a SVM and a specific neural network for feature extraction called Autoencoder (AE). In this comparison the SVM performs with prediction accuracies around 80%. The hybrid model performs better achieving prediction accuracies around 90%. The best results of this comparator are achieved by the DNN, which has a prediction accuracy of around 98%.
更多查看译文
AI 理解论文
溯源树
样例
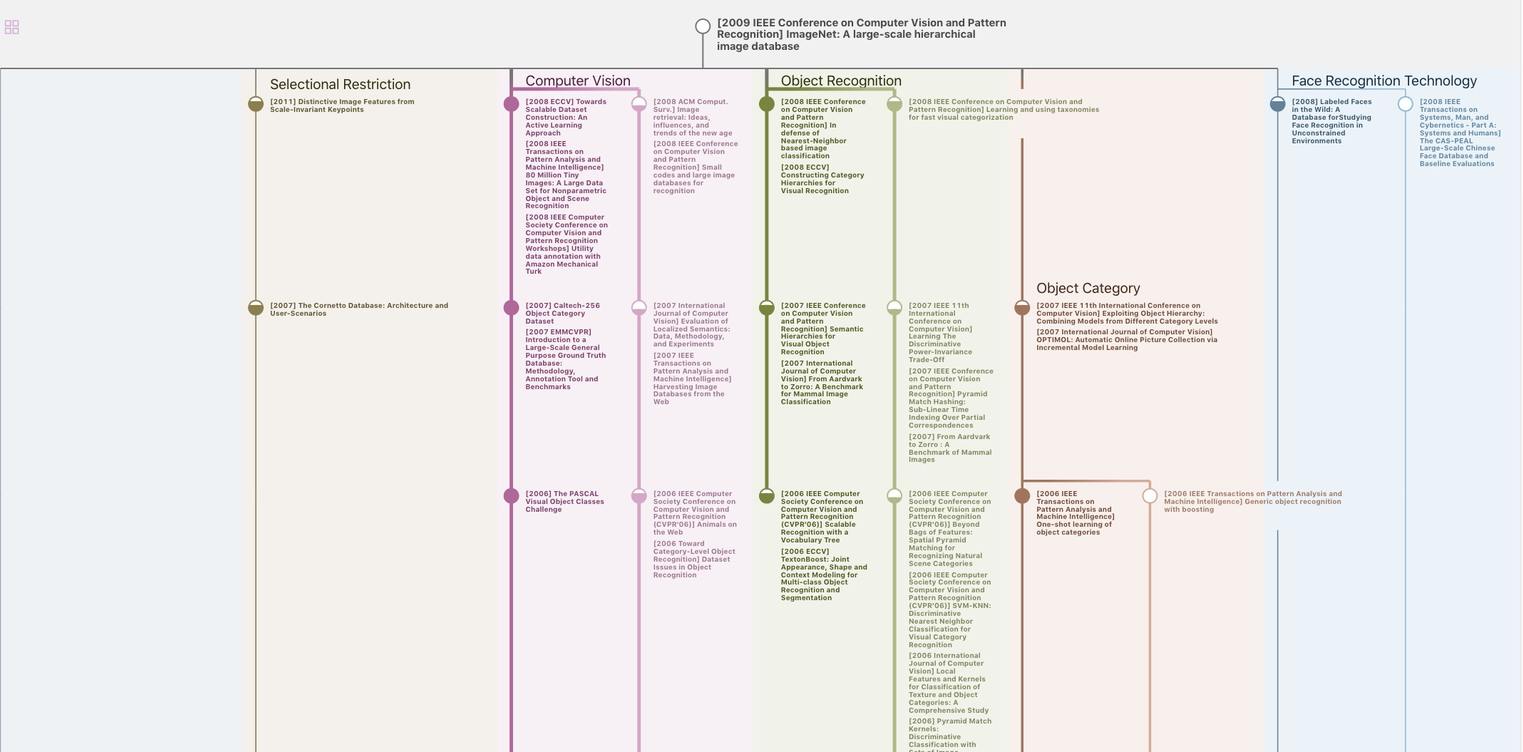
生成溯源树,研究论文发展脉络
Chat Paper
正在生成论文摘要