Machine Learning for Lung Cancer Prediction Using Pan-Tumor Associated Peripheral Blood Laboratory Markers
SSRN Electronic Journal(2022)
摘要
Background: Low-dose CT (LDCT) is recommended for screening people at high risk for lung cancer, but large numbers of low-risk people, such as the never-smoking population, are missed. Liquid biopsy for early cancer and cancer recurrence detection has been studied for a long time, but meaningful clinical laboratory data based on peripheral blood were underappreciated and underutilized.Methods: Using machine learning methods, a lung cancer prediction model was trained on 24 indicators of peripheral blood laboratory markers and patient ages were recorded at the time of lung cancer diagnosis. We assembled 7060 lung cancer cases and 3368 contemporaneous benign cases to train and test the model, using the area under the receiver operating characteristic curve (AUC), sensitivity, and specificity as measures of model performance. The benign disease datasets were divided into three subpopulations according to disease type, including one infectious subpopulation and two non-infectious subpopulations, which were used to train or test the model. The models were prospectively validated on internal datasets based on 77 lung cancer cases involving hospitalization for benign diseases.Findings: In the lung cancer prediction model with the infectious subpopulation (group 1) as the test dataset, the AUC value of stage I-II lung cancer patients was 0.70. The benign vertebral disease dataset was used as the test dataset, and the AUC value of stage I-II lung cancer patients was 0.74. Taking cerebrovascular disease as the test dataset, the AUC value of stage I-II lung cancer patients was 0.75. Using cerebrovascular disease as the test dataset, the diagnostic sensitivity was 67.2% at the predefined specificity of 95%. The model was validated on an internally traceable lung cancer dataset composed of 77 lung cancer patients with hospitalization records in our hospital for non-neoplastic diseases before the diagnosis of lung cancer. Analysis of previous hospitalization data showed that at 95% specificity, our model would predict lung cancer in eight patients at the time of their previous hospitalization, among which five patients had negative chest radiographs. Considering the five patients with negative chest radiographs, our model would predict lung cancer 25.10 ± 16.48 months before their actual lung cancer diagnosis. Two of these patients with negative chest radiographs had stage I lung cancer at the time of their diagnosis. Our lung cancer prediction model is publicly available at https://www.mtaibt.com.Interpretation: The pTablab (pan-tumor associated peripheral blood laboratory markers) lung cancer prediction model has better performance in predicting lung cancer for people who do not require treatment for infectious diseases and can predict lung cancer before lung nodules appear on imaging.Funding: Integrated innovation and application of key technologies for precise prevention and treatment of primary lung cancer, Chongqing, China (No. 2019ZX002).Declaration of Interest: The authors declare that they have no conflicts of interestEthical Approval: This study was approved by the Institutional Review Board of Chongqing University Cancer Hospital.
更多查看译文
AI 理解论文
溯源树
样例
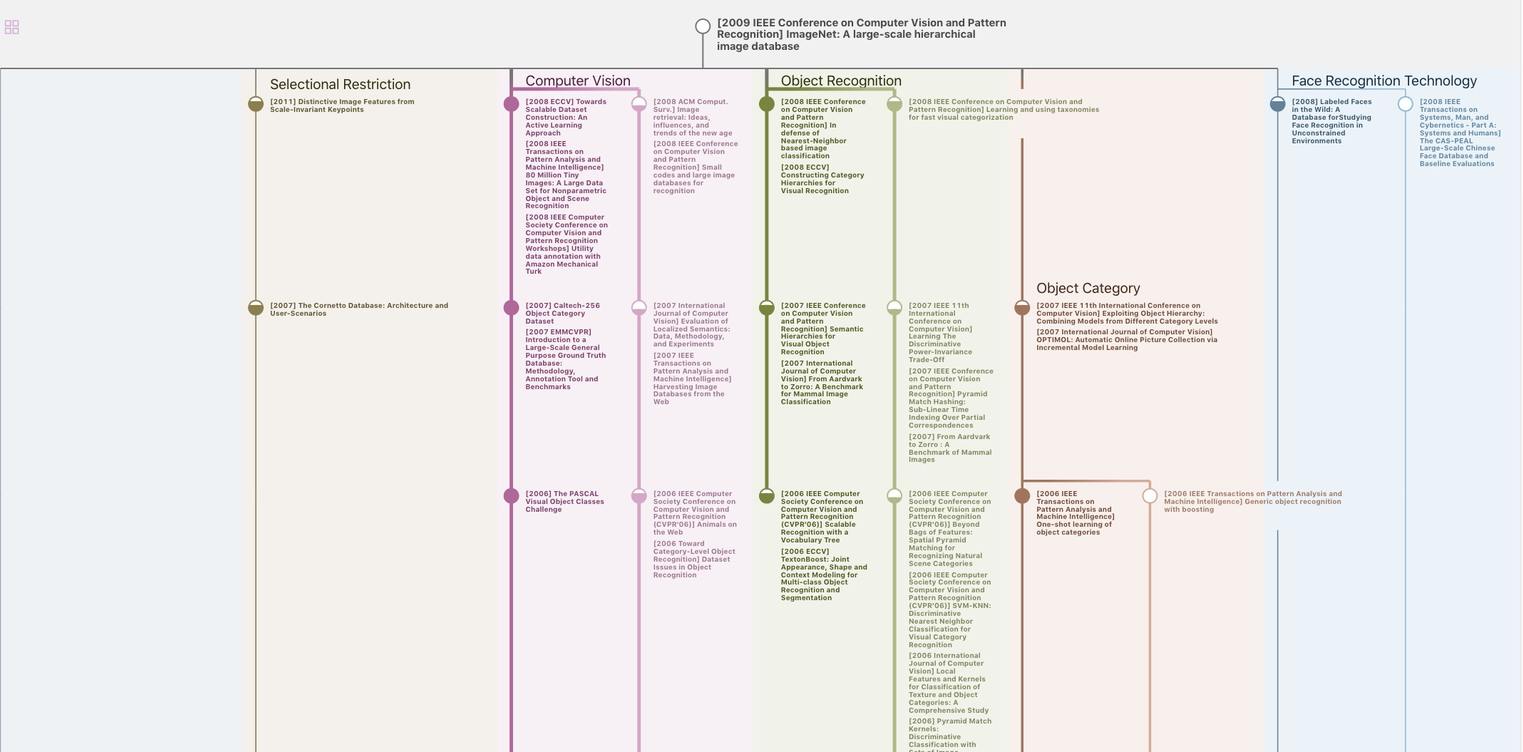
生成溯源树,研究论文发展脉络
Chat Paper
正在生成论文摘要