A Weakly Supervised Time Series Analysis Framework for Anomaly Detection in Nuclear Power Plants
Volume 15: Student Paper Competition(2022)
摘要
Abstract Condition monitoring is essential to the management and maintenance of Nuclear Power Plants (NPPs), as anomalies in the condition of components can affect the normal operation state of the entire plant. Therefore, timely and automatic detection of anomalies plays an important role and is in high demand. At present, deep learning is widely used for anomaly detection. Nevertheless, anomalies are difficult to define, sparsely occurring, and are accompanied by variable noise labels, which poses challenges to detection. Moreover, the problems such as loss of temporal features and gradient vanishing that exist in general deep learning models when dealing with time series data also increase the difficulty of anomaly detection. In response to these problems, a weakly supervised time series analysis framework for anomaly detection in NPPs is proposed, constituted of weakly supervised learning (WSL) and attention mechanism. The validation of the proposed framework was performed on the High Temperature Engineering Test Reactor (HTTR) anomaly cases dataset using the analytical code “ACCORD”, which distributed anomalies independently across multiple instruments and were recorded from the responding sensors to each anomaly. At this stage, 3 classes of anomalies were used as input data for the validation experiments. The experimental results demonstrate the effectiveness and feasibility of the proposed framework on anomaly tasks for the condition monitoring of NPPs.
更多查看译文
关键词
anomaly detection,time series analysis,time series,power plants
AI 理解论文
溯源树
样例
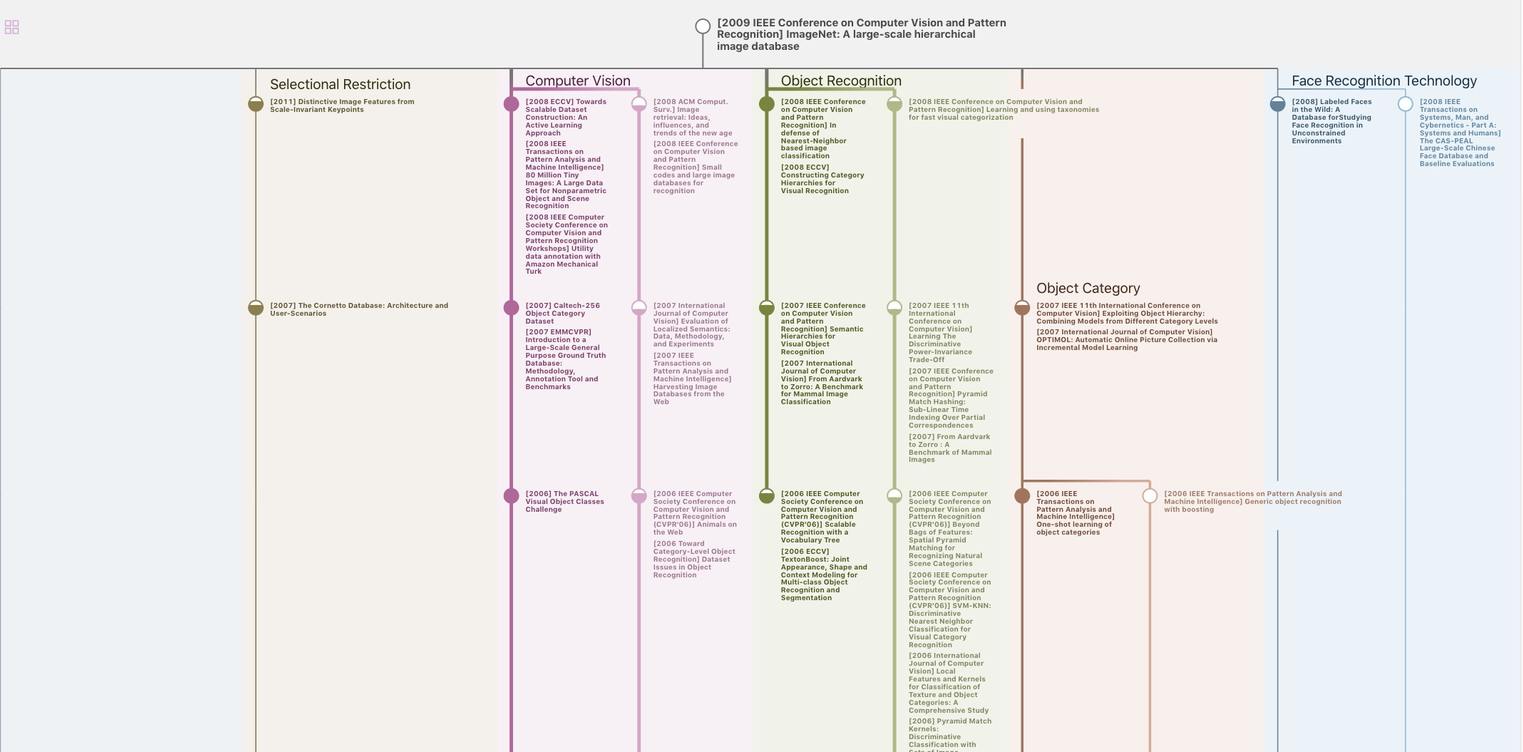
生成溯源树,研究论文发展脉络
Chat Paper
正在生成论文摘要