Adaptive convolution kernel network for change detection in hyperspectral images
Applied Optics(2023)
摘要
Feature extraction is a key step in hyperspectral image change detection. However, many targets with great various sizes, such as narrow paths, wide rivers, and large tracts of cultivated land, can appear in a satellite remote sensing image at the same time, which will increase the difficulty of feature extraction. In addition, the phenomenon that the number of changed pixels is much less than unchanged pixels will lead to class imbalance and affect the accuracy of change detection. To address the above issues, based on the U-Net model, we propose an adaptive convolution kernel structure to replace the original convolution operations and design a weight loss function in the training stage. The adaptive convolution kernel contains two various kernel sizes and can automatically generate their corresponding weight feature map during training. Each output pixel obtains the corresponding convolution kernel combination according to the weight. This structure of automatically selecting the size of the convolution kernel can effectively adapt to different sizes of targets and extract multi-scale spatial features. The modified cross-entropy loss function solves the problem of class imbalance by increasing the weight of changed pixels. Study results on four datasets indicate that the proposed method performs better than most existing methods.
更多查看译文
关键词
adaptive convolution kernel network,hyperspectral images,change detection
AI 理解论文
溯源树
样例
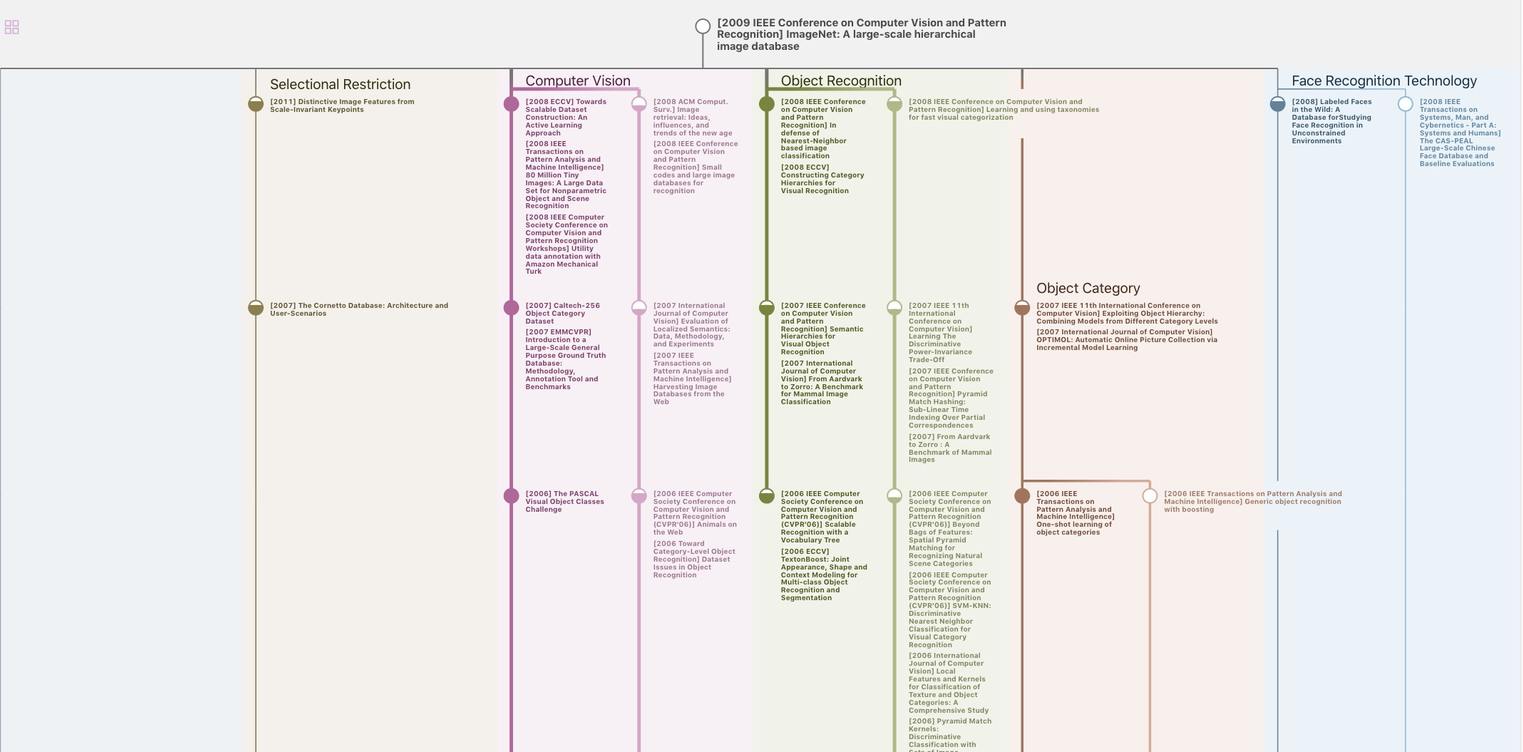
生成溯源树,研究论文发展脉络
Chat Paper
正在生成论文摘要