Method of Transformation of Image Classification Labels into Segmentation Masks
Microsystems, Electronics and Acoustics(2022)
摘要
Semantic image segmentation plays a crucial role in a wide range of industrial applications and has been receiving significant attention. Unfortunately, image segmentation tasks are notoriously difficult and different industries often require human experts. Convolutional neural networks (CNNs) have been successfully applied in many fields of image segmentation. But all of them still require a huge amount of hand-labeled data for training. A lot of research was conducted in the field of unsupervised and semi-supervised learning, which studies how to shrink the amount of training data at the same time preserving the quality of the model. But still another field of research - transformation of “cheap” (in terms of time, money and human resources) markup into “expensive” is novel. In this work a new approach of generating semantic segmentation masks, using only classification labels of the image, was proposed. Proposed method is based on the GradCam algorithm, which can produce image activation heatmap, using only class label. But GradCams’ heatmaps are raw for final use, so additional techniques and transforms should be applied in order to get final usable masks. Experiments were conducted on the task of detecting defects on steel plates — Kaggle- Severstal: Steel Defect Detection. After that Dice metric was computed using a classical training approach and proposed method: classical approach - 0.621, proposed method - 0.465. Proposed approach requires much less human resources compared to the classical approach. Moreover, after visual inspection of results it is obvious that the proposed approach has successfully completed the task of defect localization.
更多查看译文
关键词
image classification labels,segmentation,transformation
AI 理解论文
溯源树
样例
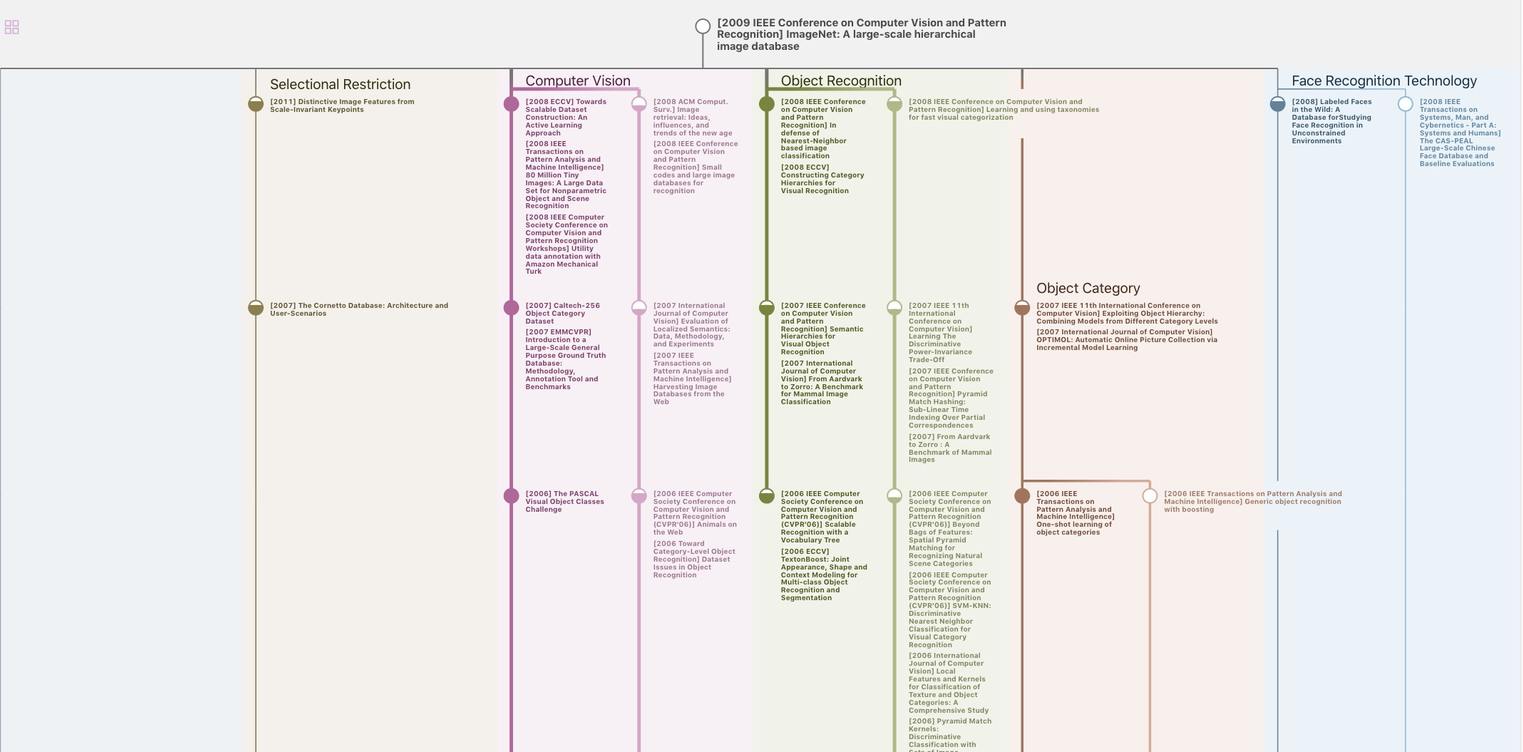
生成溯源树,研究论文发展脉络
Chat Paper
正在生成论文摘要