Robotic Continuous Grasping System by Shape Transformer-Guided Multiobject Category-Level 6-D Pose Estimation
IEEE TRANSACTIONS ON INDUSTRIAL INFORMATICS(2023)
Abstract
Robotic grasping is one of the key functions for realizing industrial automation and human-machine interaction. However, current robotic grasping methods for unknown objects mainly focus on generating the 6-D grasp poses, which cannot obtain rich object pose information and are not robust in challenging scenes. Based on this, in this article, we propose a robotic continuous grasping system that achieves end-to-end robotic grasping of intraclass unknown objects in 3-D space by accurate category-level 6-D object pose estimation. Specifically, to achieve object pose estimation, first, we propose a global shape extraction network (GSENet) based on ResNet1D to extract the global shape of an object category from the 3-D models of intraclass known objects. Then, with the global shape as the prior feature, we propose a transformer-guided network to reconstruct the shape of intraclass unknown object. The proposed network can effectively introduce internal and mutual communication between the prior feature, current feature, and their difference feature. The internal communication is performed by self-attention. The mutual communication is performed by cross attention to strengthen their correlation. To achieve robotic grasping for multiple objects, we propose a low-computation and effective grasping strategy based on the predefined vector orientation, and develop a graphical user interface for monitoring and control. Experiments on two benchmark datasets demonstrate that our system achieves state-of-the-art 6-D pose estimation accuracy. Moreover, the real-world experiments show that our system also achieves superior robotic grasping performance, with a grasping success rate of 81.6% for multiple objects.
MoreTranslated text
Key words
Grasping,Shape,Robots,Three-dimensional displays,Robot kinematics,Pose estimation,Feature extraction,Category-level 6-D pose estimation,global shape,robotic continuous grasping,shape transformer
AI Read Science
Must-Reading Tree
Example
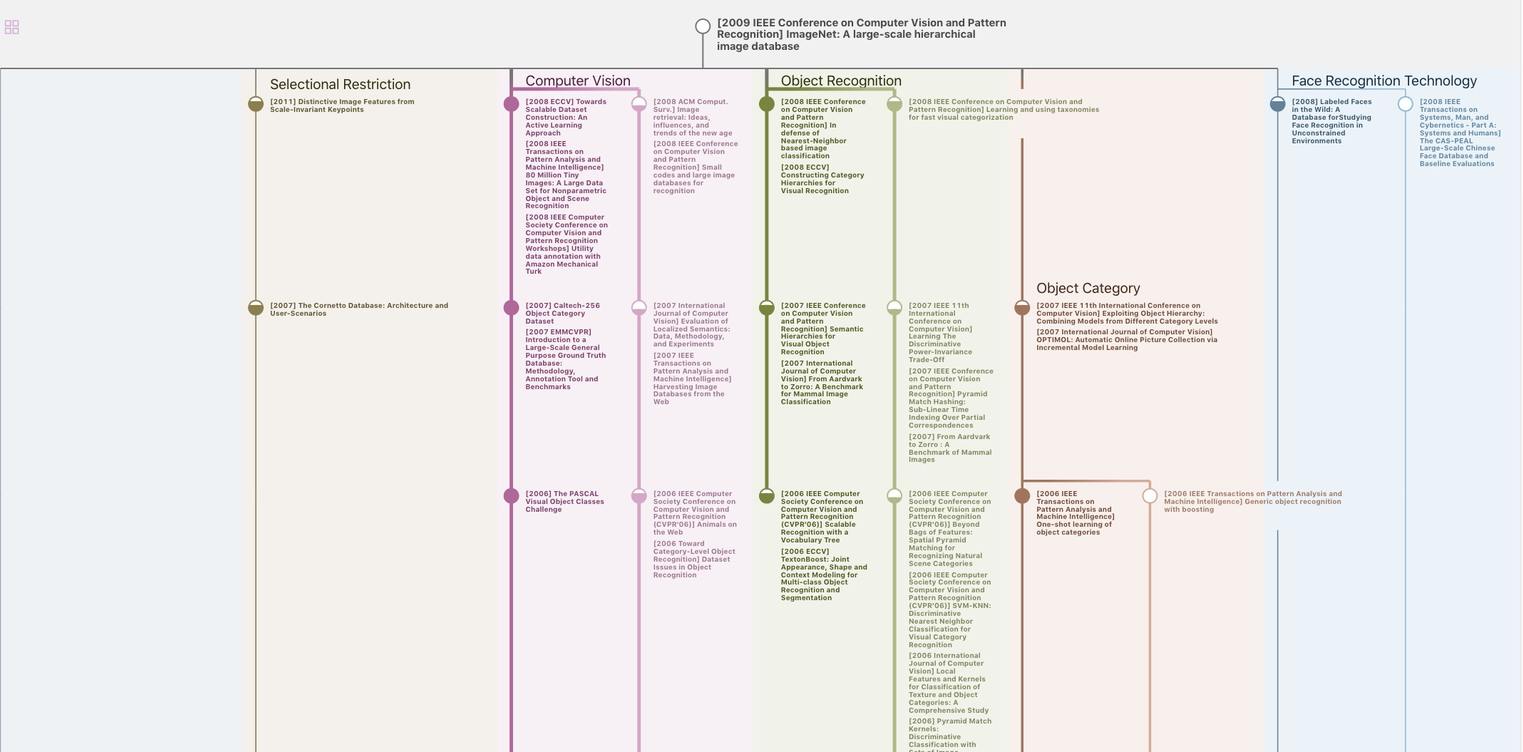
Generate MRT to find the research sequence of this paper
Chat Paper
Summary is being generated by the instructions you defined