Accelerating Machine Learning inference using FPGAs: the PYNQ framework tested on an AWS EC2 F1 Instance
Proceedings of 41st International Conference on High Energy physics — PoS(ICHEP2022)(2022)
摘要
In the past few years, using Machine and Deep Learning techniques has become more and more viable, thanks to the availability of tools which allow people without specific knowledge in the realm of data science and complex networks to build AIs for a variety of research fields. This process has encouraged the adoption of such techniques, e.g. in the context of High Energy Physics. In order to facilitate the translation of Machine Learning (ML) models to fit in the usual workflow for programming FPGAs, a variety of tools have been developed. One example is the HLS4ML toolkit, which allows the translation of Neural Networks (NN) built using tools like TensorFlow to a High-Level Synthesis description (e.g. C++) in order to implement this kind of ML algorithms on FPGAs. This paper presents the activity running at the University of Bologna and INFN-Bologna devoted to preliminary studies for the trigger systems of the Compact Muon Solenoid experiment at the CERN LHC accelerator. An open-source project from Xilinx called PYNQ is being tested combined with the HLS4ML toolkit. The PYNQ purpose is to grant designers the possibility to exploit the benefits of programmable logic and microprocessors using the Python language. The use of cloud computing in this work allows us to test the capabilities of this workflow, from the creation and training of a Neural Network and the creation of a HLS project using HLS4ML, to managing NN inference with custom Python drivers. The main application explored in this work lives in the context of the trigger system of the CMS, where new reconstruction algorithms are being developed due to the advent of the High-Luminosity phase of the LHC.
更多查看译文
关键词
fpgas,machine learning inference,pynq framework,machine learning
AI 理解论文
溯源树
样例
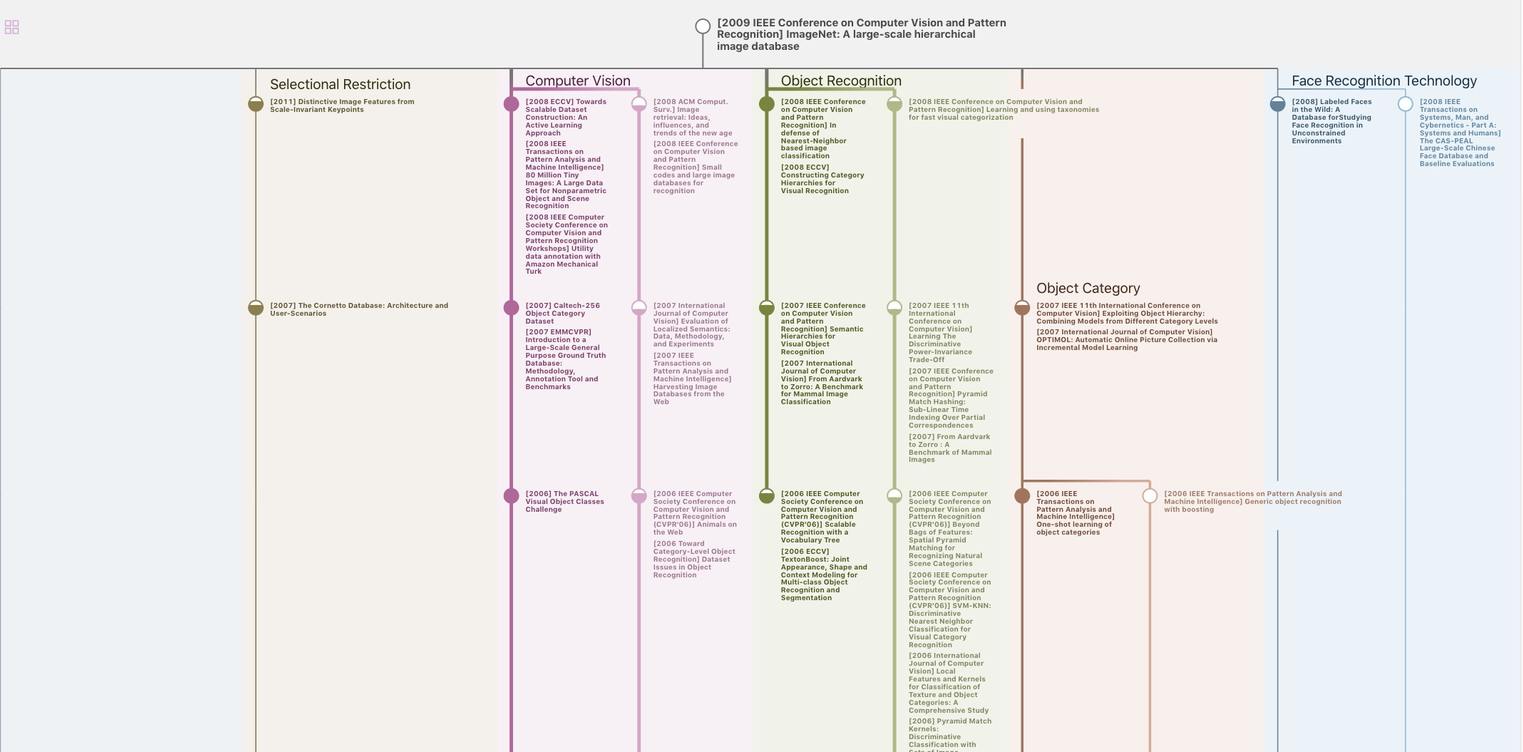
生成溯源树,研究论文发展脉络
Chat Paper
正在生成论文摘要